Semantic information extraction and search of mineral exploration data using text mining and deep learning methods
ORE GEOLOGY REVIEWS(2024)
摘要
Large-scale mineral resource reports offer a wealth of information for geological knowledge mining and mineral explorers' knowledge discovery. The geological conditions in which mineral deposits develop may be learned a great deal from these mineral exploration reports. Mineral exploration data may be queried and aggregated to effectively mitigate future exploration risks and reduce costs. However, due to the reports being presented in unstructured textual format, it becomes challenging to extract valuable geological data without manually scanning through a vast number of reports. This laborious process poses difficulties for geologists. To address this issue, this paper proposes a system that extracts a set of geologically relevant keywords/keyphrases of each chapter from each mineral exploration reposts using latent Dirichlet allocation (LDA), develops a topic graph, recognizes the geological entity and related relations for constructing a knowledge graph, and uses visualization of those graphs (e.g., topic graphs and knowledge graphs) to explore the contents of the report. The text mining and machine learning technique described here serves as the foundation for future research into incorporating semantic analysis into geological information extraction. The findings of this study show how automated text analysis may help with the quick processing of huge quantities of reports in order to identify target mineral systems and their related geological location and rock mineral composition. The suggested approaches can quickly and reliably convert mineral exploration data (e.g., text, figure, and table) into a structured form, which is a hitherto untouched field in geological knowledge mining.
更多查看译文
关键词
Mineral exploration data,Semantic search,Text mining,Topic extraction,Knowledge graph
AI 理解论文
溯源树
样例
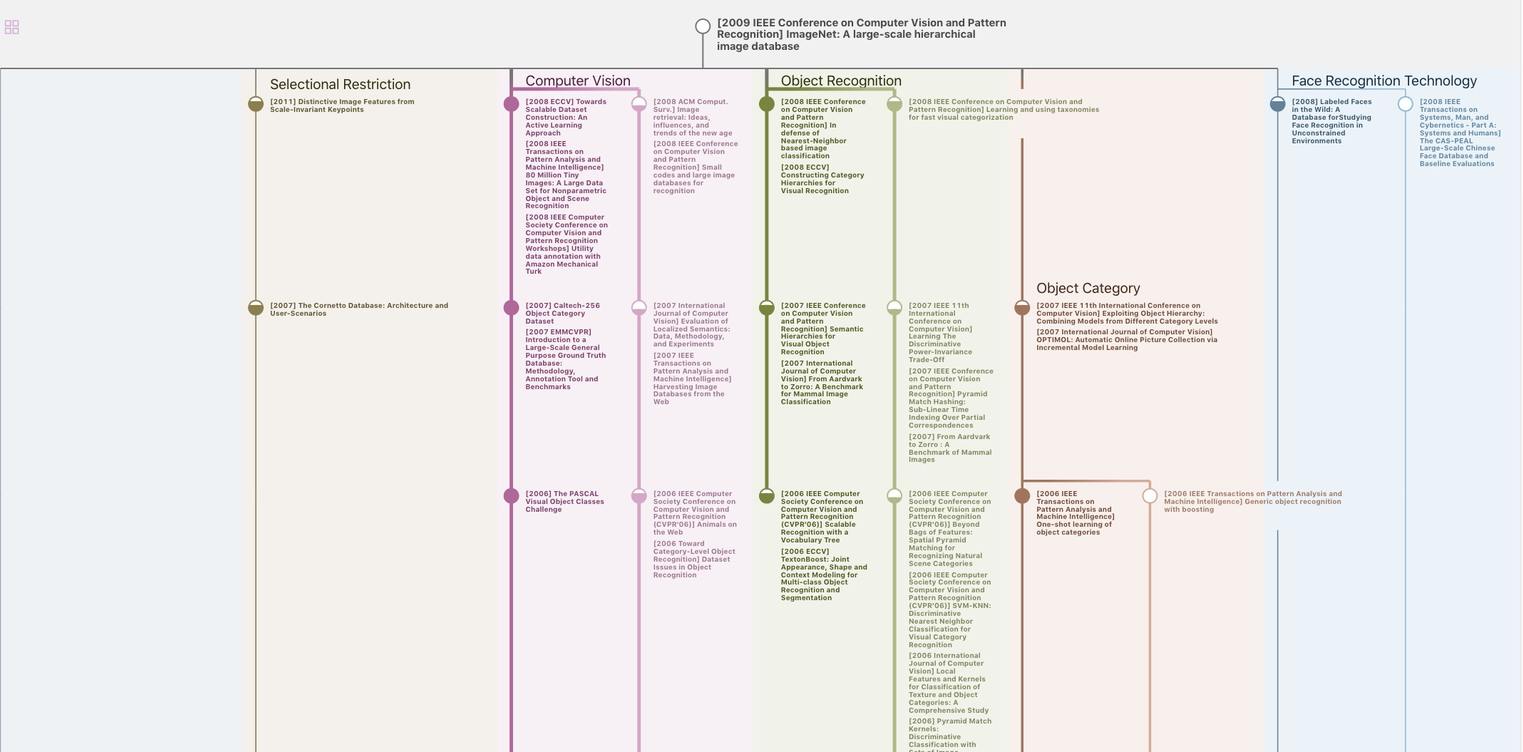
生成溯源树,研究论文发展脉络
Chat Paper
正在生成论文摘要