Do Topographic Deep ANN Models of the Primate Ventral Stream Predict the Perceptual Effects of Direct IT Cortical Interventions?
biorxiv(2024)
Abstract
Ever-advancing artificial neural network (ANN) models of the ventral visual stream capture core object recognition behavior and the neural mechanisms underlying it with increasing precision. These models take images as input, propagate through simulated neural representations that resemble biological neural representations at all stages of the primate ventral stream, and produce simulated behavioral choices that resemble primate behavioral choices. We here extend this modeling approach to make and test predictions of neural intervention experiments. Specifically, we enable a new prediction regime for topographic deep ANN (TDANN) models of primate visual processing through the development of perturbation modules that translate micro-stimulation, optogenetic suppression, and muscimol suppression into changes in model neural activity . This unlocks the ability to predict the behavioral effects from particular neural perturbations. We compare these predictions with the key results from the primate IT perturbation experimental literature via a suite of nine corresponding benchmarks. Without any fitting to the benchmarks, we find that TDANN models generated via co-training with both a spatial correlation loss and a standard categorization task qualitatively predict all nine behavioral results. In contrast, TDANN models generated via random topography or via topographic unit arrangement after classification training predict less than half of those results. However, the models’ quantitative predictions are consistently misaligned with experimental data, over-predicting the magnitude of some behavioral effects and under-predicting others. None of the TDANN models were built with separate model hemispheres and thus, unsurprisingly, all fail to predict hemispheric-dependent effects. Taken together, these findings indicate that current topographic deep ANN models paired with perturbation modules are reasonable guides to predict the qualitative results of direct causal experiments in IT, but that improved TDANN models will be needed for precise quantitative predictions.
### Competing Interest Statement
The authors have declared no competing interest.
MoreTranslated text
AI Read Science
Must-Reading Tree
Example
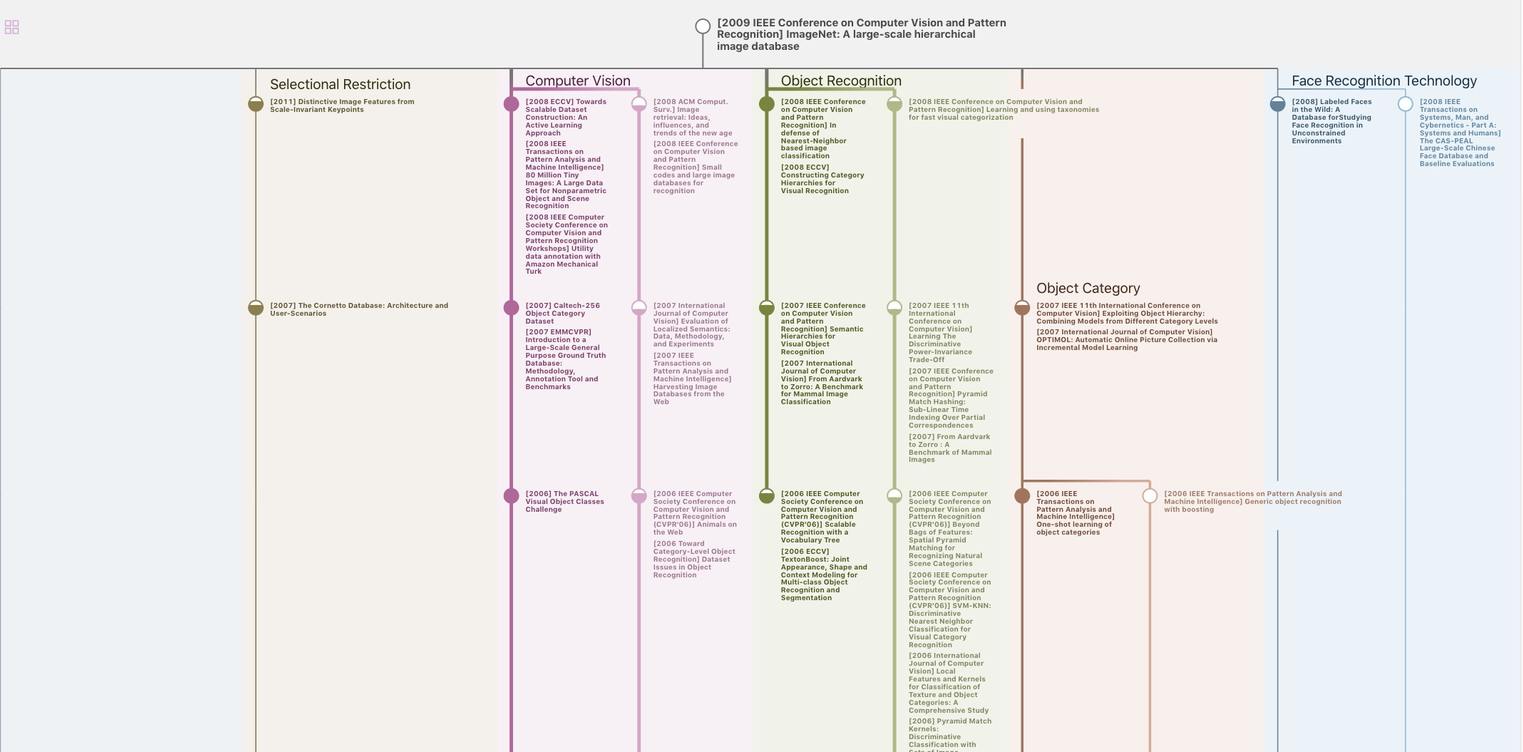
Generate MRT to find the research sequence of this paper
Chat Paper
Summary is being generated by the instructions you defined