Wind speed prediction utilizing dynamic spectral regression broad learning system coupled with multimodal information
ENGINEERING APPLICATIONS OF ARTIFICIAL INTELLIGENCE(2024)
Abstract
As the integration of wind energy into the power system increases, accurate wind speed prediction becomes crucial to ensure the reliable and economically efficient operation of the grid. The non-stationary, chaotic, and nonlinear characteristics of wind speed pose significant challenges for prediction models in uncovering the dynamic evolution process. To address these challenges, we proposed a wind speed prediction method based on the dynamic spectral regression broad learning system coupled with multimodal information (DSR-BLS). Firstly, we proposed a frequency density clustering-based mode decomposition (FDCMD) algorithm, which automatically transforms the non-stationary wind speed into multiple relatively stationary modal components. Next, we proposed the dynamic spectral regression (DSR) algorithm, which is based on dynamic-inner latent variable modeling and spectral regression. DSR can extract features and reconstruct the dynamic characteristics of wind speed through non-uniform embedding in the phase space. Finally, DSR-BLS is proposed to enhance the deterministic point prediction accuracy of the original BLS by utilizing multimodal features and dynamic features. The experiments show that DSR-BLS outperforms the comparative prediction methods in multi-step ahead prediction results.
MoreTranslated text
Key words
Dynamic feature extraction,Latent dynamic modeling,Nonuniform embedding,Broad learning system,Dynamic-inner canonical correlation analysis,Spectral regression
AI Read Science
Must-Reading Tree
Example
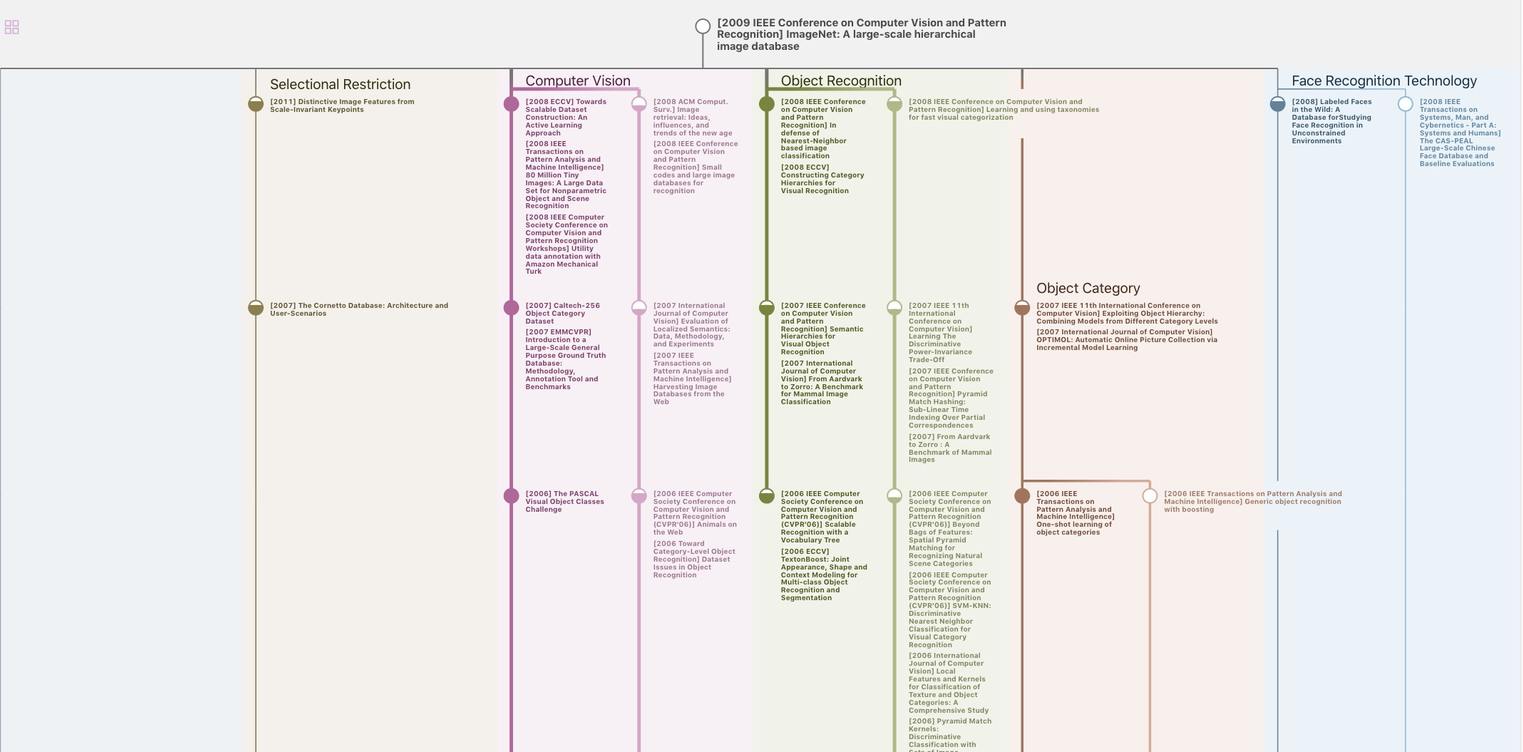
Generate MRT to find the research sequence of this paper
Chat Paper
Summary is being generated by the instructions you defined