Efficient Lightweight Intrusion Detection Method for IoT OS: A Study on the Application of Multi-Teacher Distillation.
2023 8th International Conference on Data Science in Cyberspace (DSC)(2023)
摘要
As the second important security measure behind the firewall, network intrusion detection provides effective assurance for computer network security. In recent years, deep learning (DL) has emerged as a promising approach for enhancing intrusion detection and achieving high experimental accuracy. However, due to performance limitations caused by resource consumption, deep neural network models with high requirements for floating-point calculations cannot be directly applied to Internet of Things devices. In our study, the decision tree is initially employed to reduce the feature dimension and extract spatial features. We then combine the teacher network based on the Bi-GRU algorithm to capture the temporal traffic features, achieving an accuracy rate of 99.41% on the CICIDS2017 dataset. We use these two high-accuracy models as teachers for multi-teacher knowledge distillation. The student model adopts a lightweight network architecture, with a scale only 2.5% of that of the teacher model. Finally, we compare the performance of the teacher network and the student network on Internet of Things devices. We find that the accuracy loss is only 0.09% compared to the teacher network. Moreover, the accuracy for DDoS attacks has been improved by 30.1% compared to the non-distilled model. The IDS framework we designed is well-suited for scenarios such as the Internet of Vehicles and can be integrated with the cloud to update the device-side model and enhance system security.
更多查看译文
关键词
Intrusion detection,Knowledge distillation,Bi-GRU,Lightweight
AI 理解论文
溯源树
样例
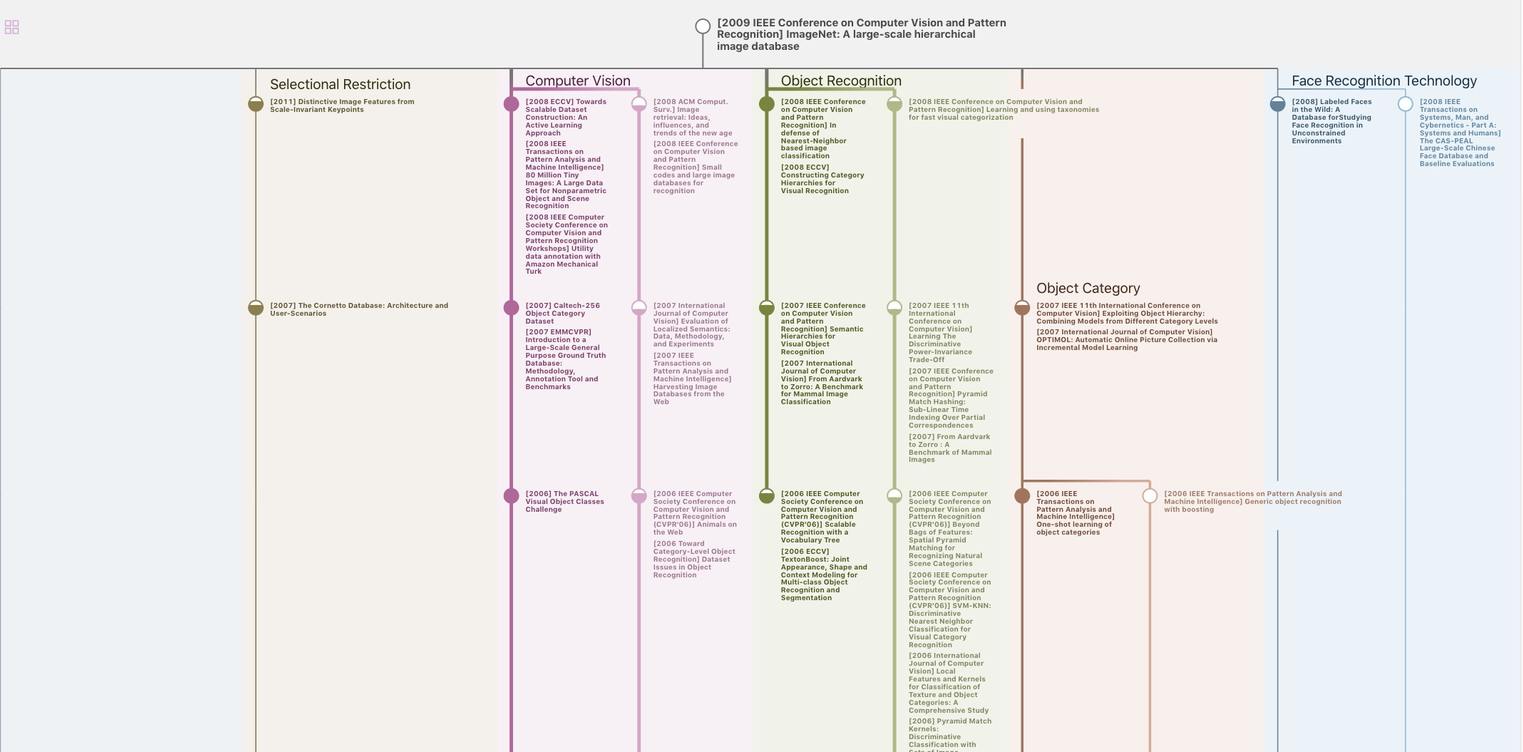
生成溯源树,研究论文发展脉络
Chat Paper
正在生成论文摘要