Universal Adversarial Perturbation From Dominant Feature For Speaker Recognition.
2023 8th International Conference on Data Science in Cyberspace (DSC)(2023)
摘要
Deep neural networks (DNNs) have greatly improved speaker recognition performance. However, DNNs are vulnerable to imperceptible adversarial example, which has attracted the attention in both academic and industrial areas in recent years. Although many elegant adversarial attack methods have been proposed, few of them studied Universal Adversarial Perturbation (UAP) against speaker recognition. In this paper, we propose a novel algorithm from the perspective of dominant features to generate UAP to mislead speaker recognition DNNs. Our method uses dominance as an optimization objective, and designs a tiny neural network to improve the efficiency. We conduct experiments on the TIMIT dataset and our method has achieved state-of-the-art results regarding attack success rate and imperceptibility. Moreover, leveraging the concept of dominant feature, our method significantly improves the training speed and can generate UAP more efficiently.
更多查看译文
关键词
speaker recognition,adversarial examples,universal adversarial perturbation,dominant feature
AI 理解论文
溯源树
样例
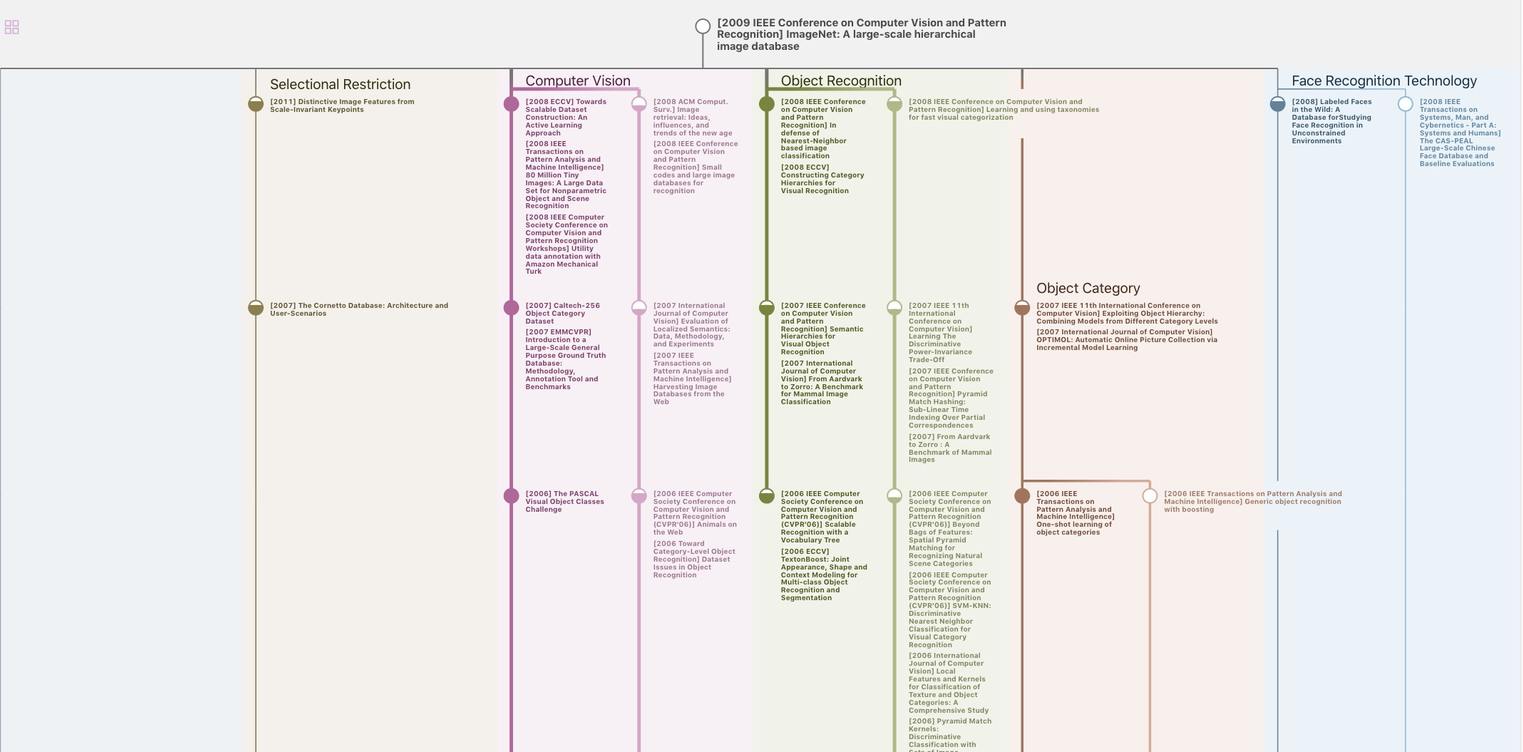
生成溯源树,研究论文发展脉络
Chat Paper
正在生成论文摘要