Machine Learning-Based NOMA for Multiuser MISO Broadcast Channels
IEEE Communications Letters(2024)
Abstract
This letter proposes machine learning-based non-orthogonal multiple access (NOMA) for a multiuser multiple-input single-output (MISO) broadcast channel (BC), where a transmitter selects and serves multiple users with a fixed rate using NOMA. Unlike a single-input single-output NOMA, where the optimal decoding order is determined by channel gains, the optimal decoding order for a MISO NOMA should be found with a brute force approach. In this case, the transmitter needs to check the validity of all decoding orders under a transmit power budget, so should calculate power allocations for all decoding order candidates, whose complexity is prohibitive as the number of users increases. Our proposed scheme uses a machine learning model to directly predict the optimal decoding order based on channel gains and cross-channel correlations. Once the decoding order is determined, we only need to calculate the power allocation for that decoding order, greatly reducing complexity. Our results show that our machine learning model performs well in finding the optimal decoding order and achieves performance comparable to the optimal scheme.
MoreTranslated text
Key words
NOMA,Transmitters,Decoding,Array signal processing,Interference,Signal to noise ratio,MISO communication,Non-orthogonal multiple access,machine learning,deep neural network,multiple-input single-output broadcast channel,decoding order
AI Read Science
Must-Reading Tree
Example
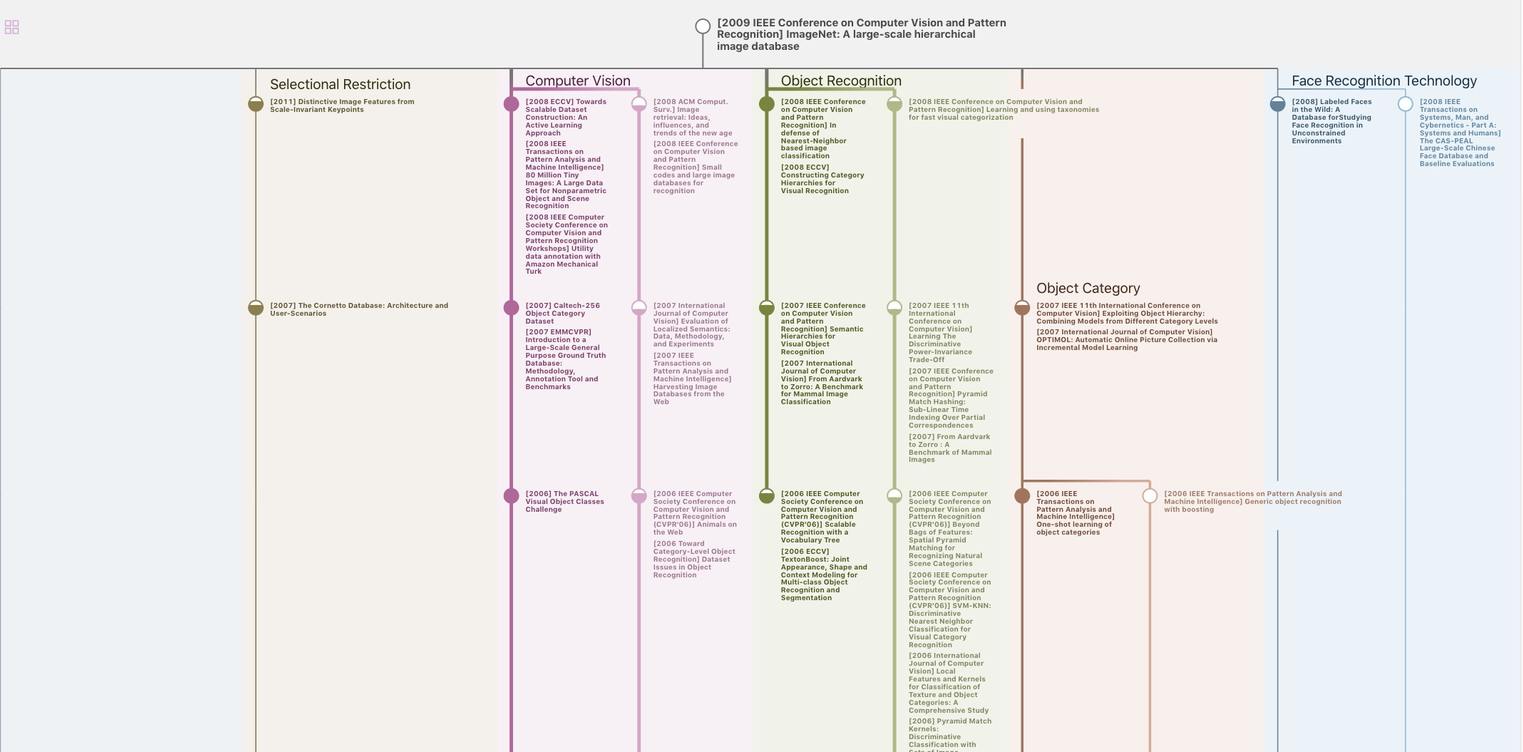
Generate MRT to find the research sequence of this paper
Chat Paper
Summary is being generated by the instructions you defined