An Optimal Transport-Based Federated Reinforcement Learning Approach for Resource Allocation in Cloud-Edge Collaborative IoT
IEEE INTERNET OF THINGS JOURNAL(2024)
摘要
In the traditional cloud-edge collaborative Internet of Things (IoT), the high-communication cost and slow convergence of the models often result in high-delay and energy consumption. In this article, a model and data dual-driven resource optimization mechanism is proposed for cloud-edge collaborative IoT applications. The model and data dual-driven mechanism is a joint delay and energy consumption optimization mechanism based on optimal transport and federated actor-critic (OTFAC) is proposed, which combines the offline and online learning. To be specific, in the model-driven offline learning phase, an optimization problem on the bandwidth and computation resource allocation is first formulated. The optimal transport (OT)-based offline optimization model is constructed. And then the OT-based algorithm is proposed to solve the optimization problem. In the data-driven online learning phase, federated actor-critic-based online optimization model are constructed. And then, the federated learning (FL) and actor-critic (AC) learning-based online resource optimization algorithm is designed to further reduce the delay and energy consumption with edge servers serving as local aggregators. Simulation results illustrate that the proposed OTFAC in this article reduces the average delay by 55% and the average energy consumption by 51% as compared with the benchmark hierarchical aggregation method HierFAVG. Compared with benchmark FL-based deep deterministic policy gradient method DDPG, the average delay is reduced by 47% and the average energy consumption is reduced by 43% by the proposed method.
更多查看译文
关键词
Actor-critic (AC) network,deep reinforcement learning (DRL),edge computing,joint delay and energy con-sumption optimization,optimal transport (OT)
AI 理解论文
溯源树
样例
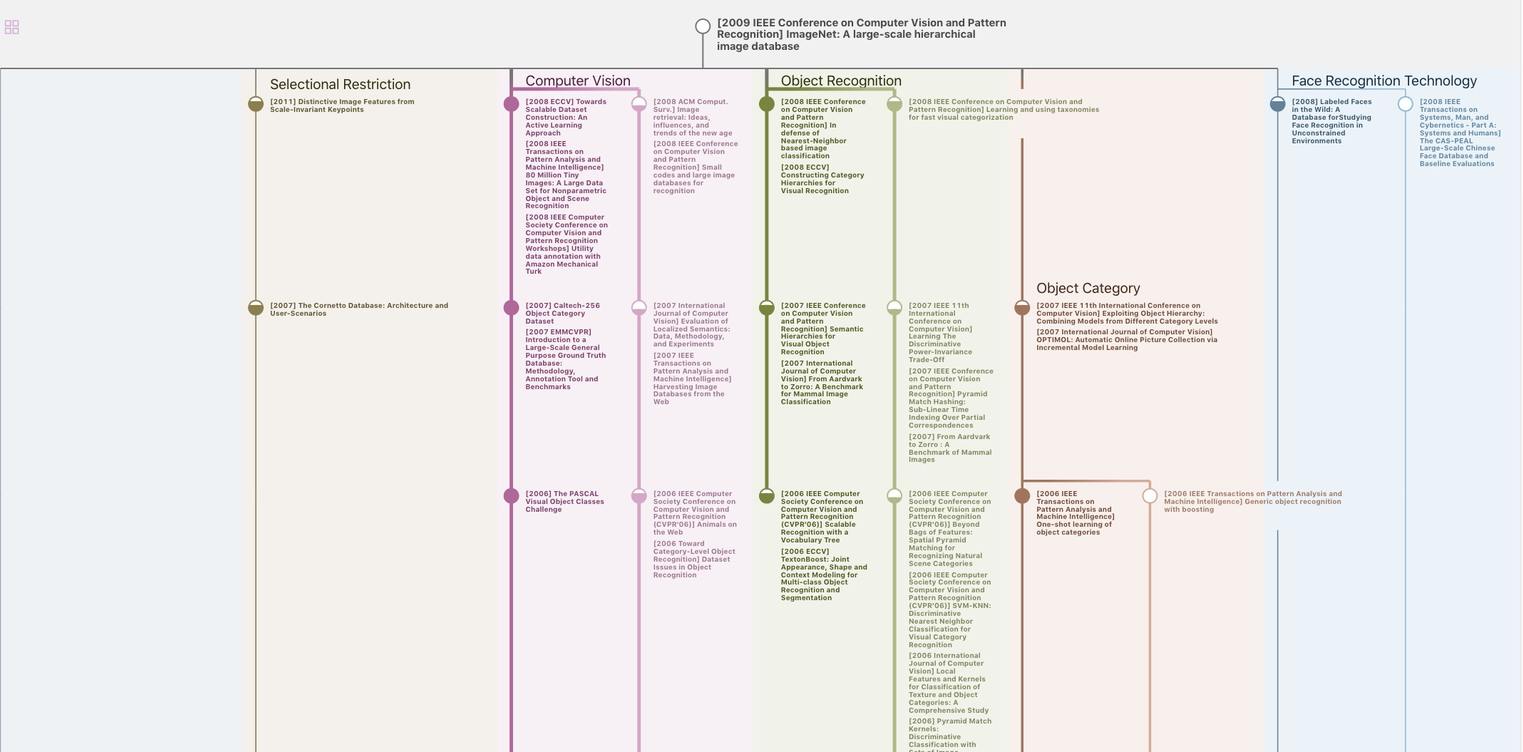
生成溯源树,研究论文发展脉络
Chat Paper
正在生成论文摘要