Blood Pressure Estimation Based on PPG and ECG Signals Using Knowledge Distillation
Cardiovascular Engineering and Technology(2024)
摘要
Objective Easy access bio-signals are useful for alleviating the shortcomings and difficulties associated with cuff-based and invasive blood pressure (BP) measurement techniques. This study proposes a deep learning model, trained using knowledge distillation, based on photoplethysmographic (PPG) and electrocardiogram (ECG) signals to estimate systolic and diastolic blood pressures. Methods The estimation model comprises convolutional layers followed by one bidirectional recurrent layer and attention layers. The training approach involves knowledge distillation, where a smaller model (student model) is trained by leveraging information from a larger model (teacher model). Results The proposed multistage model was evaluated on 1205 subjects from Medical Information Mart for Intensive Care (MIMIC) III database using the Association for the Advancement of Medical Instrumentation (AAMI) and the standards of the British Hypertension Society (BHS). The results revealed that our model performance achieved grade A in estimating both systolic blood pressure (SBP) and diastolic blood pressure (DBP) and met the requirements of the AAMI standard. After training with knowledge distillation (KD), the model achieved a mean absolute error and standard deviation of 2.94 ± 5.61 mmHg for SBP and 2.02 ± 3.60 mmHg for DBP. Conclusion Our results demonstrate the benefits of the knowledge distillation training method in reducing the number of parameters and improving the predictive accuracy of the blood pressure regression model.
更多查看译文
关键词
PPG,ECG,Knowledge distillation,Blood pressure
AI 理解论文
溯源树
样例
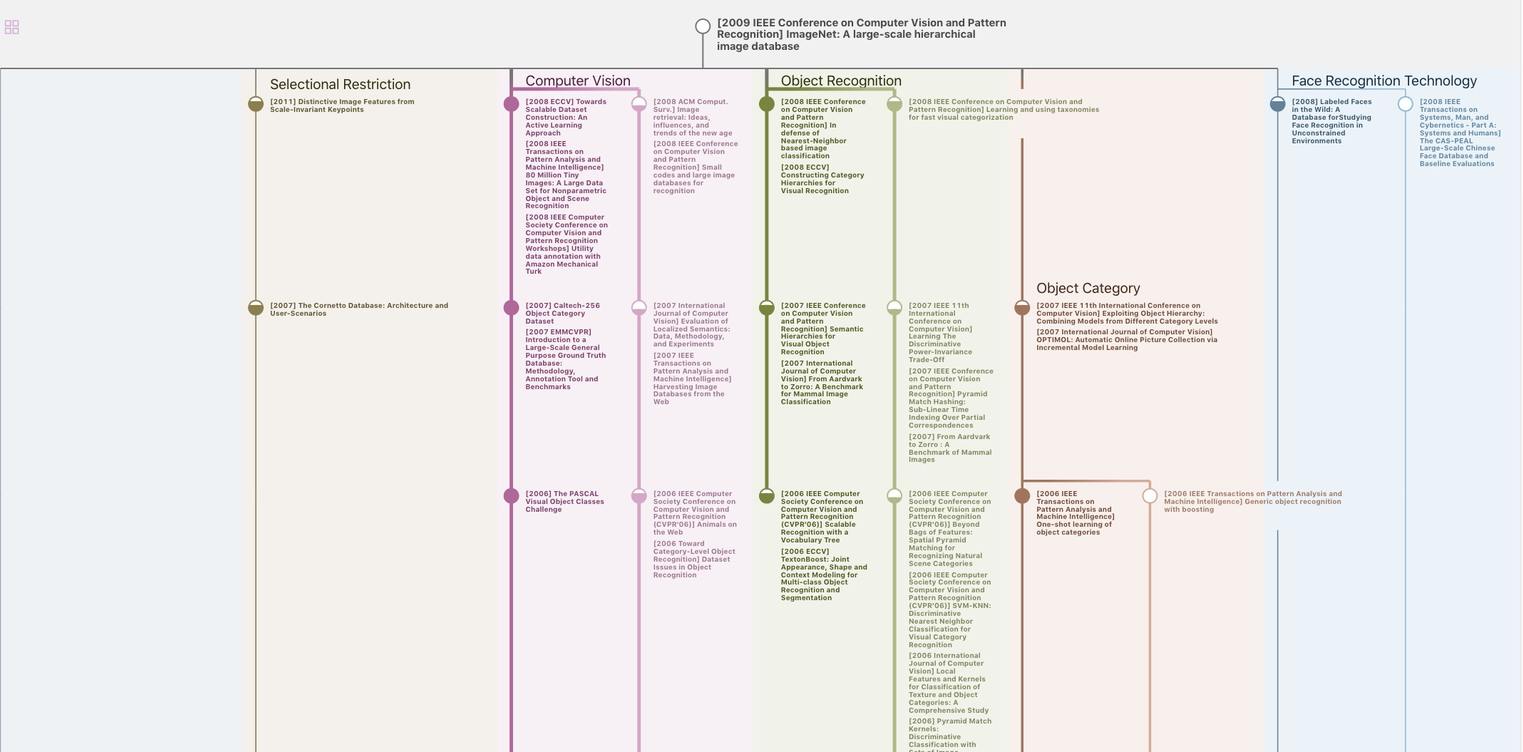
生成溯源树,研究论文发展脉络
Chat Paper
正在生成论文摘要