Advancing Noise-Resilient Twist Angle Characterization in Bilayer Graphene through Raman Spectroscopy via GAN-CNN Modeling
arxiv(2024)
摘要
In this study, we introduce an innovative methodology for robust twist angle
identification in bilayer graphene using Raman spectroscopy, featuring the
integration of generative adversarial network and convolutional neural network
(GAN-CNN). Our proposed approach showcases remarkable resistance to noise
interference, particularly in ultra-low Signal-to-Noise Ratio (SNR) conditions.
We demonstrate the GAN-CNN model's robust learning capability, even when SNR
reaches minimal levels. The model's exceptional noise resilience negates the
necessity for preprocessing steps, facilitating accurate classification, and
substantially reducing computational expenses. Empirical results reveal the
model's prowess, achieving heightened accuracy in twist angle identification.
Specifically, our GAN-CNN model achieves a test accuracy exceeding 99.9
recall accuracy of 99.9
spectra. This work not only contributes to the evolution of noise-resistant
spectral analysis methodologies but also provides crucial insights into the
application of advanced deep learning techniques for bilayer graphene
characterization through Raman spectroscopy. The findings presented herein have
broader implications for enhancing the precision and efficiency of material
characterization methodologies, laying the foundation for future advancements
in the field.
更多查看译文
AI 理解论文
溯源树
样例
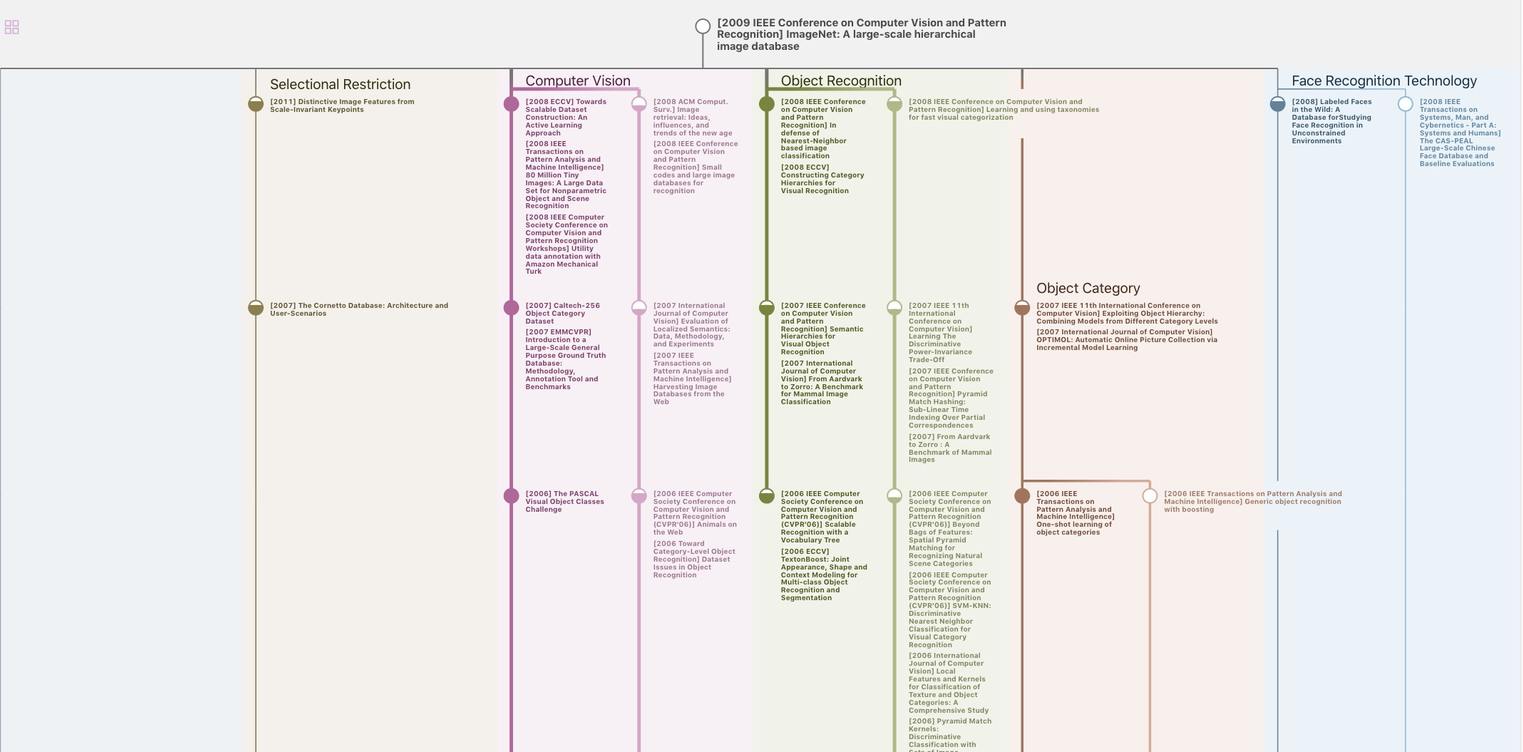
生成溯源树,研究论文发展脉络
Chat Paper
正在生成论文摘要