When to Grow? A Fitting Risk-Aware Policy for Layer Growing in Deep Neural Networks
AAAI 2024(2024)
Abstract
Neural growth is the process of growing a small neural network to a large network and has been utilized to accelerate the training of deep neural networks. One crucial aspect of neural growth is determining the optimal growth timing. However, few studies investigate this systematically. Our study reveals that neural growth inherently exhibits a regularization effect, whose intensity is influenced by the chosen policy for growth timing. While this regularization effect may mitigate the overfitting risk of the model, it may lead to a notable accuracy drop when the model underfits. Yet, current approaches have not addressed this issue due to their lack of consideration of the regularization effect from neural growth. Motivated by these findings, we propose an under/over fitting risk-aware growth timing policy, which automatically adjusts the growth timing informed by the level of potential under/overfitting risks to address both risks. Comprehensive experiments conducted using CIFAR-10/100 and ImageNet datasets show that the proposed policy achieves accuracy improvements of up to 1.3% in models prone to underfitting while achieving similar accuracies in models suffering from overfitting compared to the existing methods.
MoreTranslated text
Key words
CV: Image and Video Retrieval,ML: Deep Learning Algorithms
AI Read Science
Must-Reading Tree
Example
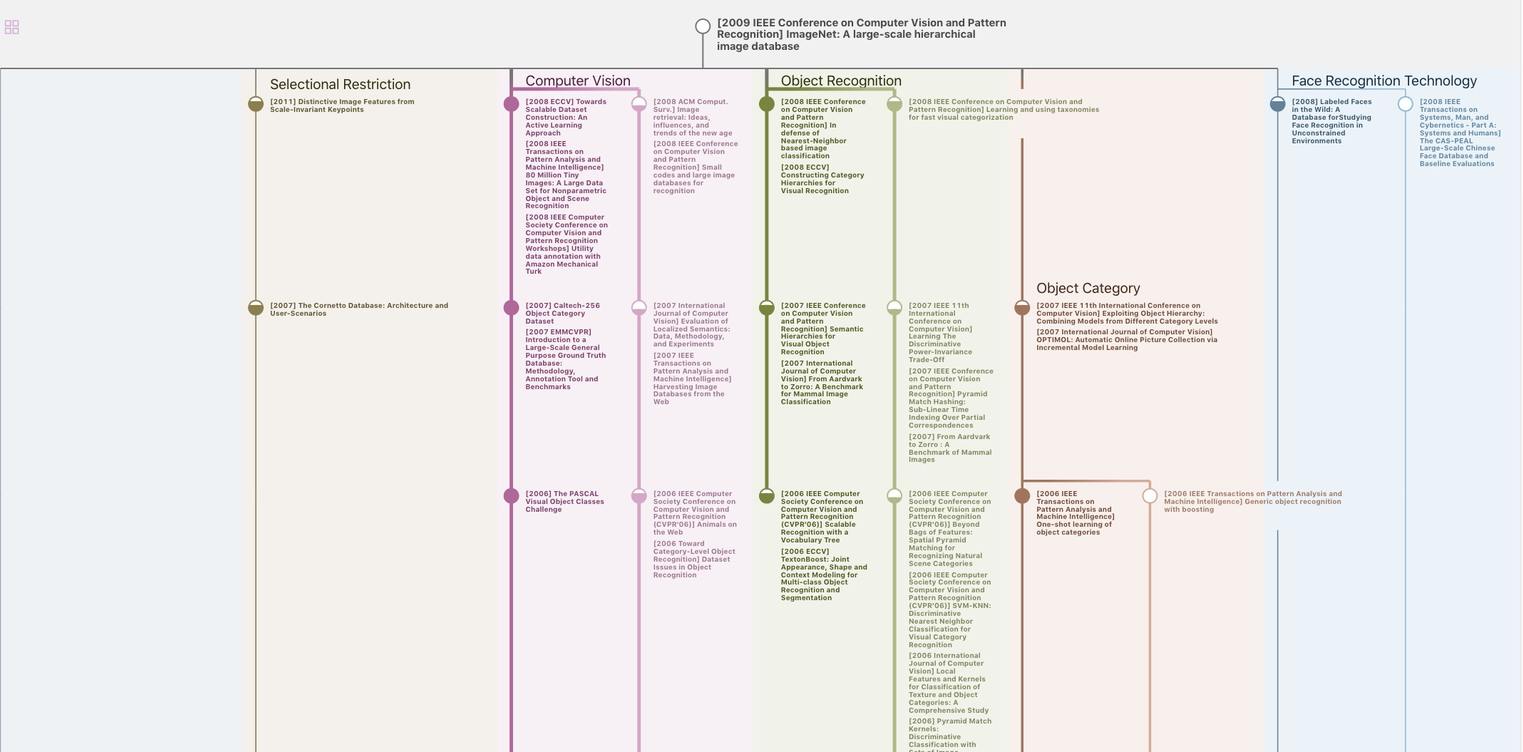
Generate MRT to find the research sequence of this paper
Chat Paper
Summary is being generated by the instructions you defined