UMIE: Unified Multimodal Information Extraction with Instruction Tuning
AAAI 2024(2024)
摘要
Multimodal information extraction (MIE) gains significant attention as the popularity of multimedia content increases. However, current MIE methods often resort to using task-specific model structures, which results in limited generalizability across tasks and underutilizes shared knowledge across MIE tasks. To address these issues, we propose UMIE, a unified multimodal information extractor to unify three MIE tasks as a generation problem using instruction tuning, being able to effectively extract both textual and visual mentions. Extensive experiments show that our single UMIE outperforms various state-of-the-art (SoTA) methods across six MIE datasets on three tasks. Furthermore, in-depth analysis demonstrates UMIE's strong generalization in the zero-shot setting, robustness to instruction variants, and interpretability. Our research serves as an initial step towards a unified MIE model and initiates the exploration into both instruction tuning and large language models within the MIE domain. Our code, data, and model are available at https://github.com/ZUCC-AI/UMIE.
更多查看译文
关键词
NLP: Information Extraction,NLP: Language Grounding & Multi-modal NLP
AI 理解论文
溯源树
样例
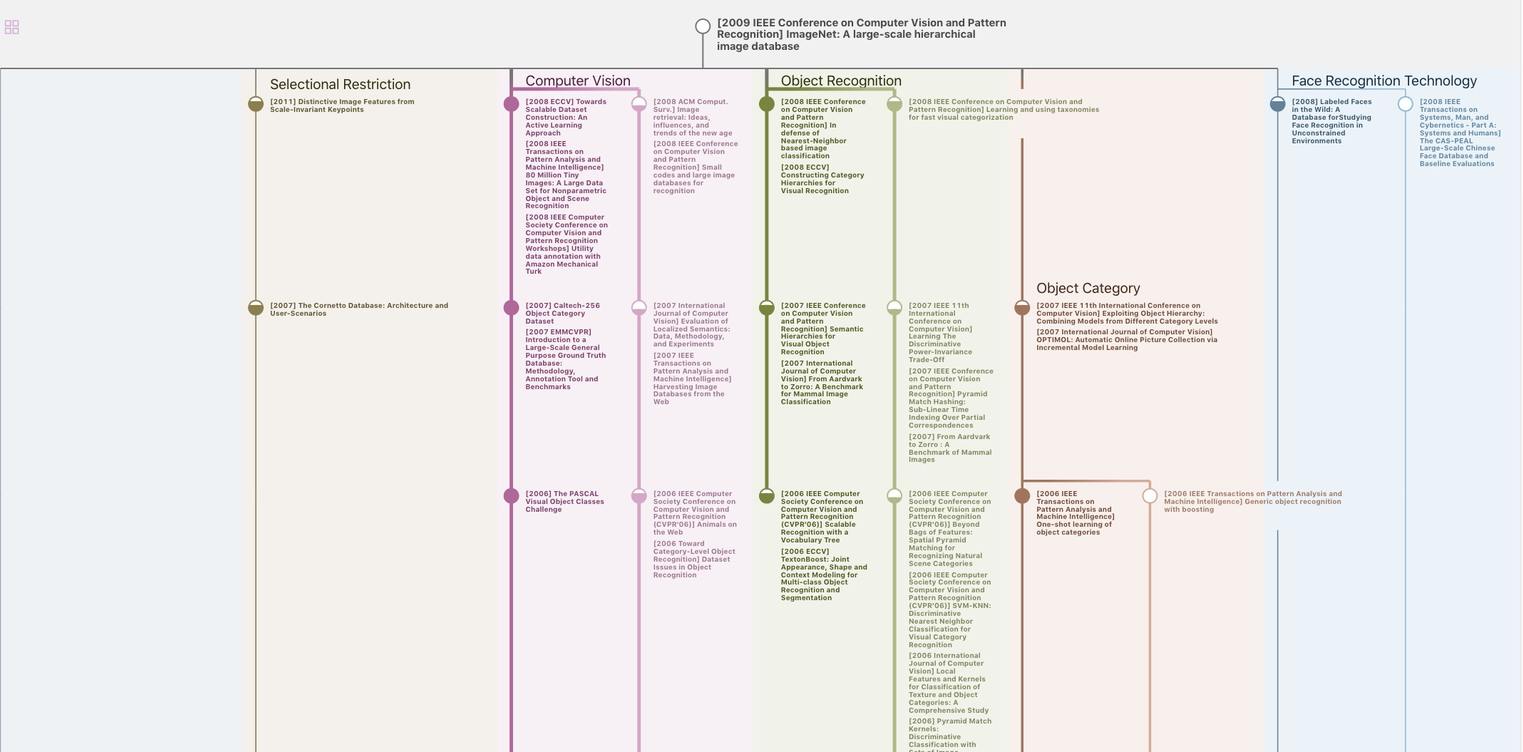
生成溯源树,研究论文发展脉络
Chat Paper
正在生成论文摘要