Reliability-Optimized User Admission Control for URLLC Traffic: A Neural Contextual Bandit Approach
CoRR(2024)
摘要
Ultra-reliable low-latency communication (URLLC) is the cornerstone for a
broad range of emerging services in next-generation wireless networks. URLLC
fundamentally relies on the network's ability to proactively determine whether
sufficient resources are available to support the URLLC traffic, and thus,
prevent so-called cell overloads. Nonetheless, achieving accurate
quality-of-service (QoS) predictions for URLLC user equipment (UEs) and
preventing cell overloads are very challenging tasks. This is due to dependency
of the QoS metrics (latency and reliability) on traffic and channel statistics,
users' mobility, and interdependent performance across UEs. In this paper, a
new QoS-aware UE admission control approach is developed to proactively
estimate QoS for URLLC UEs, prior to associating them with a cell, and
accordingly, admit only a subset of UEs that do not lead to a cell overload. To
this end, an optimization problem is formulated to find an efficient UE
admission control policy, cognizant of UEs' QoS requirements and cell-level
load dynamics. To solve this problem, a new machine learning based method is
proposed that builds on (deep) neural contextual bandits, a suitable framework
for dealing with nonlinear bandit problems. In fact, the UE admission
controller is treated as a bandit agent that observes a set of network
measurements (context) and makes admission control decisions based on
context-dependent QoS (reward) predictions. The simulation results show that
the proposed scheme can achieve near-optimal performance and yield substantial
gains in terms of cell-level service reliability and efficient resource
utilization.
更多查看译文
AI 理解论文
溯源树
样例
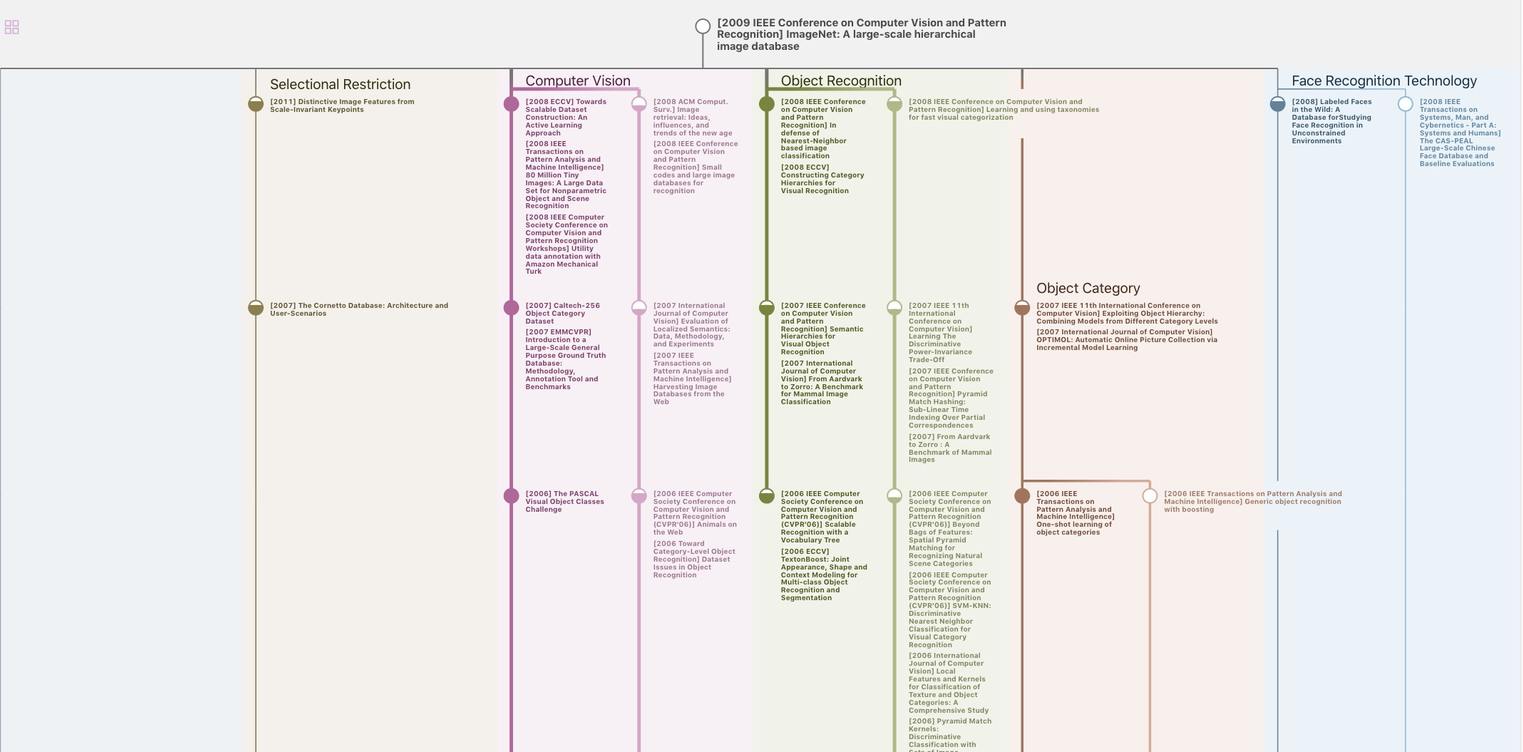
生成溯源树,研究论文发展脉络
Chat Paper
正在生成论文摘要