An automatic and unsupervised image mask acquisition method based on generative adversarial networks
SYSTEMS SCIENCE & CONTROL ENGINEERING(2024)
摘要
This paper proposes an unsupervised method for automatically labelling and obtaining image masks in defect detection. Since it is very labour intensive to acquire the image masks needed for deep learning (e.g. in semantic segmentation tasks) via manual labelling, we propose a method that utilize a generative adversarial network to obtain image masks automatically. Using this method, it is only necessary to input a considerable defect-free image to train. Then the proposed method can generate defect-free image samples like the input defect images, and the defect's location can be determined by comparing the input sample image containing defects with the generated sample image, thereby obtaining the input image mask. Our proposed method has been validated through experimental results, demonstrating its effectiveness. In addition to automatically and obtaining the required masks, our method achieved greater detection accuracy using the deep learning model Mask R-CNN compared with the manual labelling supervised method and a semi-supervised method.
更多查看译文
关键词
Deep learning,defect detection,Mask R-CNN
AI 理解论文
溯源树
样例
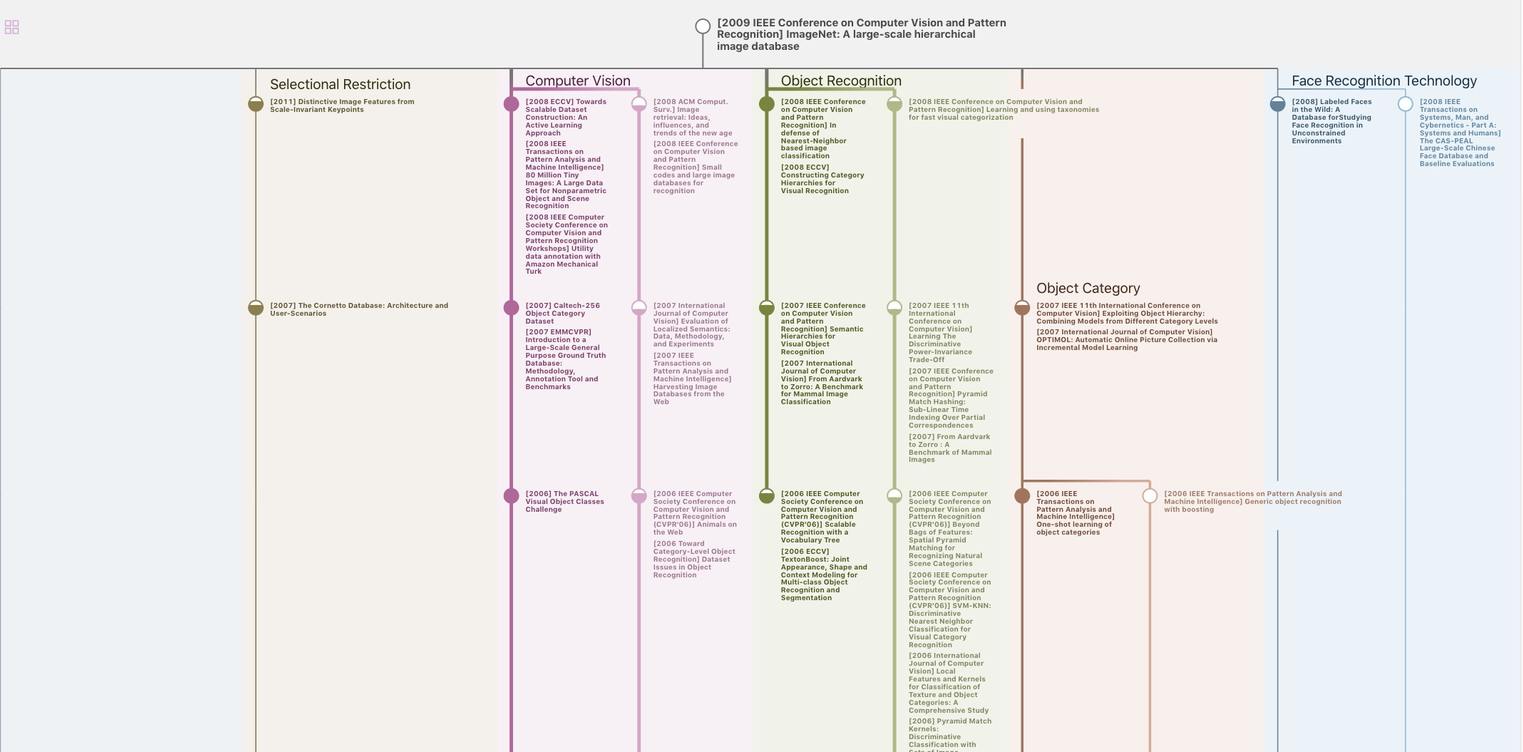
生成溯源树,研究论文发展脉络
Chat Paper
正在生成论文摘要