DBGSA: A novel data adaptive bregman clustering algorithm
ENGINEERING APPLICATIONS OF ARTIFICIAL INTELLIGENCE(2024)
摘要
Traditional clustering algorithms such as K -means are highly sensitive to the initial centroid selection and perform poorly on non -convex dataset. To address these problems, a novel data adaptive Bregman clustering algorithm (DBGSA) is proposed in this paper, which combines the universal gravitational algorithm to bring similar points closer in the dataset. In addition, we introduce the Bregman divergence generalized power mean information loss minimization to identify cluster centers and build a hyperparameter identification optimization model, which effectively solves the problems of manual adjustment and uncertainty in the improved dataset. Extensive experiments are conducted on four simulated datasets and six real datasets. The results demonstrate that DBGSA significantly improves the accuracy of various clustering algorithms by an average of 63.8% compared to the contrasted algorithms, making it applicable to diverse datasets.
更多查看译文
关键词
K-means,Data-driven,Bregman divergence,Hyperparameter adaptive optimization
AI 理解论文
溯源树
样例
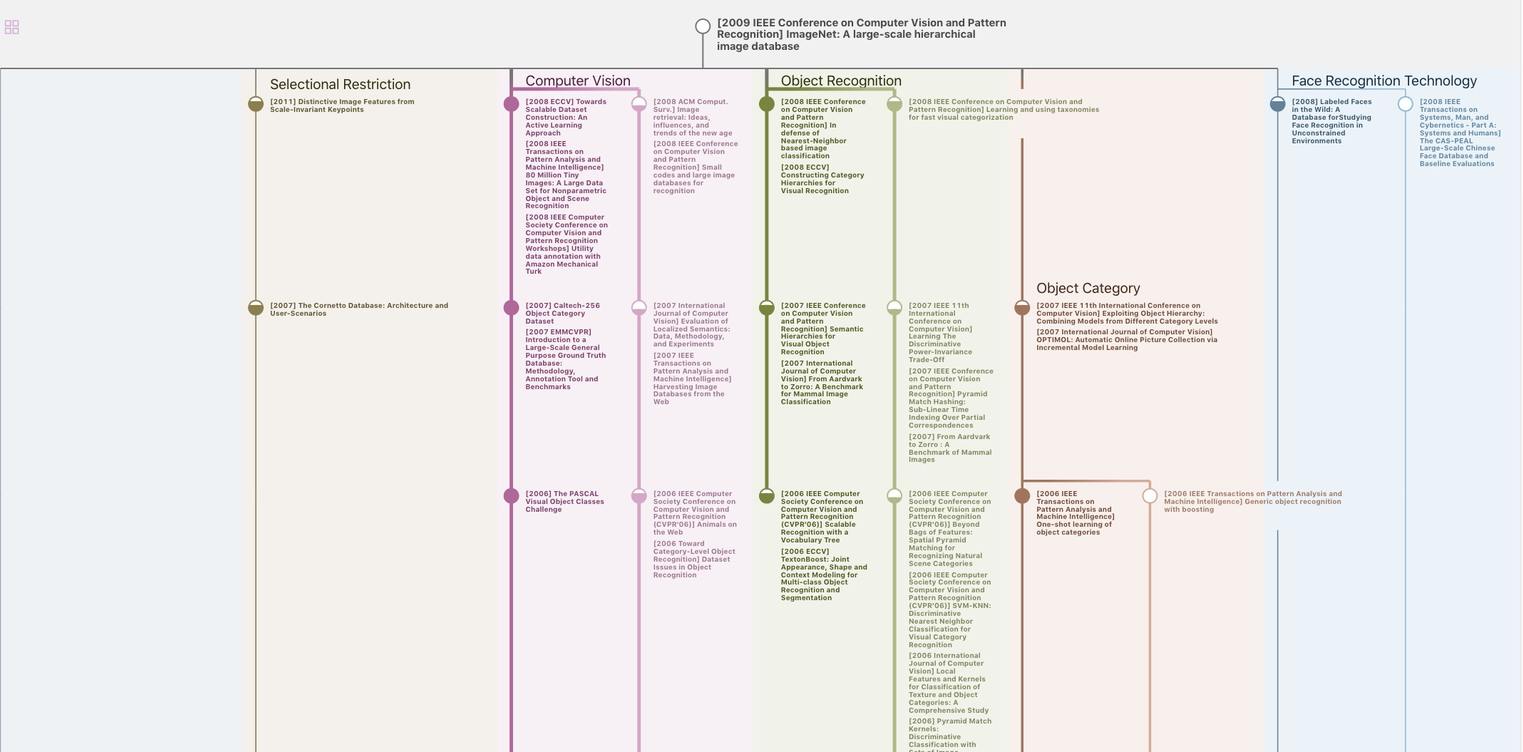
生成溯源树,研究论文发展脉络
Chat Paper
正在生成论文摘要