An interpretable machine learning model for stroke recurrence in patients with symptomatic intracranial atherosclerotic arterial stenosis
FRONTIERS IN NEUROSCIENCE(2024)
摘要
Background and objective Symptomatic intracranial atherosclerotic stenosis (SICAS) is the most common etiology of ischemic stroke and one of the main causes of high stroke recurrence. The recurrence of stroke is closely related to the prognosis of ischemic stroke. This study aims to develop a machine learning model based on high-resolution vessel wall imaging (HR-VWI) to predict the risk of stroke recurrence in SICAS.Methods This study retrospectively collected data from 180 SICAS stroke patients treated at the hospital between 2020.01 and 2022.01. Relevant imaging and clinical data were collected, and follow-up was conducted. The dataset was divided into a training set and a validation set in a ratio of 7:3. We employed the least absolute shrinkage and selection operator (LASSO) regression to perform a selection on the baseline data, laboratory tests, and neuroimaging data generated by HR-VWI scans collected from the training set. Finally, five machine learning techniques, including logistic regression model (LR), support vector machine (SVM), Gaussian naive Bayes (GNB), Complement naive Bayes (CNB), and k-nearest neighbors algorithm (kNN), were employed to develop a predictive model for stroke recurrence. Shapley Additive Explanation (SHAP) was used to provide visualization and interpretation for each patient. The model's effectiveness was evaluated using average accuracy, sensitivity, specificity, precision, f1 score, PR curve, calibration curve, and decision curve analysis.Results LASSO analysis revealed that "history of hypertension," "homocysteine level," "NWI value," "stenosis rate," "intracranial hemorrhage," "positive remodeling," and "enhancement grade" were independent risk factors for stroke recurrence in SICAS patients. In 10-fold cross-validation, the area under the curve (AUC) ranged from 0.813 to 0.912 in ROC curve analysis. The area under the precision-recall curve (AUPRC) ranged from 0.655 to 0.833, with the Gaussian Naive Bayes (GNB) model exhibiting the best ability to predict stroke recurrence in SICAS. SHAP analysis provided interpretability for the machine learning model and revealed essential factors related to the risk of stroke recurrence in SICAS.Conclusion A precise machine learning-based prediction model for stroke recurrence in SICAS has been established to assist clinical practitioners in making clinical decisions and implementing personalized treatment measures.
更多查看译文
关键词
stroke recurrence,HR-VWI,machine learning,SICAS,plaque
AI 理解论文
溯源树
样例
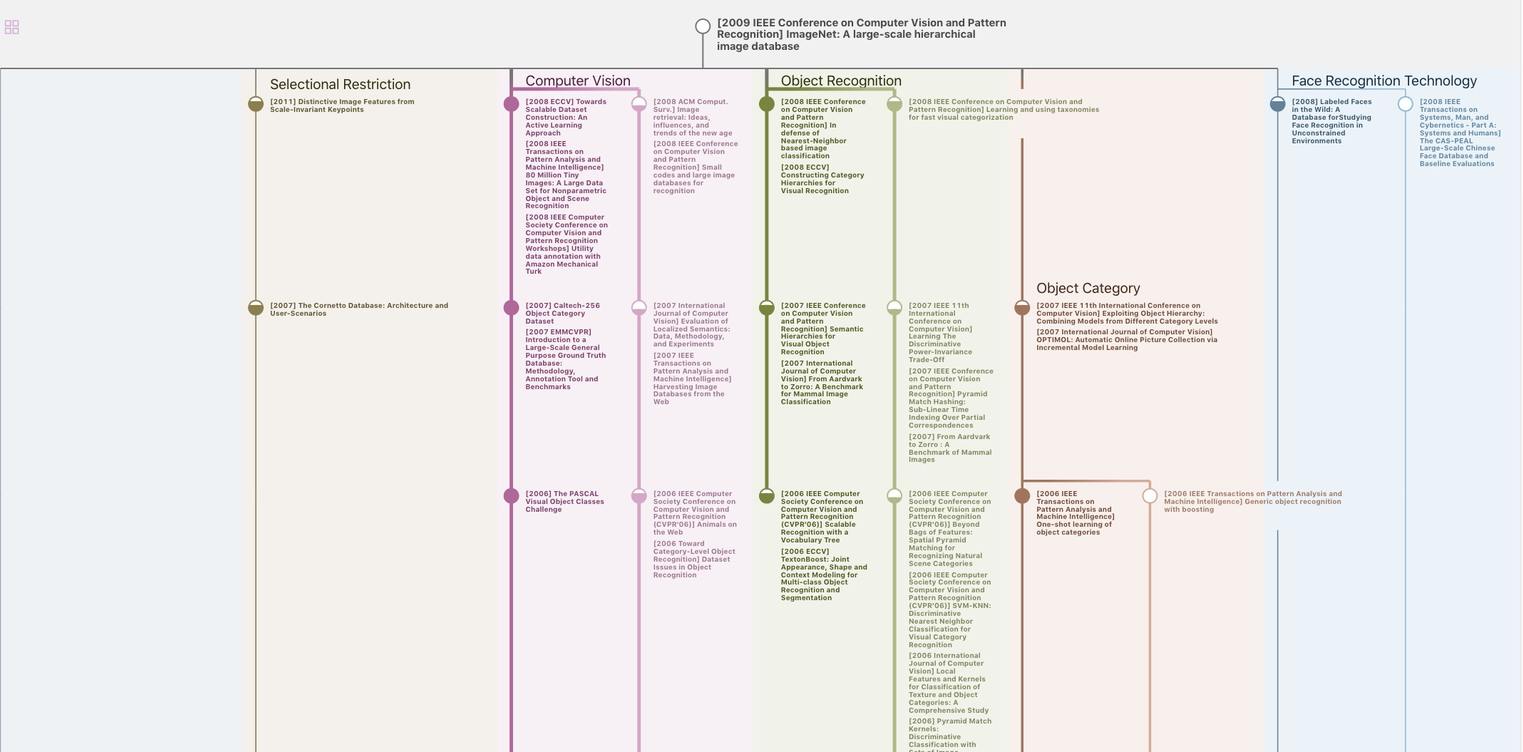
生成溯源树,研究论文发展脉络
Chat Paper
正在生成论文摘要