MATNet: a Multi-Attention Transformer Network for Nuclei Segmentation in Thymoma Histopathology Images
Multimedia Tools and Applications(2024)
Key words
Thymoma histopathology image,Nuclei segmentation,Transformer,Self-attention,Cross-attention
AI Read Science
Must-Reading Tree
Example
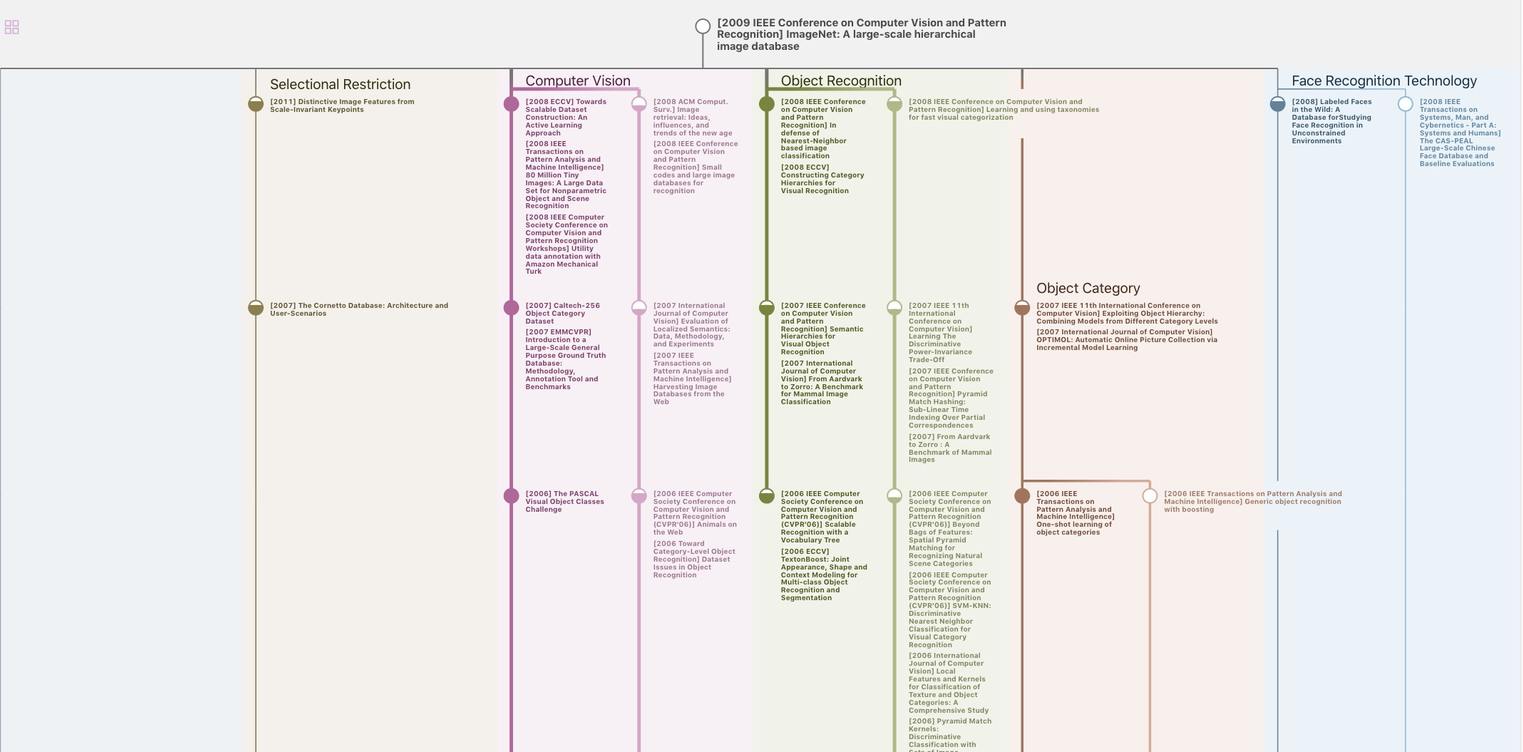
Generate MRT to find the research sequence of this paper
Chat Paper
Summary is being generated by the instructions you defined