RSMformer: an efficient multiscale transformer-based framework for long sequence time-series forecasting
Applied Intelligence(2024)
Abstract
Long sequence time-series forecasting (LSTF) is a significant and challenging task. Many real-world applications require long-term forecasting of time series. In recent years, Transformer-based models have emerged as a promising solution for addressing LSTF tasks. Nevertheless, the model’s performance is constrained by several issues, including the single time scale, the quadratic calculation complexity of the self-attention mechanism, and the high memory occupation. Based on the limitations mentioned above, we propose a novel approach in this paper, namely the multiscale residual sparse attention model RSMformer, built upon the Transformer architecture. Firstly, a residual sparse attention (RSA) mechanism is devised to select dominant queries for computation, utilizing the attention sparsity criterion. This approach effectively reduces the computational complexity to 𝒪 ( L log L ). Secondly, we employ a multiscale forecasting strategy to iteratively refine the accuracy of prediction results at multiple scales by utilizing up-and-down sampling techniques and cross-scale centralization schemes, which effectively capture the temporal dependencies at different time scales. Extensive experiments on six publicly available datasets show that RSMformer performs significantly better than the compared state-of-the-art benchmarks and excels in the LSTF tasks.
MoreTranslated text
Key words
Long sequence time-series forecasting,Transformer,Residual sparse attention,Multiscale forecasting
AI Read Science
Must-Reading Tree
Example
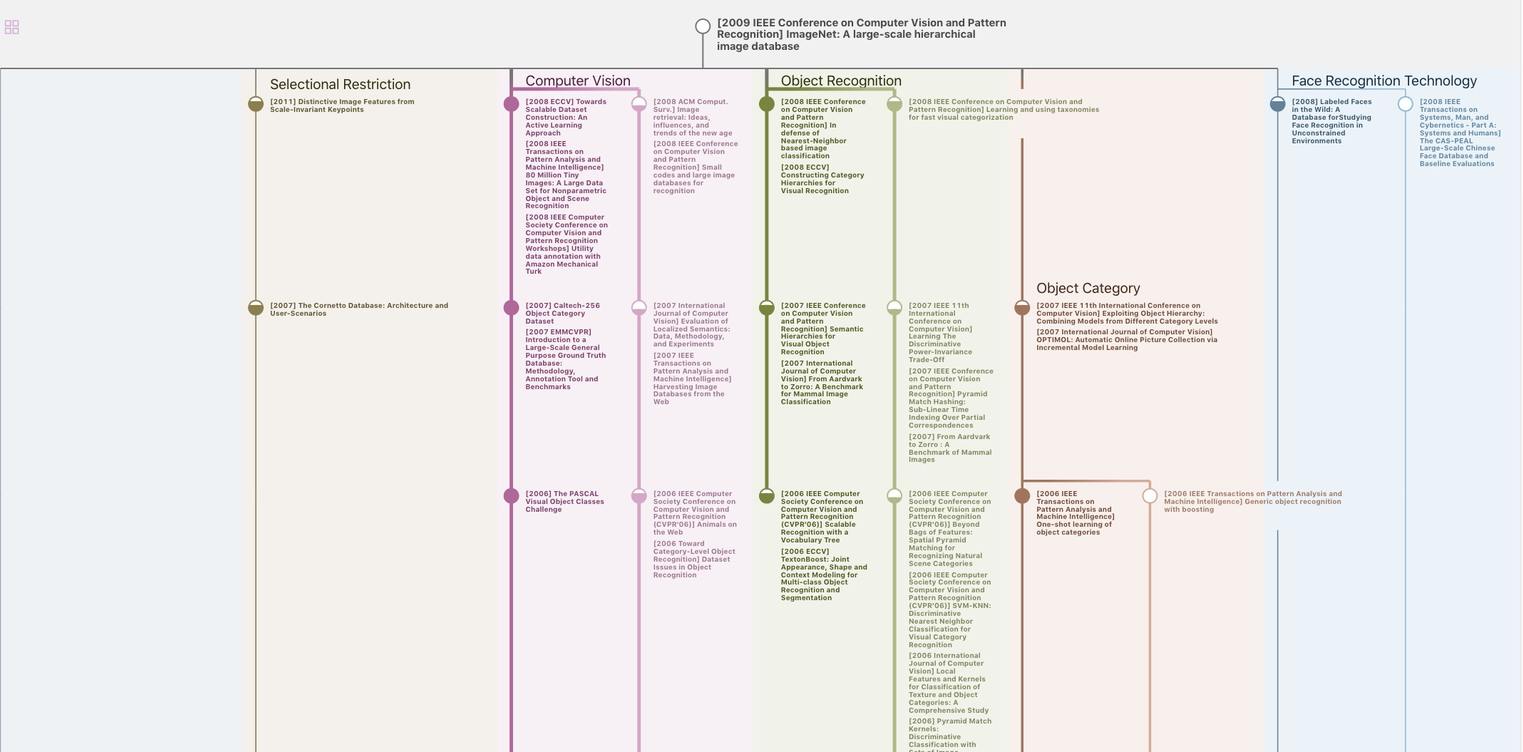
Generate MRT to find the research sequence of this paper
Chat Paper
Summary is being generated by the instructions you defined