MHRE: Multivariate link prediction method for medical hyper-relational facts
Applied Intelligence(2024)
Abstract
As hyper-relational facts continue to proliferate within knowledge graphs, link prediction on binary relations has become inadequate, while link prediction on hyper-relations has emerged as a research hotspot. Existing methods typically employ n-ary tuples, primary triple with auxiliary descriptions, or hypergraphs to represent hyper-relational facts and conduct link prediction. However, medical hyper-relational facts are more intricate and frequently lack multiple components, which presents challenges for current methods in conveying their structure, semantics, and predicting multiple missing elements simultaneously. To address these issues, in this paper, we introduce MHRE, the pioneering link prediction method specifically designed for medical hyper-relational facts. Initially, we represent medical hyper-relational facts as a heterogeneous multi-relational directed graph with hyper-relations at its core to depict both its structure and implicit semantics. Next, we develop a role-aware graph attention mechanism network to acquire distributed vector representations of entities and relations within the graph. Importantly, it fine-tunes the semantic weights of different components within hyper-relational facts by incorporating neighboring nodes and role information through learning. Lastly, we devise a prediction module based on self-attention mechanisms, enabling the simultaneous prediction of multiple missing elements within a medical hyper-relational fact. We conduct experiments using publicly available datasets, such as JF17K, WikiPeople, and their adapted versions, alongside a proprietary medical dataset. We compare MHRE with state-of-the-art baselines and further conduct ablation studies and parameter analysis. The experimental results confirm the efficacy and superiority of MHRE. In a range of benchmark tests involving hyper-relational facts, MHRE consistently outperforms current state-of-the-art methods. Graphical abstract
MoreTranslated text
Key words
Hyper-relational knowledge graph,Medical knowledge graph,Link prediction,Knowledge graph completion,Knowledge representation learning
AI Read Science
Must-Reading Tree
Example
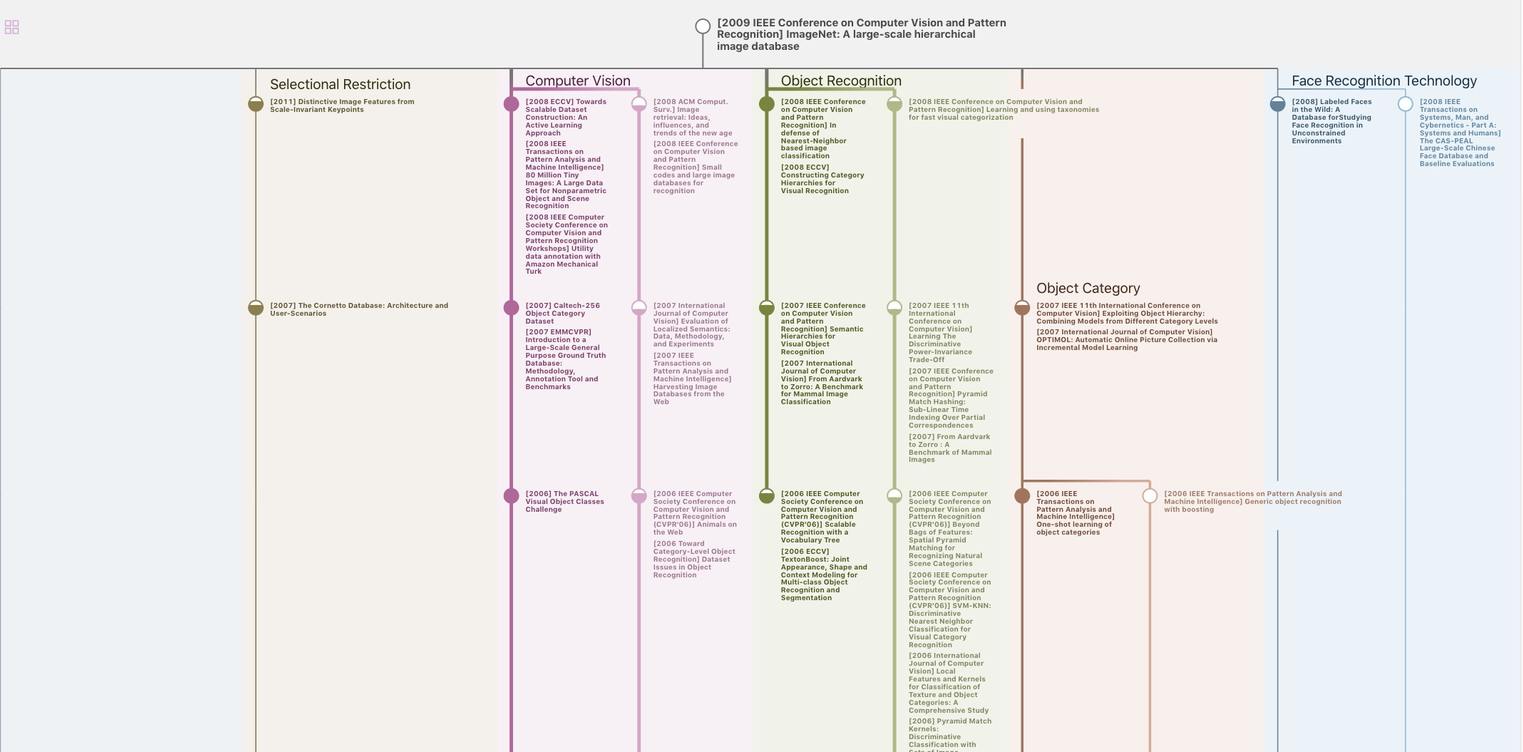
Generate MRT to find the research sequence of this paper
Chat Paper
Summary is being generated by the instructions you defined