Studying the Impact of Sampling in Highly Frequent Time Series
PROGRESS IN ARTIFICIAL INTELLIGENCE, EPIA 2023, PT I(2023)
摘要
Nowadays, all kinds of sensors generate data, and more metrics are being measured. These large quantities of data are stored in large data centers and used to create datasets to train Machine Learning algorithms for most different areas. However, processing that data and training the Machine Learning algorithms require more time, and storing all the data requires more space, creating a Big Data problem. In this paper, we propose simple techniques for reducing large time series datasets into smaller versions without compromising the forecasting capability of the generated model and, simultaneously, reducing the time needed to train the models and the space required to store the reduced sets. We tested the proposed approach in three public and one private dataset containing time series with different characteristics. The results show, for the datasets studied that it is possible to use reduced sets to train the algorithms without affecting the forecasting capability of their models. This approach is more efficient for datasets with higher frequencies and larger seasonalities. With the reduced sets, we obtain decreases in the training time between 40 and 94% and between 46 and 65% for the memory needed to store the reduced sets.
更多查看译文
关键词
Time series,Forecasting,Data reduction,Numerosity reduction,Big data,Machine learning,Holt-winters
AI 理解论文
溯源树
样例
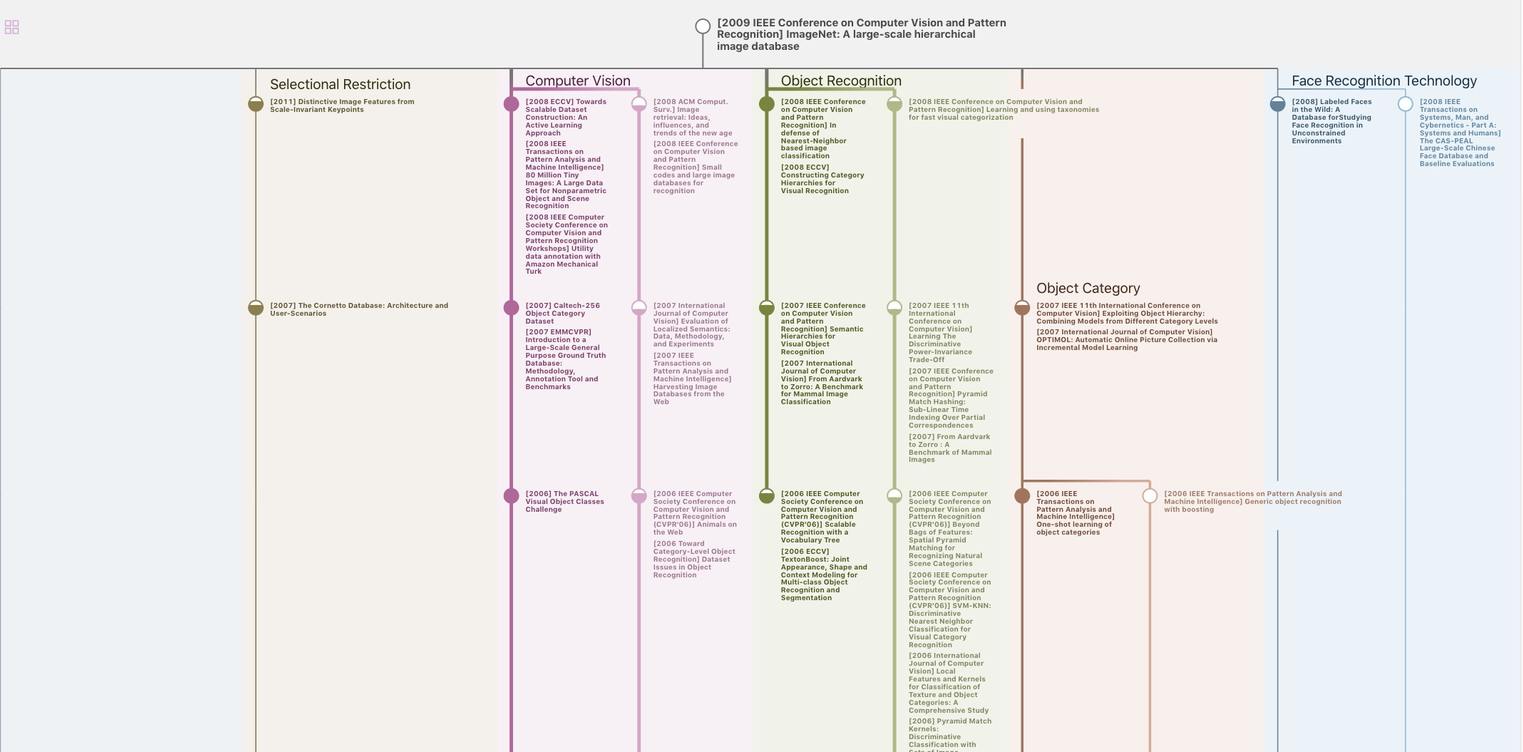
生成溯源树,研究论文发展脉络
Chat Paper
正在生成论文摘要