Retrieved Generative Captioning for Medical Images
20TH INTERNATIONAL CONFERENCE ON CONTENT-BASED MULTIMEDIA INDEXING, CBMI 2023(2023)
摘要
Understanding the content of medical images and mapping it into text is a very trending topic in intersection of two main domains; computer vision and natural language processing. This is known as medical image captioning, which plays a vital role in developing automatic systems for diagnosis purposes. Recent research in the medical field provided promising results for both deep-learning based and retrieval-based models for image captioning. However, each one of them has its own drawbacks, that can be overcome if combined. In addition, existing diagnosis systems are still not able to provide enough explanation about the findings, which might be similar to what a physician can deliver. In this regard, we present in this paper a combination of a generative deep-learning based method and a retrieval-based model for medical image captioning. First, we train an attention-based encoder-decoder model to generate new captions for given medical images. Then, we fit the generated caption from the generative model to the retrieval-based model, which retrieves the most similar caption from the training database. This multi-stage approach allows us to generate most important words of the caption (with the generative model) and then search for the most close caption that includes such words (with the retrieval-based model). Another way of combining both models is by selecting at each time the caption with highest score among generated and retrieved captions. We evaluate our proposed model on the medical ROCO dataset for which we achieved a BLEU-4 score of 07.89 for the radiology class and 03.19 for the out-of-class data, for the multi-stage model. Similarly, best results were achieved for the fused model (predicted caption is the best among generated and retrieved) where we obtain a BLEU-4 values of 18.61 for the radiology class and 13.28 for the out-of-class data. Even though our results seem to be low, they outperformed the state-of-the-art results on the same dataset and could be further improved.
更多查看译文
关键词
Image Captioning,Medical Images,Neural Networks,Retrievalbased,Captioning,Generative-based Captioning
AI 理解论文
溯源树
样例
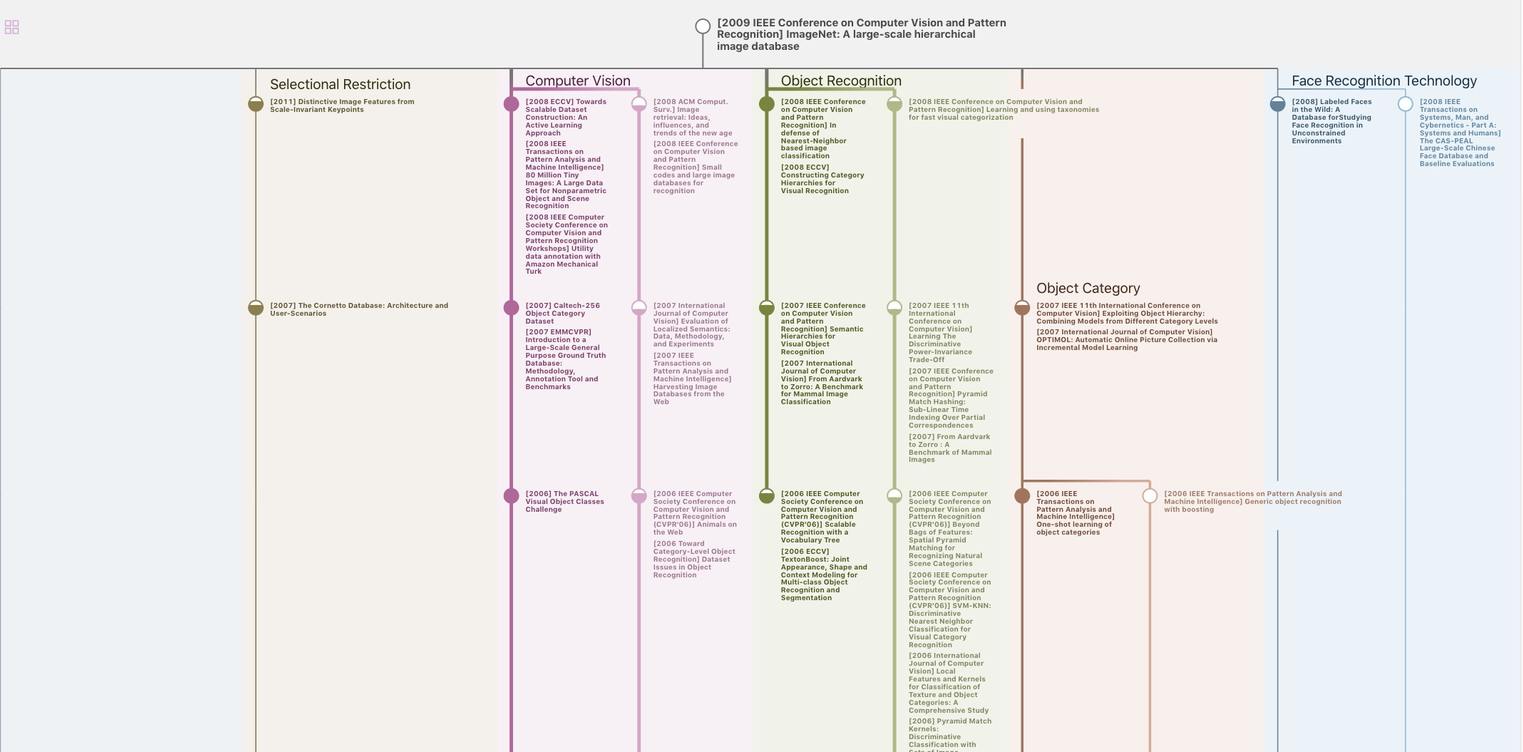
生成溯源树,研究论文发展脉络
Chat Paper
正在生成论文摘要