Optimal prediction of positive-valued spatial processes: Asymmetric power-divergence loss
Spatial Statistics(2024)
摘要
This article studies the use of asymmetric loss functions for the optimal prediction of positive-valued spatial processes. We focus on the family of power-divergence loss functions with properties such as continuity, convexity, connection to well known divergence measures, and the ability to control the asymmetry and behaviour of the loss function via a power parameter. The properties of power-divergence loss functions, optimal power-divergence (OPD) spatial predictors, and related measures of uncertainty quantification are studied. In addition, we examine in general the notion of asymmetry in loss functions defined for positive-valued spatial processes and define an asymmetry measure, which we apply to the family of power-divergence loss functions and other common loss functions. The paper concludes with a simulation study comparing the optimal power-divergence predictor to predictors derived from other common loss functions. Finally, we illustrate OPD spatial prediction on a dataset of zinc measurements in the soil of a floodplain of the Meuse River, Netherlands.
更多查看译文
关键词
Spatial prediction,Decision theory,Power-divergence,ELJ,ELP
AI 理解论文
溯源树
样例
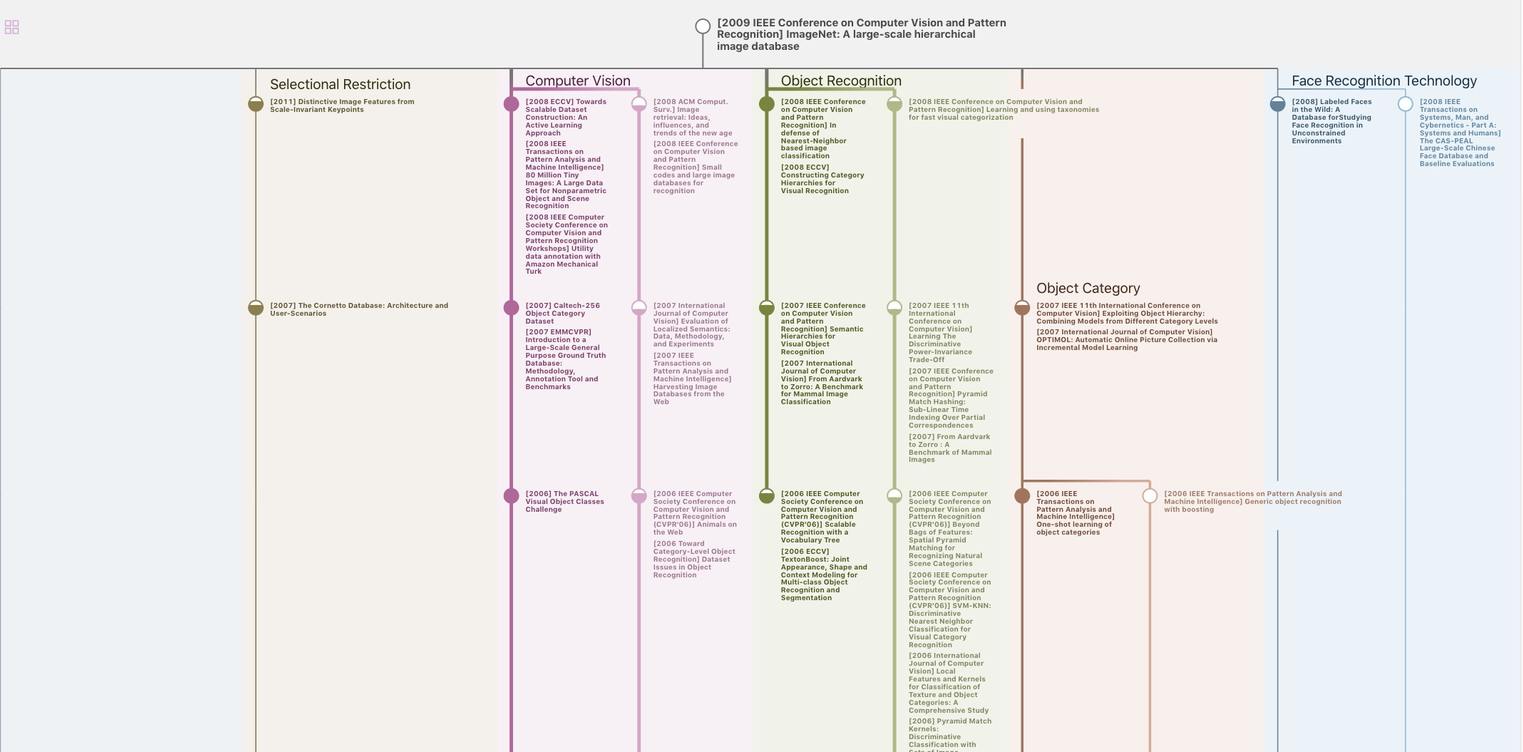
生成溯源树,研究论文发展脉络
Chat Paper
正在生成论文摘要