Fairness in Predictive Learning Analytics: A Case Study in Online STEM Education.
2023 IEEE Frontiers in Education Conference (FIE)(2023)
摘要
In this study, we investigate the fairness of predictive models in online courses. Our focus is on STEM courses and the prediction of underperforming students. The analysis of the model's fairness is dedicated to students' protected information: gender and age. The Open University Learning Analytics Dataset is selected for this study and two moments of prediction were created for the predictive models: before the midterm and two weeks before the final. The fairness of prediction models is assessed under three conditions: 1) models incorporating students' demographics and interactions with the virtual learning environment, 2) models relying solely on students' interactions with the online learning environment, and 3) models with imposed constraints on age and gender. The results show that hiding students' protected information lowers the fairness of the models. Contrary to that, using these protected attributes to enforce constraints improved fairness while still having good overall accuracy.
更多查看译文
关键词
Fairness,Adversarial debiasing,Online learning,Predictive models,Learning analytics
AI 理解论文
溯源树
样例
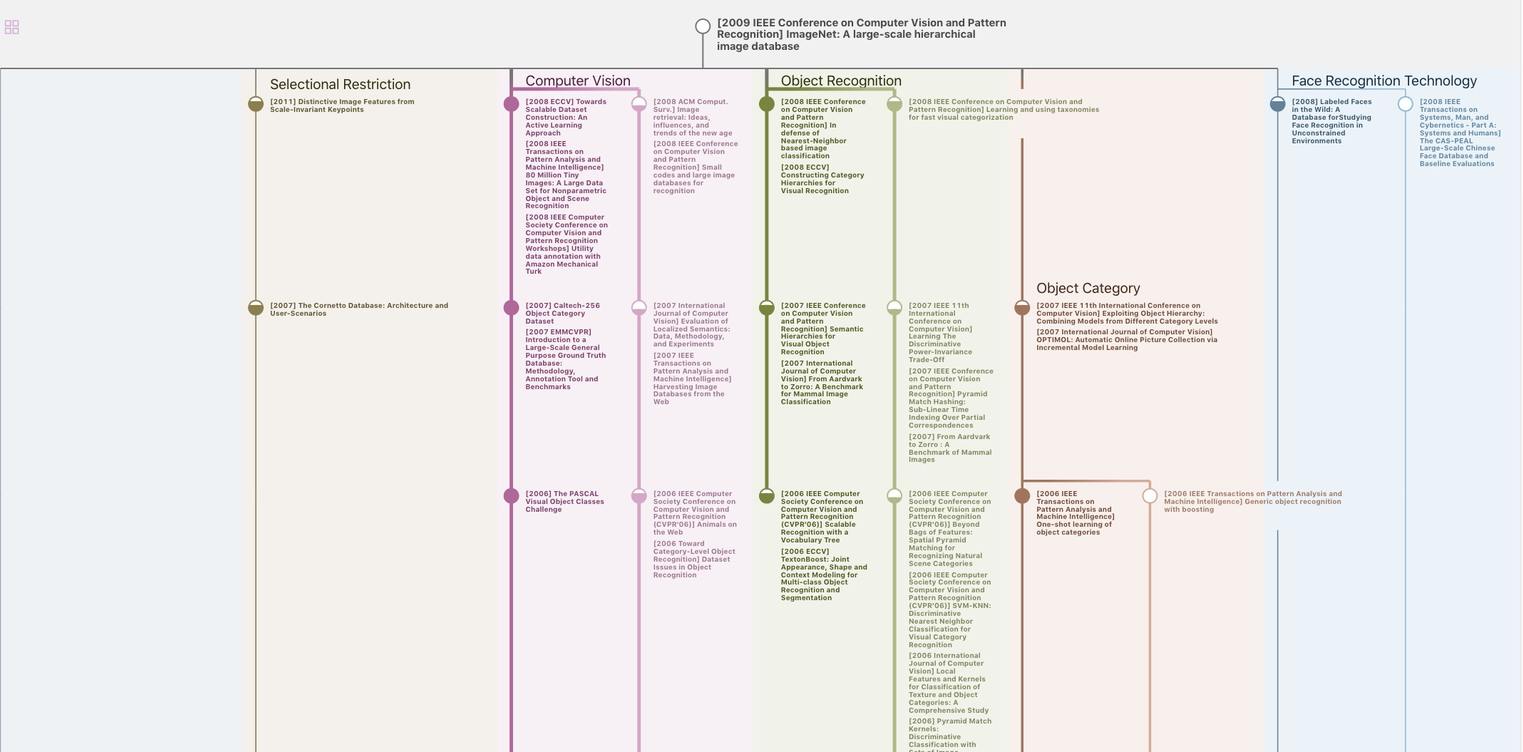
生成溯源树,研究论文发展脉络
Chat Paper
正在生成论文摘要