Enhancing pyrolysis process monitoring and prediction for biomass: A machine learning approach
FUEL(2024)
摘要
Biomass pyrolysis presents a viable solution to address the increasing global energy demand, and substantial efforts have been devoted worldwide to monitoring the conversion process through thermogravimetric experiments. In this study, the machine learning (ML) algorithm was employed to simulate and predict the temperature corresponding to specific conversion degrees (T alpha) by utilizing the compositional properties of the feedstock and heating rate as input variables. A dataset consisting of 1750 entries was collected from literature and deployed to construct neural network (NN) and random forest (RF) algorithms. After hyperparameter optimization, the RF model, equipped with 13 estimators and a maximum tree depth of 4, exhibited impressive accuracy in predicting T alpha, yielding an R2 value of 0.9462 and an RMES of 14.63 K. The subsequent feature importance analysis revealed that the conversion degree was the predominant influencing factor for T alpha, while the compositional properties of biomass accounted for 32.3 % of the overall influence. Validation experiments conducted using unexploited biomass further confirmed the robustness of the developed model. This research successfully demonstrated the feasibility of an ML approach in predicting reaction temperature based on preliminary feedstock analysis and desired pyrolysis progress, effectively eliminating the necessity for repetitive experiments. Furthermore, it provided valuable references for future ML research and applications in related fields.
更多查看译文
关键词
Biomass pyrolysis,Conversion degree,Reaction temperature,Machine learning,Composition characteristics
AI 理解论文
溯源树
样例
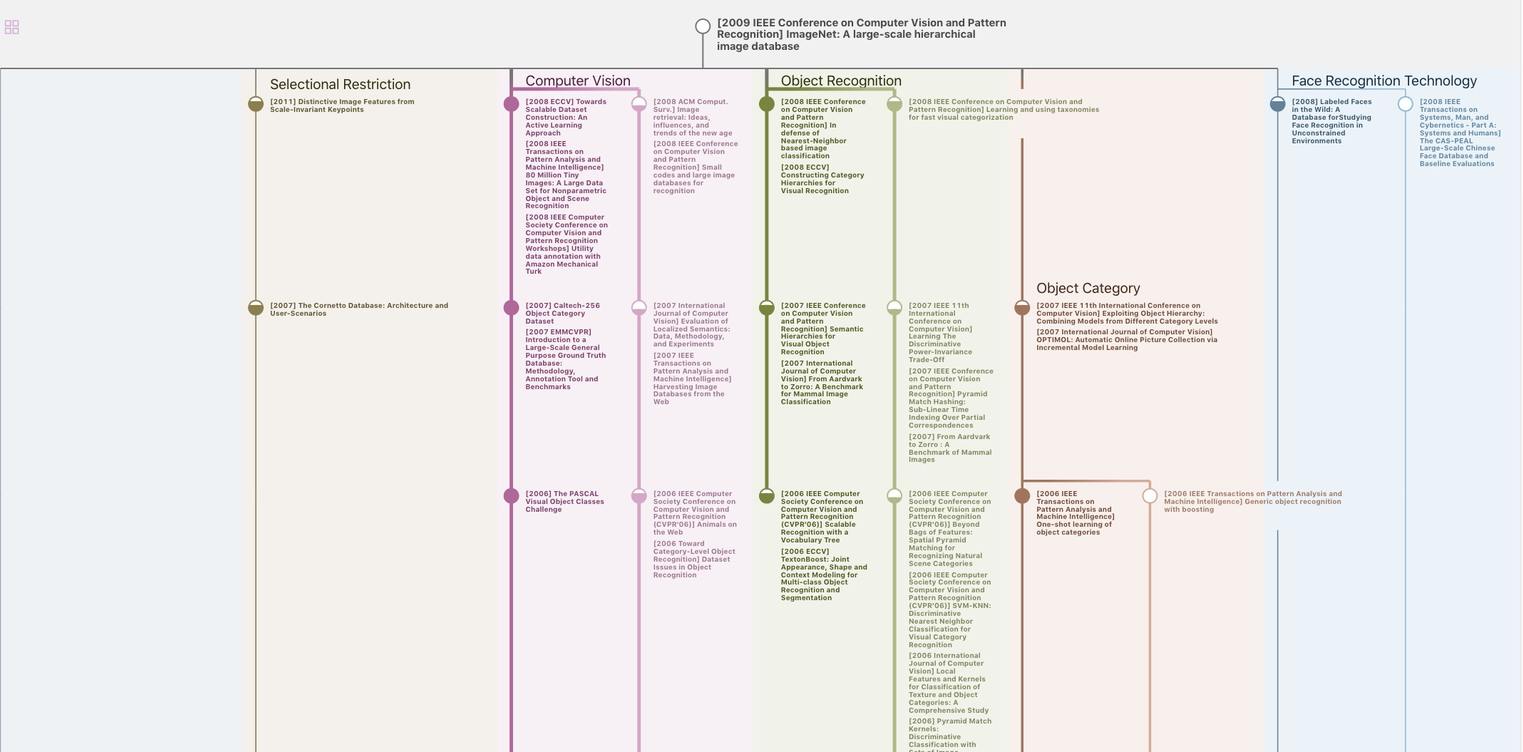
生成溯源树,研究论文发展脉络
Chat Paper
正在生成论文摘要