Closed-Loop Training for Projected GAN
IEEE SIGNAL PROCESSING LETTERS(2024)
摘要
Projected GAN, a pre-trained GAN, has been found to perform well in generating images with only a few training samples. However, it struggles with extended training, which may lead to decreased performance over time. This is because the pre-trained discriminator consistently surpasses the generator, creating an unstable training environment. In this work, we propose a solution to this issue by introducing closed-loop control (CLC) into the dynamics of Projected GAN, stabilizing training, and improving generation performance. Our proposed method consistently reduces the Frechet Inception Distance (FID) of the previous methods; for example, it reduces the FID of Projected GAN by 4.31 on the Obama dataset. Our finding is fundamental and can be used in other pre-trained GANs.
更多查看译文
关键词
Generative adversarial networks,Training,Transfer functions,Feature extraction,Generators,Image synthesis,Frequency-domain analysis,control theory,few-shot image generation
AI 理解论文
溯源树
样例
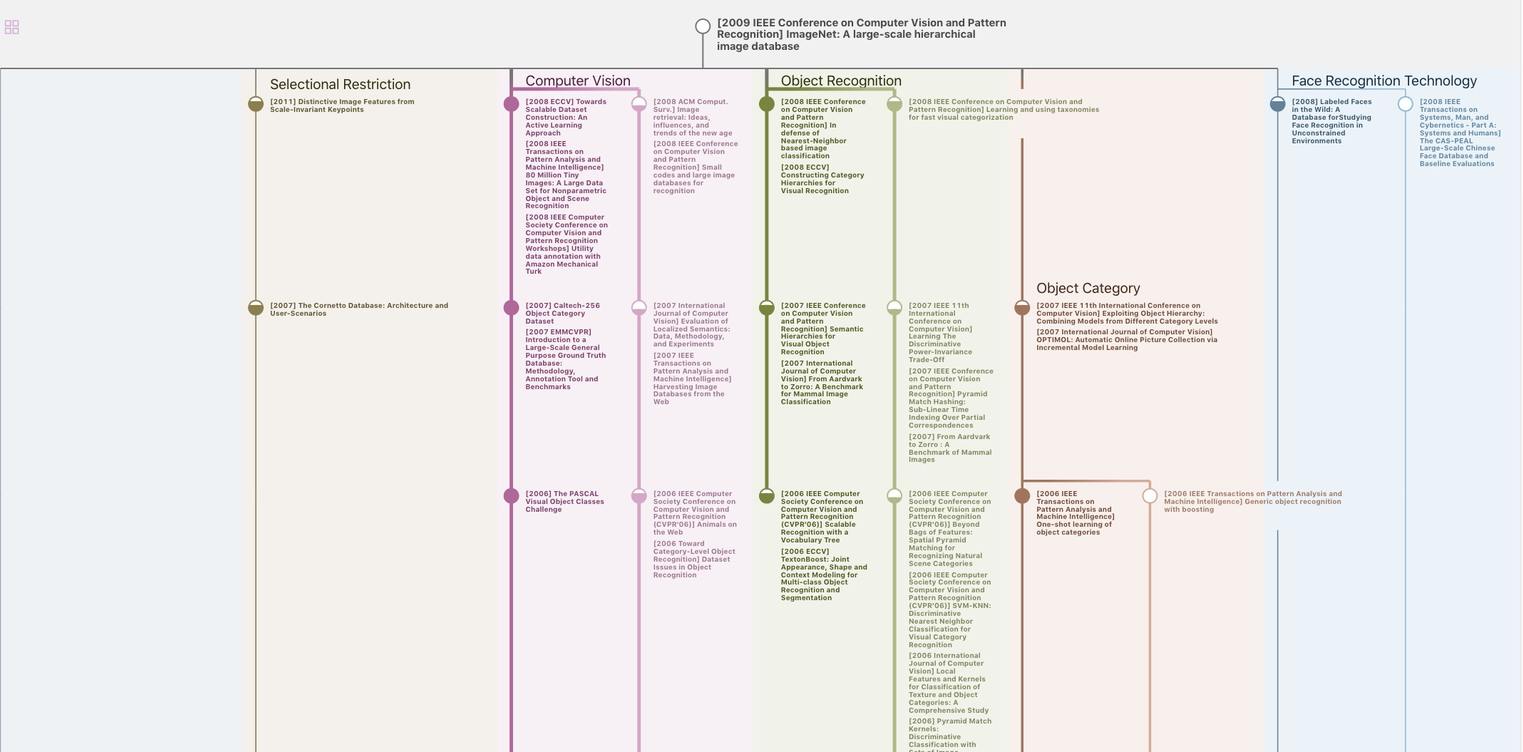
生成溯源树,研究论文发展脉络
Chat Paper
正在生成论文摘要