RA-MMIR : Multi-modal image registration by Robust Adaptive Variation Attention Gauge Field
INFORMATION FUSION(2024)
摘要
Multi-modal image registration finds extensive application in high-level vision tasks. Especially in adverse conditions, multimodal image registration and fusion is powerful for high-level visual analysis, high-level visual tasks commonly prioritize the analysis of crucial target regions within images, however precise multi-modal image registration remains a challenge. To address this issue, we rethought the collaboration between image registration and high-level visual tasks, and propose a Robust Adaptive Variation Attention Gauge Field registration framework that allows for flexible attention to both the target regions and global areas. Among them, in order to improve the robustness and timeliness of the algorithm in extracting and describing target regions or global areas features in adverse conditions, we propose a Robust Adaptive Variation Attention for building the gauge field, and in order to make robust adaptive parameters to reach the global optimum, we propose a Quasi-Simulated Annealing method based on mini-batch. To make the spatial transformation better fit feature matching and image registration, we design a deep learning and modeling approach based on spatial similarity metrics. In high-level visual tasks-based experiments such as image fusion, object detection, and 3D reconstruction, our method had the best collaborative performance and showed the best registration results under adverse conditions. The code is at https://github.com/JuiHuiQ/RA-MMIR.
更多查看译文
关键词
Multi-modal image registration,Adverse conditions,Target regions,Robust adaptive,Variational expectation maximization,Attention Gauge Field,Similarity metrics
AI 理解论文
溯源树
样例
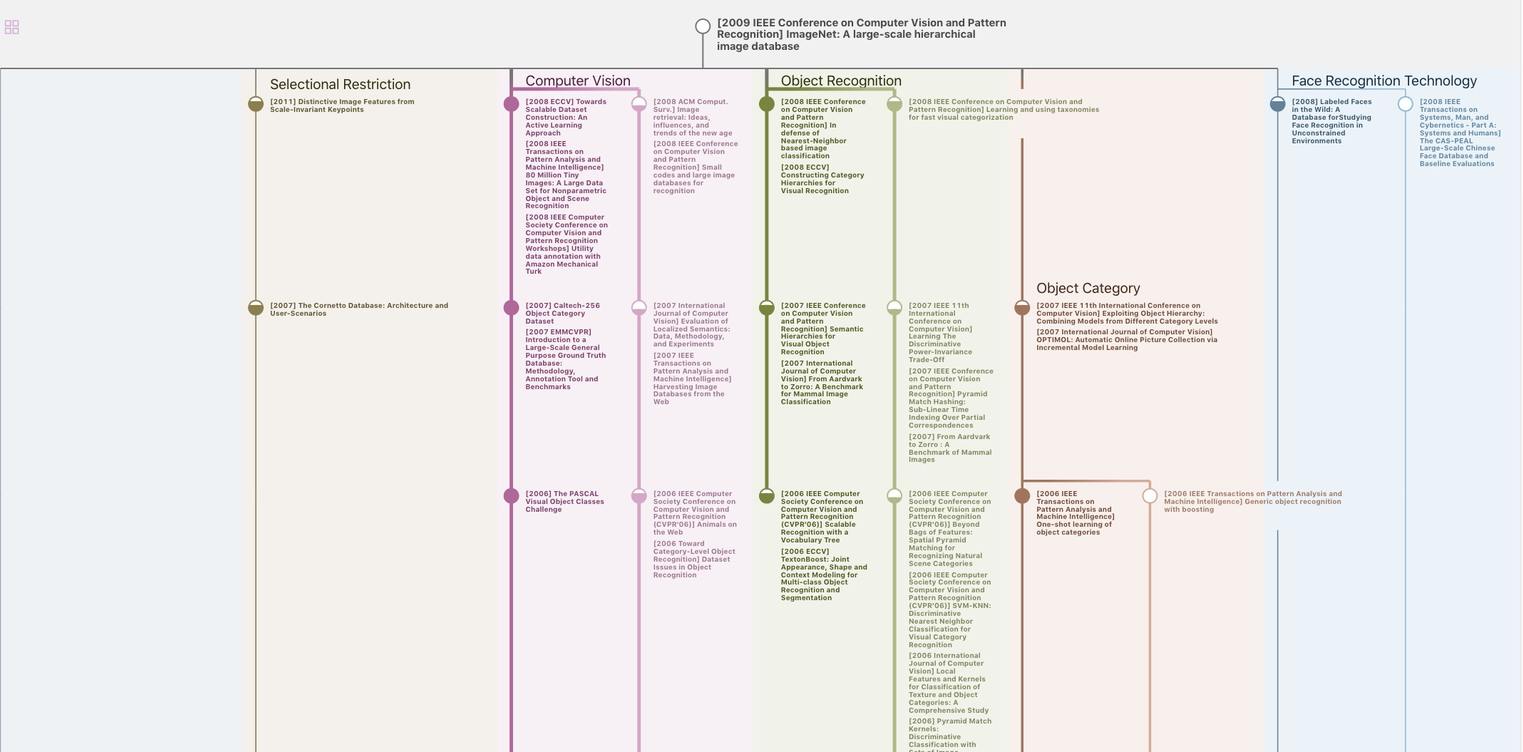
生成溯源树,研究论文发展脉络
Chat Paper
正在生成论文摘要