Peer-to-peer energy trading with energy trading consistency in interconnected multi-energy microgrids: A multi-agent deep reinforcement learning approach
INTERNATIONAL JOURNAL OF ELECTRICAL POWER & ENERGY SYSTEMS(2024)
摘要
Multi-energy microgrid technology is an essential for addressing the diversification of energy demand and local consumption of renewable energy sources. Peer-to-peer energy trading has emerged as a promising paradigm for the design of a decentralized trading framework. Therefore, this paper investigated the external peer-to-peer energy trading problem and internal energy conversion problem of interconnected multi-energy microgrids. The concept of energy trading consistency to avoid unreasonable energy trading behavior is first proposed and an off-design performance model of the energy conversion device is considered to more accurately reflect the operating status of the device. The complex decision-making problem with significantly large high-dimensional data is formulated as a partially observable Markov decision process and solved using the proposed multi-agent deep reinforcement learning approach combining the centralized training decentralized execution framework and soft actor-critic algorithm. Finally, the effectiveness of the proposed method was verified through a case simulation. The simulation results showed that the proposed method can reduce the total cost compared with the rule-based method.
更多查看译文
关键词
Multi-energy microgrids,P2P energy trading,Energy trading consistency,Multi-agent deep reinforcement learning
AI 理解论文
溯源树
样例
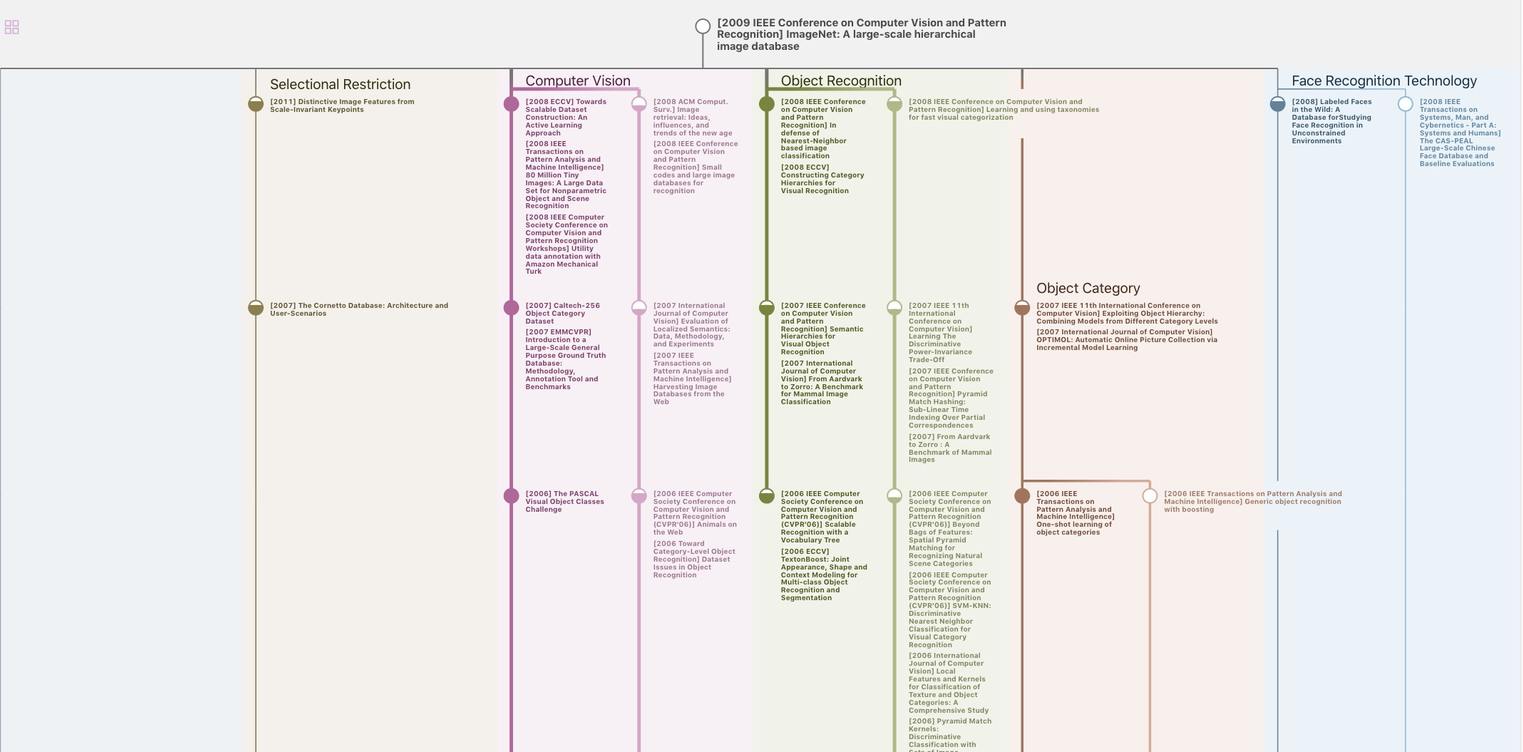
生成溯源树,研究论文发展脉络
Chat Paper
正在生成论文摘要