FDML: Feature Disentangling and Multi-view Learning for Face Forgery Detection
NEUROCOMPUTING(2024)
关键词
Facial manipulation,Multi-view learning,Feature disentangling,Forgery-relevant features,Source-relevant features
AI 理解论文
溯源树
样例
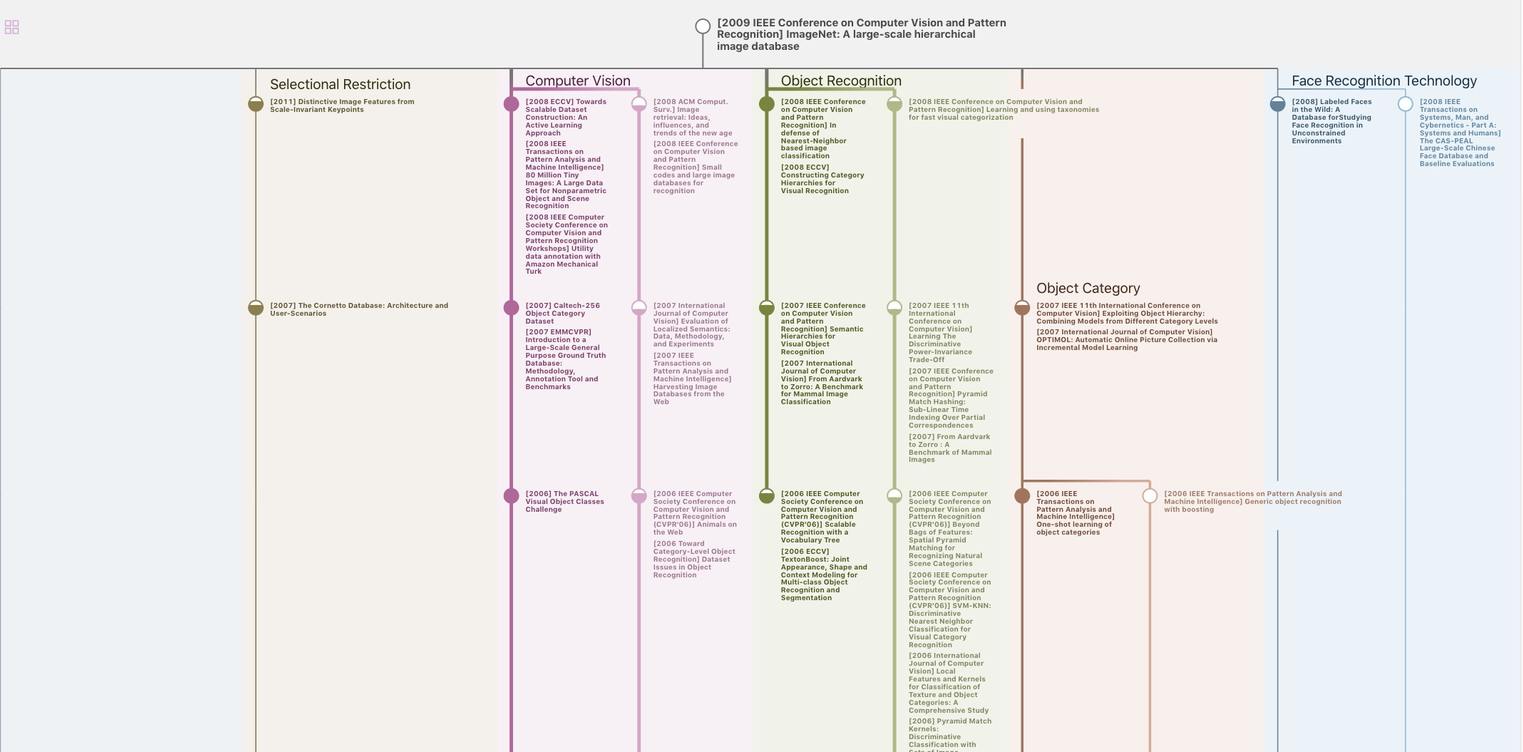
生成溯源树,研究论文发展脉络
Chat Paper
正在生成论文摘要