A Dual-Branch Interactive Fusion Network to Remove Artifacts From Single-Channel EEG
IEEE TRANSACTIONS ON INSTRUMENTATION AND MEASUREMENT(2024)
Abstract
Electroencephalogram (EEG) obtained from the scalp is often polluted with various artifacts. Deep learning (DL) has been preliminarily explored in EEG denoising and proved to be more promising than traditional methods. However, existing DL methods focus on recovering the clean EEG, while ignoring the recovery of artifacts present in contaminated signals. This limits their ability to eliminate artifacts. In this article, we propose a dual-branch interactive fusion neural network, called EEGIFNet, to remove artifacts from the single-channel EEG. In EEGIFNet, the two branches are employed to recover the clean EEG and residual artifacts, respectively. Convolution layers and bidirectional gated recurrent unit (BiGRU) are cascaded in each branch to capture local and global features. We also introduce an interaction module to extract residual components from opposite branches, which benefits both branches from each other. In addition, a fusion module is designed to adaptively fuse the outputs of the two branches and finally reconstruct the clean EEG. We evaluate the proposed method on publicly available datasets including the electrooculogram (EOG), electromyography (EMG), electrocardiogram (ECG), power line interference (PLI), and motion artifacts. Extensive experiments indicate that EEGIFNet outperforms the state-of-the-art methods on diverse artifacts and signal-to-noise (SNR) levels.
MoreTranslated text
Key words
Electroencephalography,Feature extraction,Electrooculography,Electromyography,Convolution,Noise reduction,Electrocardiography,Artifact removal,dual-branch,electroencephalogram (EEG),fusion,interaction
AI Read Science
Must-Reading Tree
Example
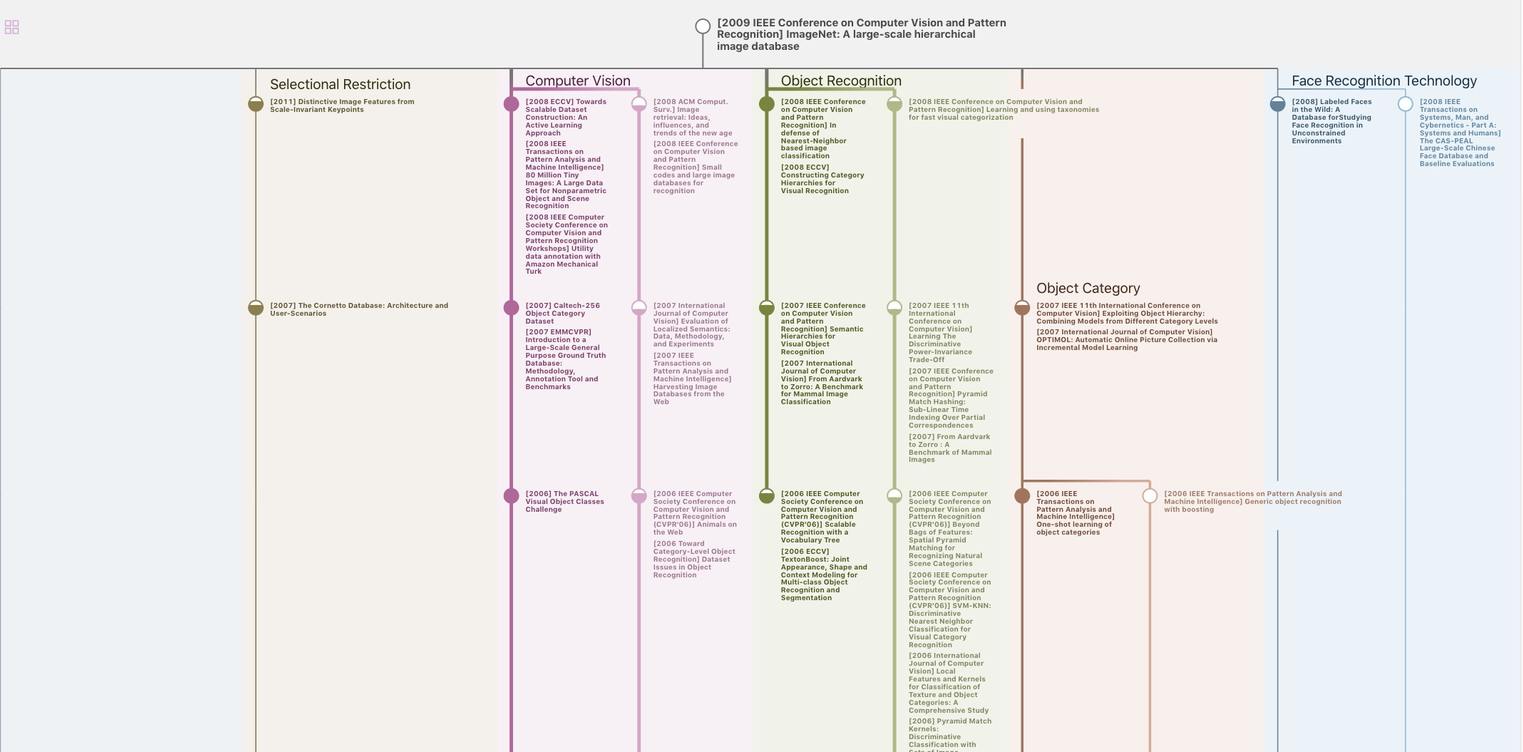
Generate MRT to find the research sequence of this paper
Chat Paper
Summary is being generated by the instructions you defined