Multimodal Data-Driven Reinforcement Learning for Operational Decision-Making in Industrial Processes
IEEE-CAA JOURNAL OF AUTOMATICA SINICA(2024)
摘要
Dear Editor, This letter proposes a multimodal data-driven reinforcement learning-based method for operational decision-making in industrial processes. Due to the frequent fluctuations of feedstock properties and operating conditions in the industrial processes, existing data-driven methods cannot effectively adjust the operational variables. In addition, multimodal data such as images, audio, and sensor data are still not fully used in industrial processes. To overcome the impact of feedstock condition fluctuations and effectively utilize operational conditions based on the multimodal data, a new method named feed-stock-guided multimodal actor-critic (FGM-AC) is proposed. This letter incorporates the feedstock properties and multimodal data into the state space to guide the decision-making process based on a reinforcement learning (RL) framework to achieve a comprehensive human perception. The effectiveness of the proposed method is verified via extensive experiments conducted on actual industrial data. The results reinforce its potential to provide accurate and dependable strategies for decision-making.
更多查看译文
AI 理解论文
溯源树
样例
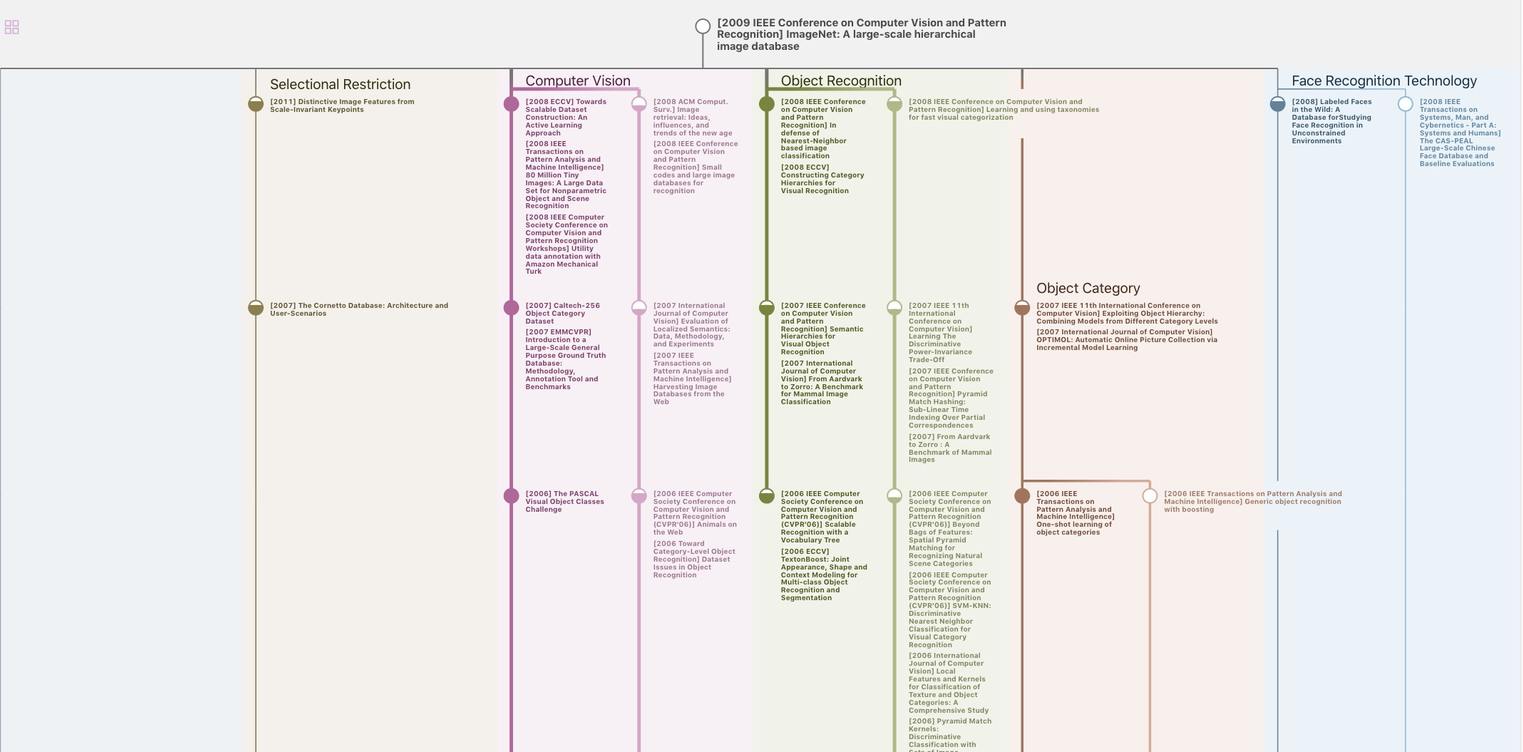
生成溯源树,研究论文发展脉络
Chat Paper
正在生成论文摘要