Dual Class Token Vision Transformer for Direction of Arrival Estimation in Low SNR
IEEE SIGNAL PROCESSING LETTERS(2024)
摘要
In this letter, we propose a deep learning-based method for the direction of arrival (DOA) estimation in the low signal-to-noise ratio (SNR) scenario. Specifically, the DOA estimation is modeled as a multi-label classification task, and a novel dual class token Vision Transformer (DCT-ViT) is designed to fit it. Different from the classical ViT architecture with a single class token, the DCT-ViT includes two class tokens which are located at the beginning and end of the latent vector sequence, respectively. This architecture enables enhanced information mining and feature extraction from the array signal data in order to improve the accuracy of DOA estimation. Furthermore, a single DCT-ViT model can accommodate different source numbers by leveraging a training dataset with different numbers of sources. Simulation results illustrate that our proposed method outperforms existing methods in the low SNR scenario, including classical model-based and other deep learning-based methods.
更多查看译文
关键词
Direction of arrival (DOA) estimation,Vision Transformer (ViT),low signal-to-noise ratio (SNR),deep learning
AI 理解论文
溯源树
样例
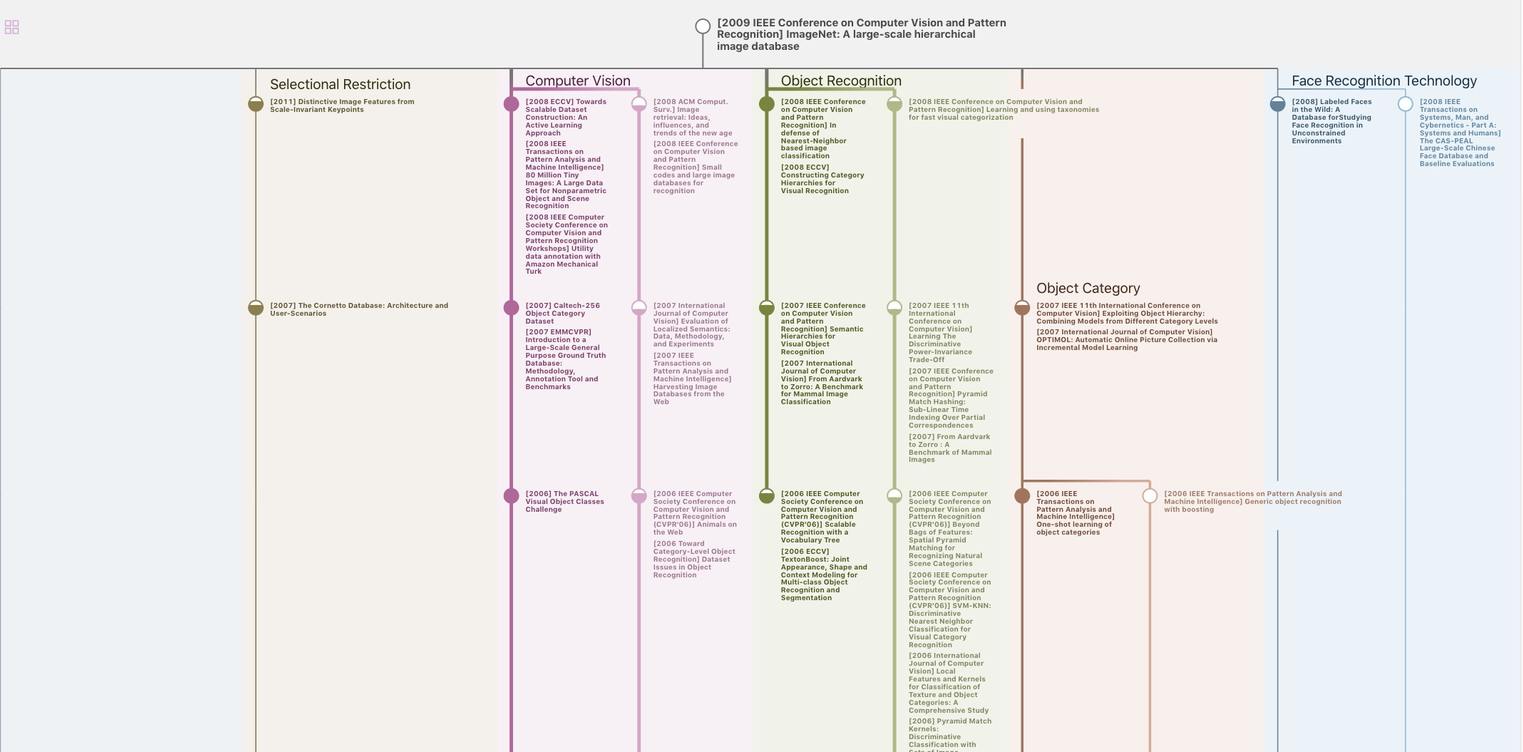
生成溯源树,研究论文发展脉络
Chat Paper
正在生成论文摘要