Medical object detector jointly driven by knowledge and data
NEURAL NETWORKS(2024)
Abstract
Most of the existing object detection algorithms are trained on medical datasets and then used for prediction. When the features of an object are not obvious in an image, these models are prone to mislocalize and misclassify it. In this paper, we propose a medical Object Detection algorithm jointly driven by Knowledge and Data (ODKD). It enables medical semantic knowledge provided by specialized physicians to be effective and helpful when traditional models have difficulty in correctly detecting objects relying on features alone. Our model consists of a base object detector together with a fusion module: the base object detector is trained based on medical datasets to obtain data-driven results; then we use a graph to represent external semantic knowledge and map the data-driven results to the nodes embedding of this graph structure. In the fusion module, a graph convolution network is used to fuse the data-driven results with the external semantic knowledge to output category adjustment coefficients. Finally, the adjustment coefficients are used to adjust the data-driven results to obtain results jointly driven by knowledge and data. Experiments show that professional medical semantic knowledge can effectively correct the erroneous results of the base detector, and the effect of our model outperforms Faster Rcnn, YOLOv5, YOLOv7, etc. on three medical datasets, Camus, Synapse, and AMOS.
MoreTranslated text
Key words
Object detection,GCN,Semantic relations,Jointly driven
AI Read Science
Must-Reading Tree
Example
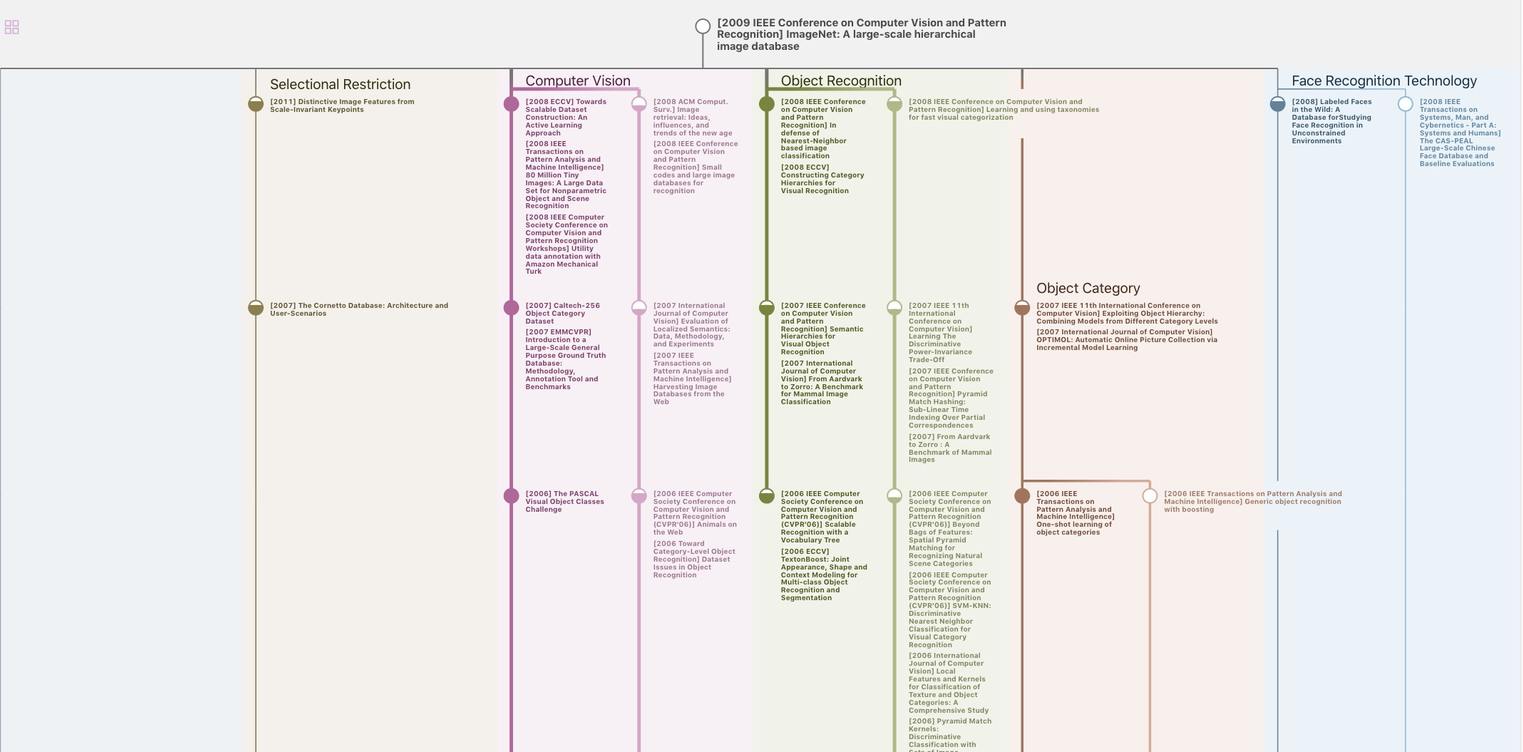
Generate MRT to find the research sequence of this paper
Chat Paper
Summary is being generated by the instructions you defined