Deep learning prediction of esophageal squamous cell carcinoma invasion depth from arterial phase enhanced CT images: a binary classification approach
BMC Medical Informatics and Decision Making(2024)
Abstract
Precise prediction of esophageal squamous cell carcinoma (ESCC) invasion depth is crucial not only for optimizing treatment plans but also for reducing the need for invasive procedures, consequently lowering complications and costs. Despite this, current techniques, which can be invasive and costly, struggle with achieving the necessary precision, highlighting a pressing need for more effective, non-invasive alternatives. We developed ResoLSTM-Depth, a deep learning model to distinguish ESCC stages T1-T2 from T3-T4. It integrates ResNet-18 and Long Short-Term Memory (LSTM) networks, leveraging their strengths in spatial and sequential data processing. This method uses arterial phase CT scans from ESCC patients. The dataset was meticulously segmented by an experienced radiologist for effective training and validation. Upon performing five-fold cross-validation, the ResoLSTM-Depth model exhibited commendable performance with an accuracy of 0.857, an AUC of 0.901, a sensitivity of 0.884, and a specificity of 0.828. These results were superior to the ResNet-18 model alone, where the average accuracy is 0.824 and the AUC is 0.879. Attention maps further highlighted influential features for depth prediction, enhancing model interpretability. ResoLSTM-Depth is a promising tool for ESCC invasion depth prediction. It offers potential for improvement in the staging and therapeutic planning of ESCC.
MoreTranslated text
Key words
Esophageal squamous cell carcinoma,Deep learning,Computed tomography
AI Read Science
Must-Reading Tree
Example
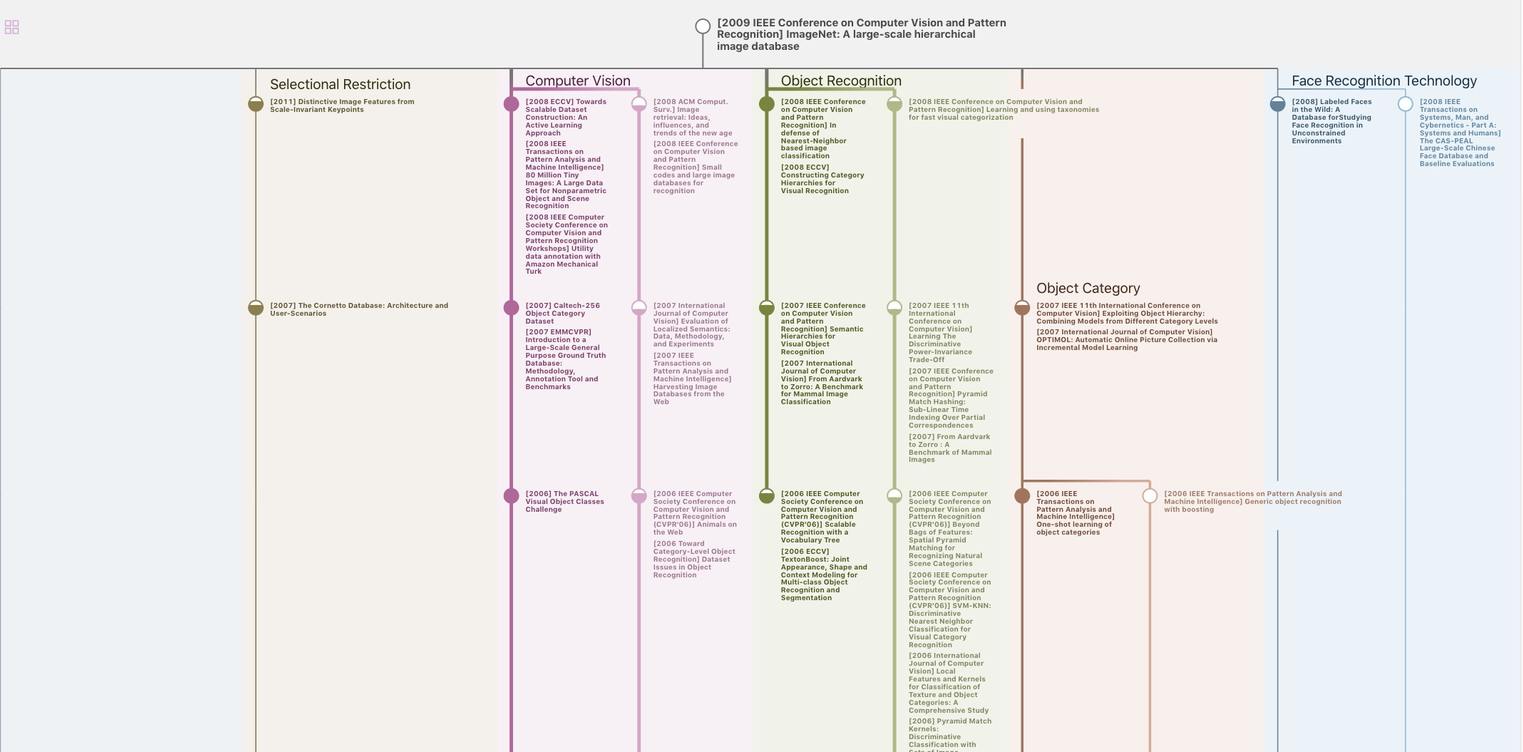
Generate MRT to find the research sequence of this paper
Chat Paper
Summary is being generated by the instructions you defined