Comparison of neural networks techniques to predict subsurface parameters based on seismic inversion: a machine learning approach
Earth Science Informatics(2024)
Abstract
Seismic inversion, complemented by machine learning algorithms, significantly improves the accuracy and efficiency of subsurface parameter estimation from seismic data. In this comprehensive study, a comparative analysis of machine learning techniques is conducted to predict subsurface parameters within the inter-well region. The objective involves employing three separate machine learning algorithms namely Probabilistic Neural Network (PNN), multilayer feedforward neural network (MLFNN), and Radial Basis Function Neural Network (RBFNN). The study commences by generating synthetic data, which is then subjected to machine learning techniques for inversion into subsurface parameters. The results unveil exceptionally detailed subsurface information across various methods. Subsequently, these algorithms are applied to real data from the Blackfoot field in Canada to predict porosity, density, and P-wave velocity within the inter-well region. The inverted results exhibit a remarkable alignment with well-log parameters, achieving an average correlation of 0.75, 0.77, and 0.86 for MLFNN, RBFNN, and PNN algorithms, respectively. The inverted volumes portray a consistent pattern of impedance variations spanning 7000–18000 m/s*g/cc, porosity ranging from 5 to 20%, and density within the range of 1.9–2.9 g/cc across the region. Importantly, all these methods yield mutually corroborative results, with PNN displaying a slight edge in estimation precision. Additionally, the interpretation of the inverted findings highlights anomalous zones characterized by low impedance, low density, and high porosity, seamlessly aligning with well-log data and being identified as sand channel. This study underscores the potential for seismic inversion, driven by machine learning techniques, to swiftly and cost-effectively determine critical subsurface parameters like acoustic impedance and porosity.
MoreTranslated text
Key words
Seismic inversion,Machine learning,Neural network,Sand channel,Activation functions
AI Read Science
Must-Reading Tree
Example
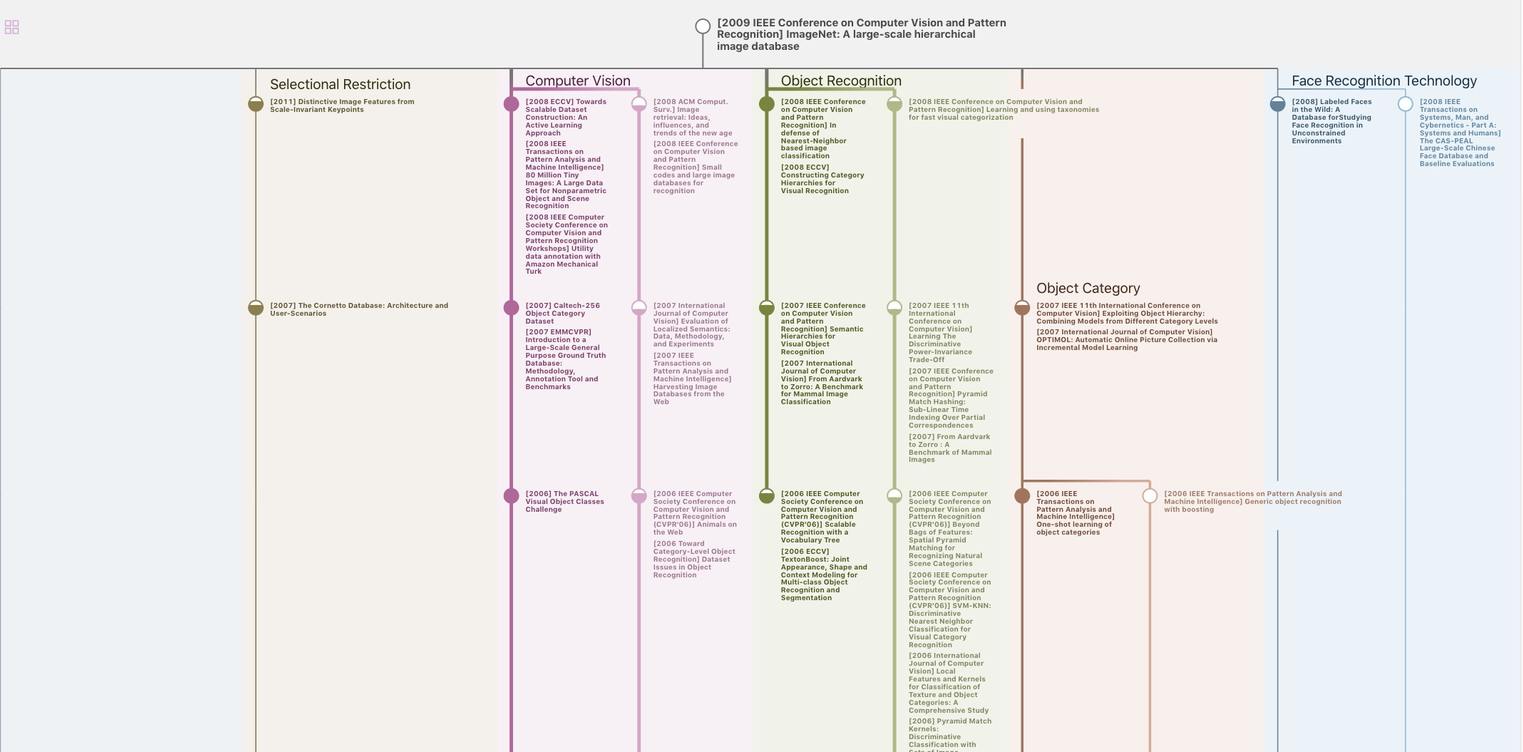
Generate MRT to find the research sequence of this paper
Chat Paper
Summary is being generated by the instructions you defined