Enhancing Ki-67 Prediction in Breast Cancer: Integrating Intratumoral and Peritumoral Radiomics From Automated Breast Ultrasound via Machine Learning
Academic Radiology(2024)
Abstract
RATIONALE AND OBJECTIVES:Traditional Ki-67 evaluation in breast cancer (BC) via core needle biopsy is limited by repeatability and heterogeneity. The automated breast ultrasound system (ABUS) offers reproducibility but is constrained to morphological and echoic assessments. Radiomics and machine learning (ML) offer solutions, but their integration for improving Ki-67 predictive accuracy in BC remains unexplored. This study aims to enhance ABUS by integrating ML-assisted radiomics for Ki-67 prediction in BC, with a focus on both intratumoral and peritumoral regions.
MATERIALS AND METHODS:A retrospective analysis was conducted on 936 BC patients, split into training (n = 655) and testing (n = 281) cohorts. Radiomics features were extracted from intra- and peritumoral regions via ABUS. Feature selection involved Z-score normalization, intraclass correlation, Wilcoxon rank sum tests, minimum redundancy maximum relevance, and least absolute shrinkage and selection operator logistic regression. ML classifiers were trained and optimized for enhanced predictive accuracy. The interpretability of the optimized model was further augmented by employing Shapley additive explanations (SHAP).
RESULTS:Of the 2632 radiomics features in each patient, 15 were significantly associated with Ki-67 levels. The support vector machine (SVM) was identified as the optimal classifier, with area under the receiver operating characteristic curve values of 0.868 (training) and 0.822 (testing). SHAP analysis indicated that five peritumoral and two intratumoral features, along with age and lymph node status, were key determinants in the predictive model.
CONCLUSION:Integrating ML with ABUS-based radiomics effectively enhances Ki-67 prediction in BC, demonstrating the SVM model's strong performance with both radiomics and clinical factors.
MoreTranslated text
Key words
Breast cancer,Ki-67 expression,Automated breast ultrasound system,Radiomics,Machine learning
AI Read Science
Must-Reading Tree
Example
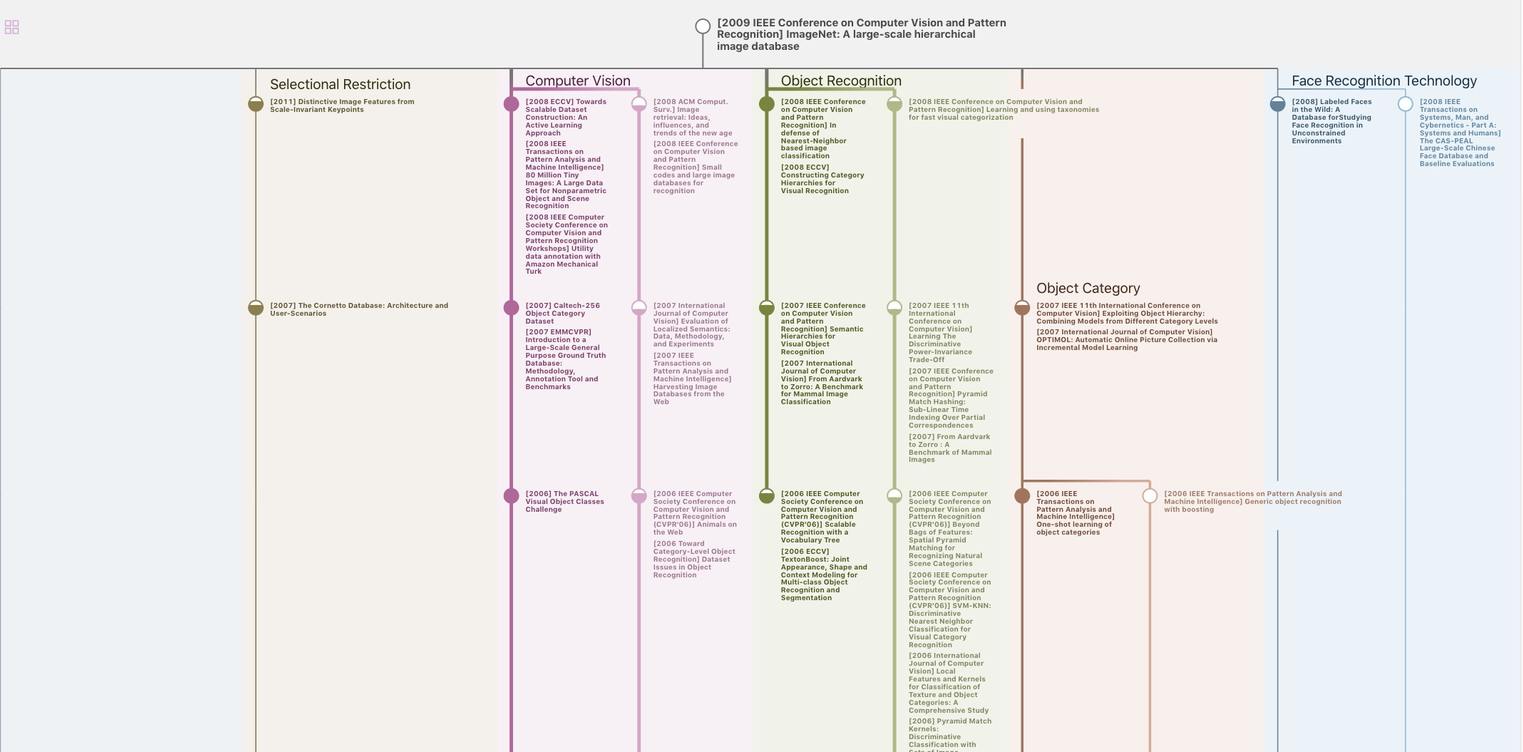
Generate MRT to find the research sequence of this paper
Chat Paper
Summary is being generated by the instructions you defined