RGR-Net: Refined Graph Reasoning Network for multi-height hotspot defect detection in photovoltaic farms
EXPERT SYSTEMS WITH APPLICATIONS(2024)
摘要
Unmanned aerial vehicle (UAV) detection of hotspot defects at multi-height is crucial for the reliable operation of photovoltaic (PV) farms. However, there are two major challenges in PV power plant data: defect information loss and noise information interference. In this paper, we design a Refined Graph Reasoning Network (RGR-Net) mainly composed of the backbone network, Cross-embedding Refined Graph Reasoning (CRGR) module, and prediction head. The proposed RGR-Net learns rich discriminative feature representations of hotspots by fully using the complementary information between the network layers and establishing global long-range dependencies. Specifically, CRGR learns to project input features into a global vector of a channel and performs inference on the representation of each defect with the global vector. CRGR utilizes two different transformation matrices to convert nodes and their connected nodes into a new feature space, thereby refining feature expression. Furthermore, we introduce the multi-height hotspot dataset (MHHS), marking the first comprehensive database tailored for defect detection across various flying altitudes, aiming to propel advancements in ensuring the safety and efficiency of PV farms. It should be noted that the current study focuses on data collected under favorable weather conditions, and further research is needed to assess the robustness of our model in extreme weather scenarios such as fog, heavy rain, or snow. Experimental results demonstrate that RGR-Net achieves an AP of 84.0% on the MHHS on average, surpassing the current state-of-the-art detectors.1
更多查看译文
关键词
Multi-height PV monitoring,Cross-embedding projection,Refined graph reasoning,Long-range dependency modeling,Intelligent inspection system
AI 理解论文
溯源树
样例
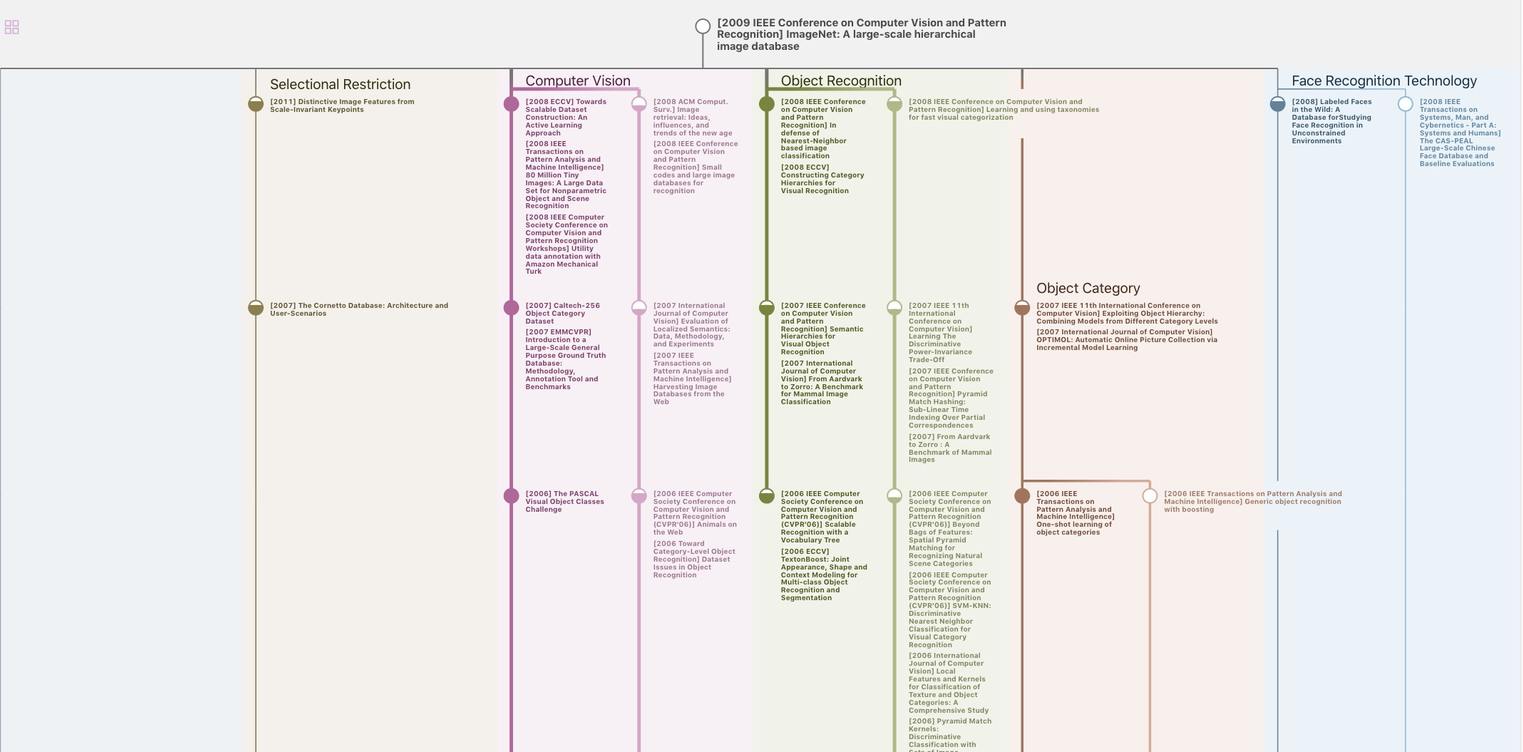
生成溯源树,研究论文发展脉络
Chat Paper
正在生成论文摘要