Humidification potential optimization of various membranes for proton exchange membrane fuel cell: Experiments and deep learning assisted metaheuristics
ALEXANDRIA ENGINEERING JOURNAL(2024)
摘要
This study addresses the durability and performance challenges in Proton Exchange Membrane Fuel Cell (PEMFC) technology, primarily focusing on optimal humidity management. Acknowledging that, beyond technical aspects, the broader commercialization of PEMFCs is critically influenced by factors such as the cost and availability of hydrogen, this research aims to provide a comprehensive solution to enhance PEMFC applicability. Utilizing Nafion (NR -212), reverse osmosis (RO), and pervaporation (PV) membranes, the study optimizes five key performance metrics: pressure drop (Delta P), dew point approach temperature (DPAT), water recovery ratio (WRR), Water Flux (J), and coefficient of performance (COP). These optimizations are conducted considering variables like temperature, humidity, flowrate, and membrane material. A deep neural network (DNN) model, incorporating Bayesian surrogacy with Gaussian process, gradient boost regression trees, and random forest, is developed using experimental data. With a correlation coefficient of 0.986, the model demonstrates high accuracy in predicting performance metrics, subsequently guiding genetic algorithms for effective PEMFC humidity control. The results show significant improvements in all metrics, with optimal values achieved for NR -212, RO, and PV membranes. This study thus presents a novel, practical deep learning approach, considering both technological advancements and external economic factors, for enhancing PEMFC operations.
更多查看译文
关键词
Humidifier technology,Proton exchange membrane fuel cells,Artificial intelligence in energy systems,Optimization with genetic algorithms,Deep learning applications in renewable energy,Energy efficiency
AI 理解论文
溯源树
样例
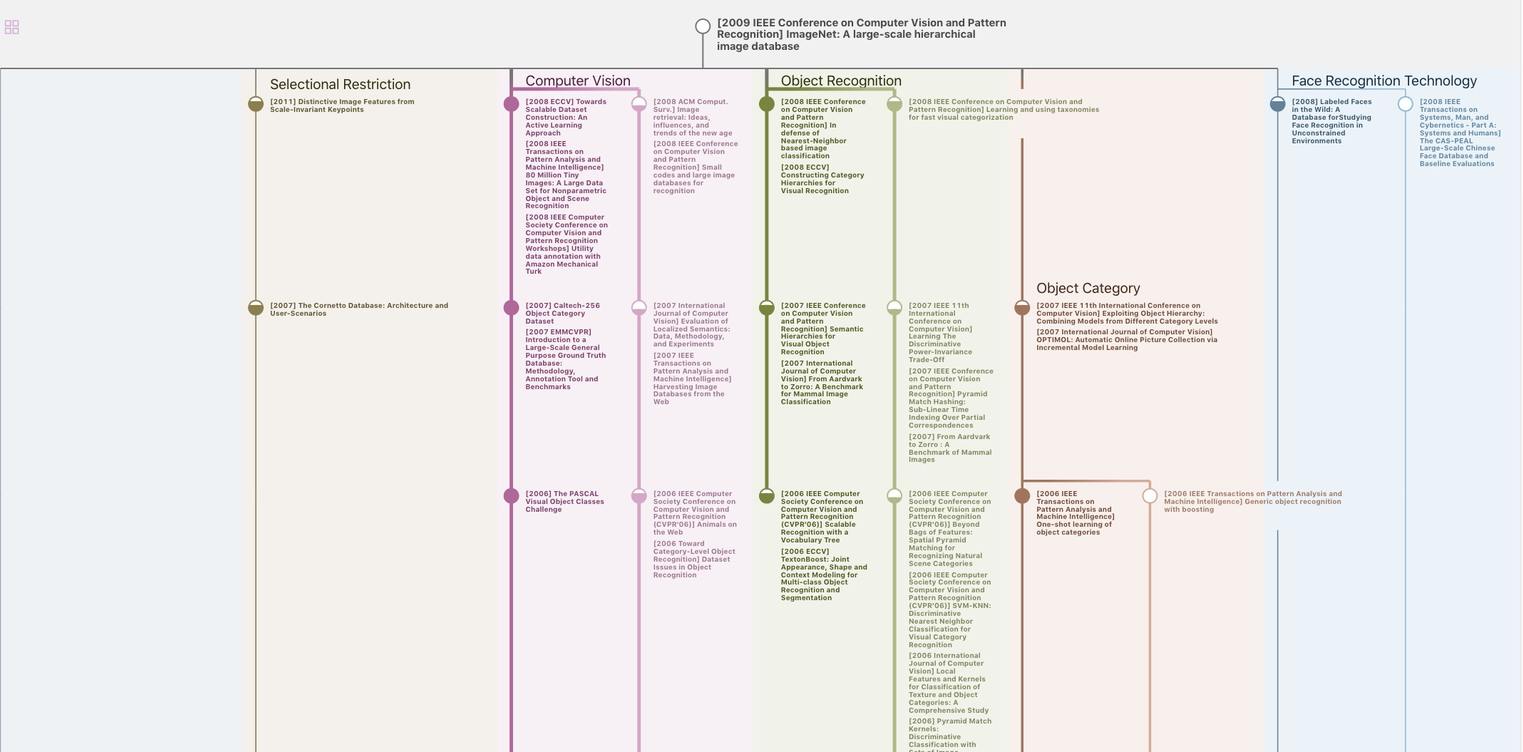
生成溯源树,研究论文发展脉络
Chat Paper
正在生成论文摘要