Examining the role of passive design indicators in energy burden reduction: Insights from a machine learning and deep learning approach
BUILDING AND ENVIRONMENT(2024)
摘要
Passive design characteristics (PDC) play a pivotal role in reducing the energy burden on households without imposing additional financial constraints on project stakeholders. However, the scarcity of PDC data has posed a challenge in previous studies when assessing their energy-saving impact. To tackle this issue, this research introduces an innovative approach that combines deep learning-powered computer vision with machine learning techniques to examine the relationship between PDC and energy burden in residential buildings. In this study, we employ a convolutional neural network computer vision model to identify and measure key indicators, including window-to-wall ratio (WWR), external shading, and operable window types, using Google Street View images within the Chicago metropolitan area as our case study. Subsequently, we utilize the derived passive design features in conjunction with demographic characteristics to train and compare various machine learning methods. These methods encompass Decision Tree Regression, Random Forest Regression, and Support Vector Regression, culminating in the development of a comprehensive model for energy burden prediction. Our framework achieves a 74.2 % accuracy in forecasting the average energy burden. These results yield invaluable insights for policymakers and urban planners, paving the way toward the realization of smart and sustainable cities.
更多查看译文
关键词
Average energy burden,Machine learning,Sustainable cities,Convolutional neural network,Computer vision
AI 理解论文
溯源树
样例
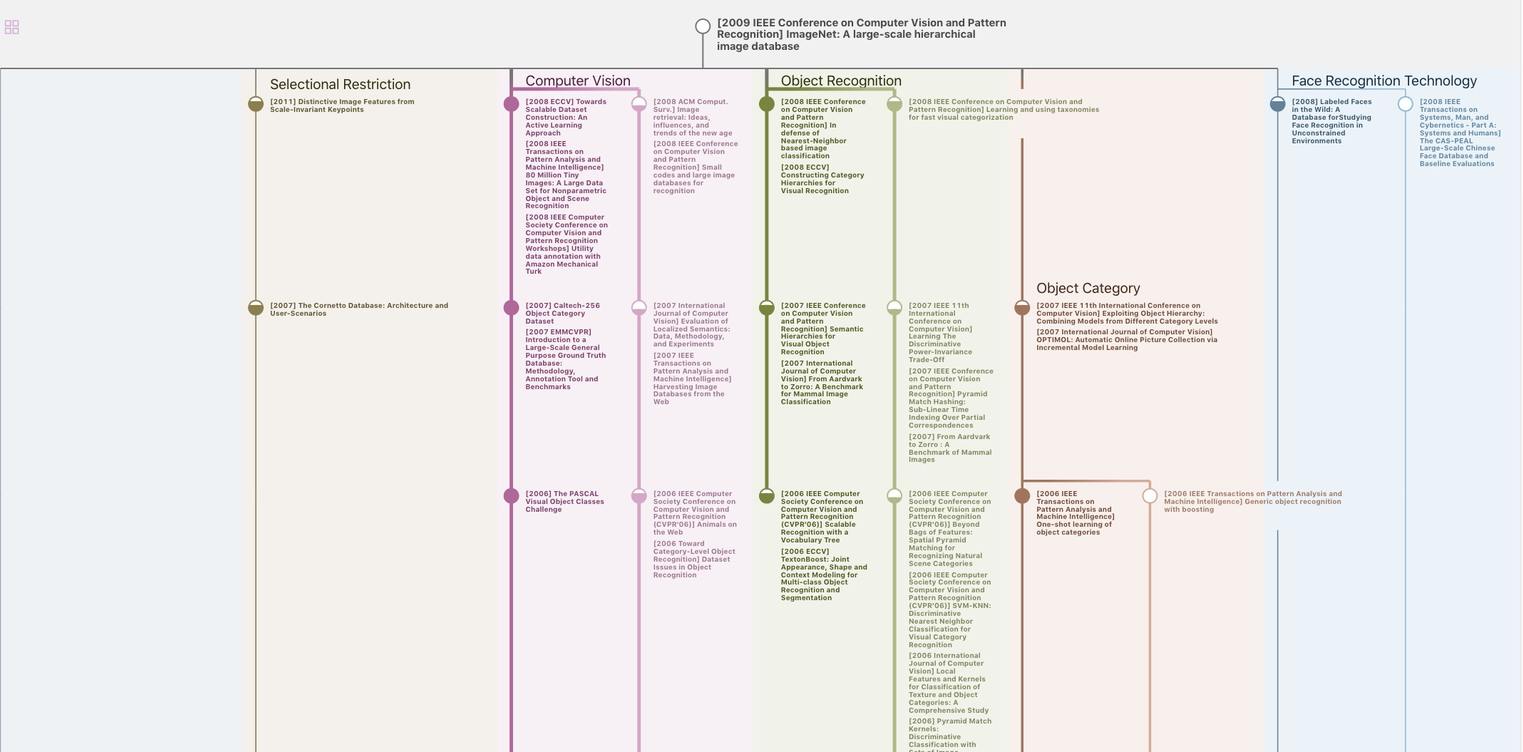
生成溯源树,研究论文发展脉络
Chat Paper
正在生成论文摘要