Explainable Deep Learning to Classify Royal Navy Ships
IEEE ACCESS(2024)
摘要
We research how deep learning convolutional neural networks can be used to automatically classify the unique data set of black-and-white naval ships images from the Wright and Logan photographic collection held by the National Museum of the Royal Navy. We contrast various types of deep learning methods: pretrained models such as ConvNeXt, ResNet and EfficientNet, and ConvMixer. We also thoroughly investigate the impact of data preprocessing and externally obtained images on model performance. Finally, we research how the models estimated can be made transparent using visually appealing interpretability techniques such as Grad-CAM. We find that ConvNeXt has the best performance for our data set achieving an accuracy of 79.62% for 0-notch classification and an impressive 94.86% for 1-notch classification. The results indicate the importance of appropriate image preprocessing. Image segmentation combined with soft augmentation significantly contributes to model performance. We consider this research to be original in several aspects. Notably, it distinguishes itself through the uniqueness of the acquired dataset. Additionally, its distinctiveness extends to the analytical modeling pipeline, which encompasses a comprehensive range of modeling steps, including data preprocessing (incorporating external data, image segmentation, and image augmentation) and the use of deep learning techniques such as ConvNeXt, ResNet, EfficientNet, and ConvMixer. Furthermore, the research employs explanatory tools like Grad-CAM to enhance model interpretability and usability. We believe the proposed methodology offers lots of potential for documenting historic image collections.
更多查看译文
关键词
Convolutional neural networks,deep learning,explainability,digitised archives,image classification,royal navy
AI 理解论文
溯源树
样例
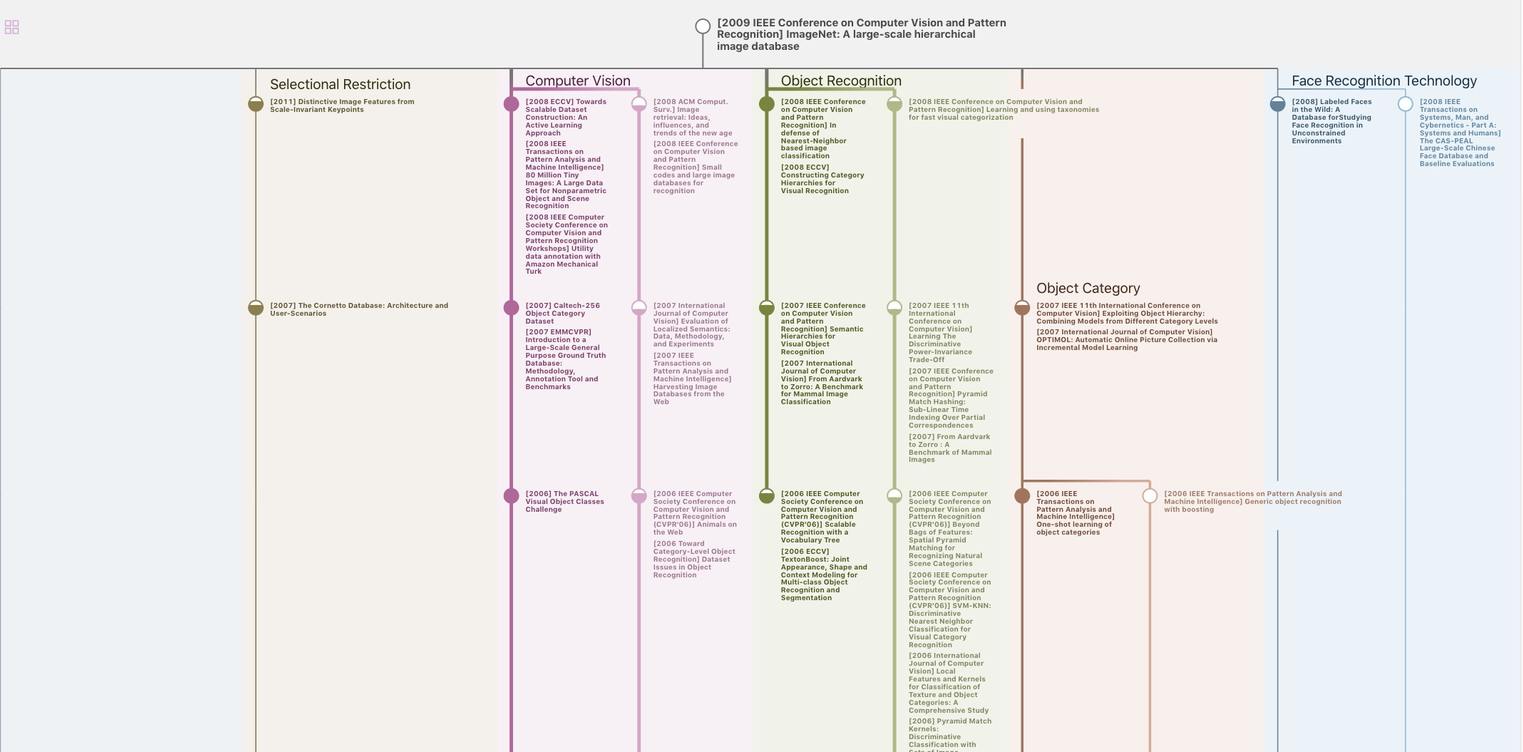
生成溯源树,研究论文发展脉络
Chat Paper
正在生成论文摘要