Genetic-Based Two Granularity Ordering Methods for Multiple Workflow Scheduling
IEEE ACCESS(2024)
摘要
In cloud computing, multiple workflow scheduling is important to optimize resource allocation and utilization for concurrent execution of diverse workflows across different applications. While previous research has focused on clustering-based resource allocation to reduce communication overheads by grouping tasks, it often overlooks the significance of task execution ordering, limiting overall performance optimization. To address this limitation, we propose two genetic-based approaches, considering task and cluster-level characteristics, to introduce novel ordering techniques for multi-workflow scheduling under cluster-based resource allocation. By comparing two granularity ordering methods, we offer valuable insights for efficient task management in multi-workflow environments. Our experiments demonstrate that the proposed approaches, especially the task granularity-based ordering method, outperform existing primary clustering methods, particularly for scenarios involving a large number of workflows or highly parallel workflows.
更多查看译文
关键词
Task analysis,Costs,Scheduling,Resource management,Optimization,Clustering algorithms,Quality of service,Cloud computing,multi-workflow,multi-objective optimization,task ordering,cluster ordering
AI 理解论文
溯源树
样例
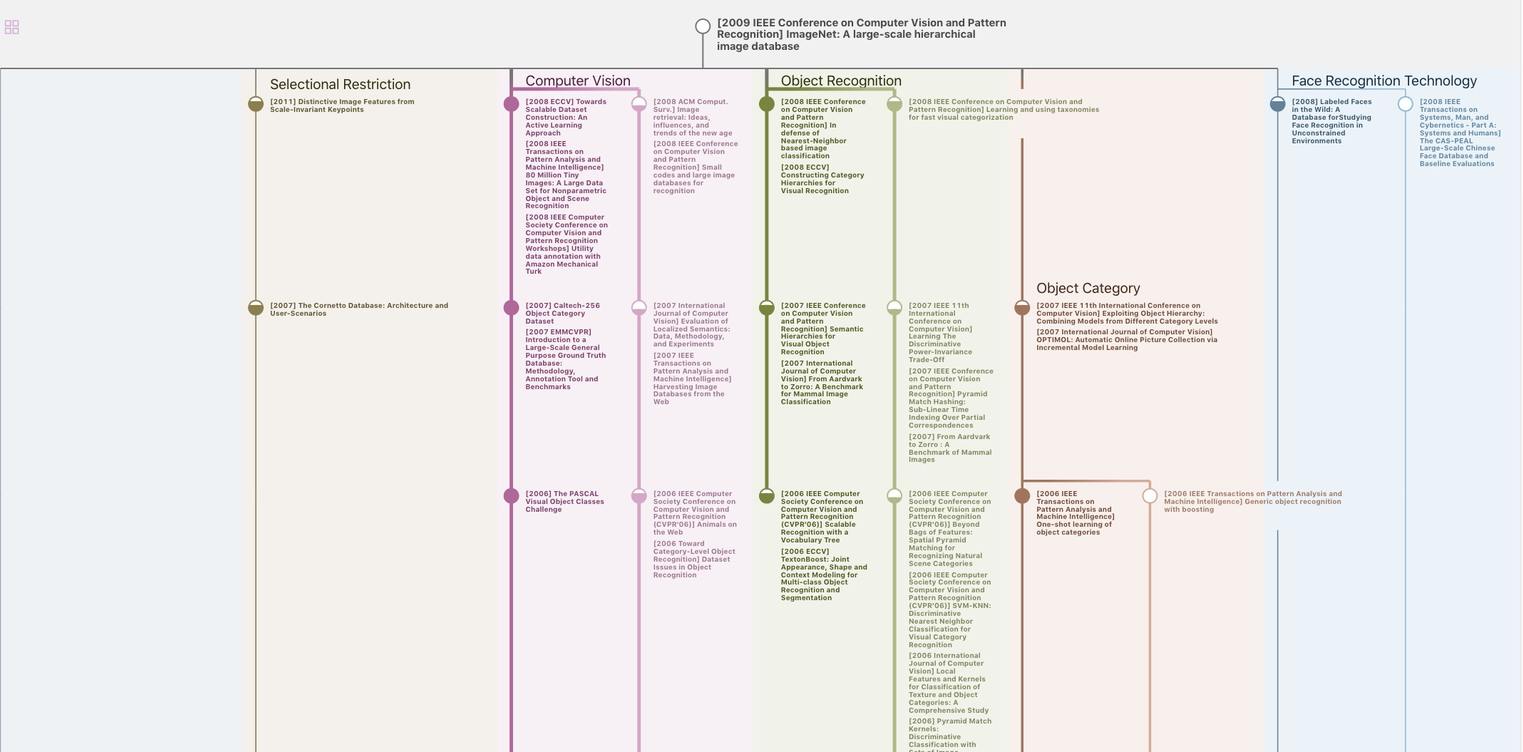
生成溯源树,研究论文发展脉络
Chat Paper
正在生成论文摘要