3D Open-Vocabulary Panoptic Segmentation with 2D-3D Vision-Language Distillation
CoRR(2024)
摘要
3D panoptic segmentation is a challenging perception task, which aims to
predict both semantic and instance annotations for 3D points in a scene.
Although prior 3D panoptic segmentation approaches have achieved great
performance on closed-set benchmarks, generalizing to novel categories remains
an open problem. For unseen object categories, 2D open-vocabulary segmentation
has achieved promising results that solely rely on frozen CLIP backbones and
ensembling multiple classification outputs. However, we find that simply
extending these 2D models to 3D does not achieve good performance due to poor
per-mask classification quality on novel categories. In this paper, we propose
the first method to tackle 3D open-vocabulary panoptic segmentation. Our model
takes advantage of the fusion between learnable LiDAR features and dense frozen
vision CLIP features, using a single classification head to make predictions
for both base and novel classes. To further improve the classification
performance on novel classes and leverage the CLIP model, we propose two novel
loss functions: object-level distillation loss and voxel-level distillation
loss. Our experiments on the nuScenes and SemanticKITTI datasets show that our
method outperforms strong baselines by a large margin.
更多查看译文
AI 理解论文
溯源树
样例
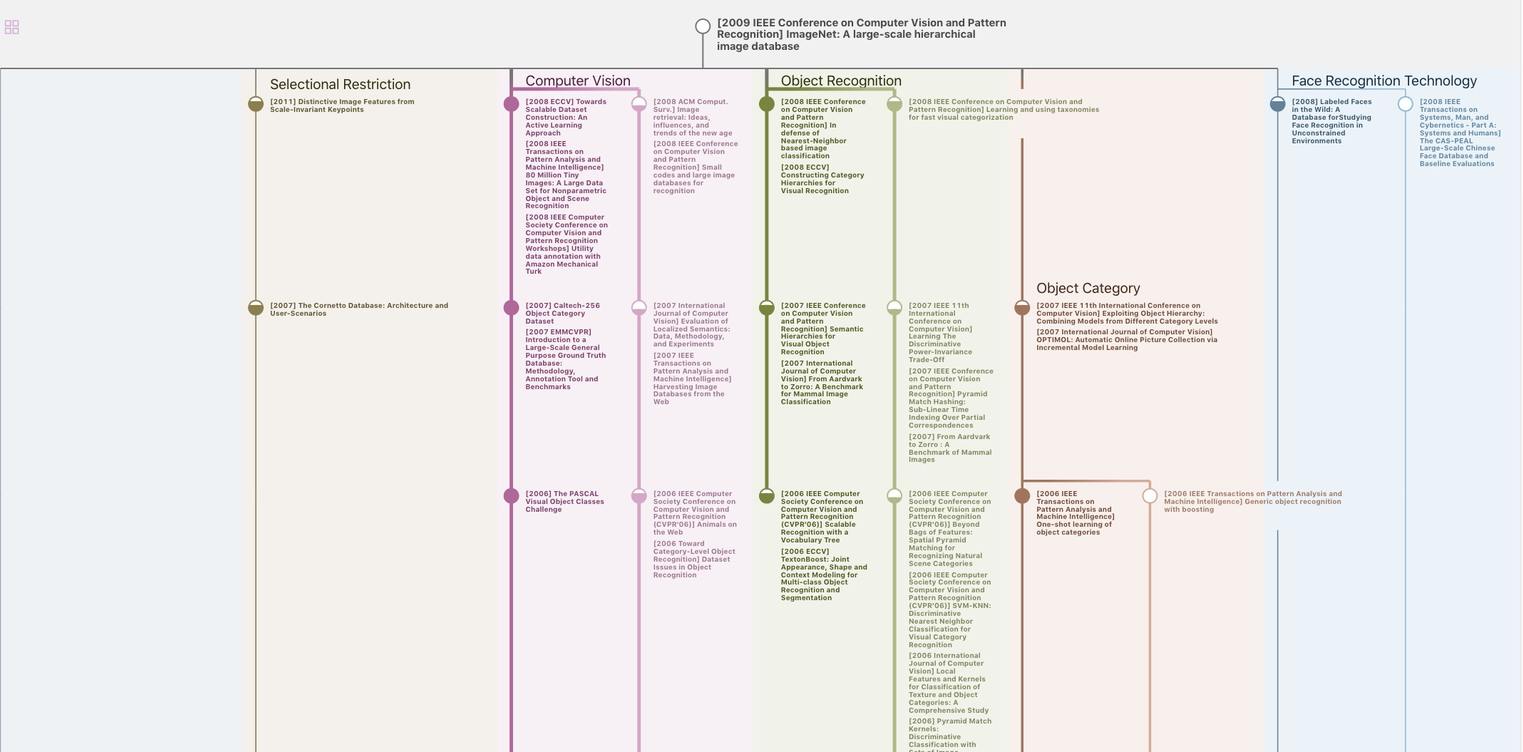
生成溯源树,研究论文发展脉络
Chat Paper
正在生成论文摘要