GUESS: GradUally Enriching SyntheSis for Text-Driven Human Motion Generation.
IEEE transactions on visualization and computer graphics(2024)
摘要
In this paper, we propose a novel cascaded diffusion-based generative framework for text-driven human motion synthesis, which exploits a strategy named GradUally Enriching SyntheSis (GUESS as its abbreviation). The strategy sets up generation objectives by grouping body joints of detailed skeletons in close semantic proximity together and then replacing each of such joint group with a single body-part node. Such an operation recursively abstracts a human pose to coarser and coarser skeletons at multiple granularity levels. Notably, we further integrate GUESS with the proposed dynamic multi-condition fusion mechanism to dynamically balance the cooperative effects of the given textual condition and synthesized coarse motion prompt in different generation stages. Extensive experiments on large-scale datasets verify that GUESS outperforms existing state-of-the-art methods by large margins in terms of accuracy, realisticness, and diversity. Please refer to the supplemental demo video for more visualizations.
更多查看译文
关键词
Human motion synthesis,Latent conditional diffusion,Deep generative model,Coarse-to-fine generation
AI 理解论文
溯源树
样例
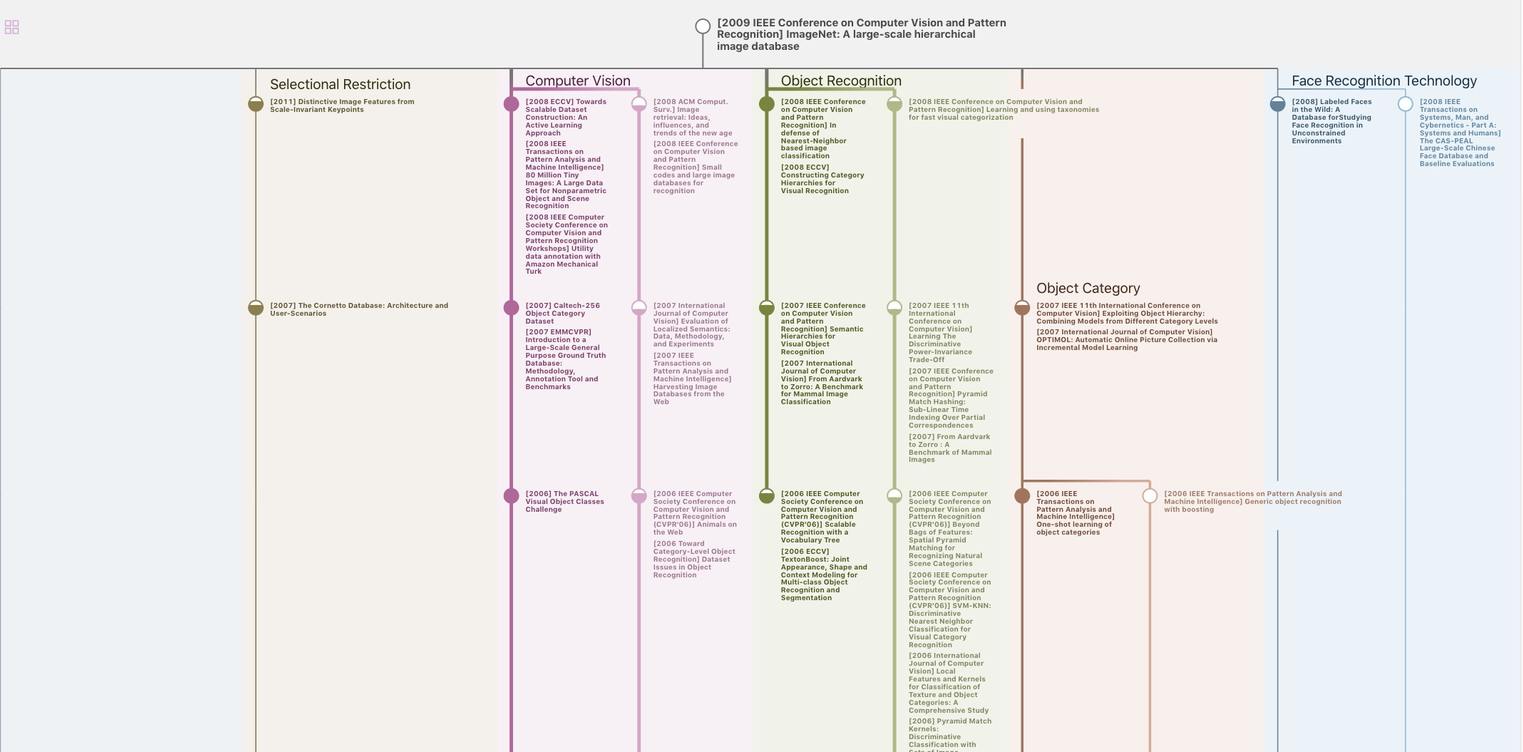
生成溯源树,研究论文发展脉络
Chat Paper
正在生成论文摘要