Multimanifold Bistructured Low Rank Representation of hyperspectral images
INFRARED PHYSICS & TECHNOLOGY(2024)
摘要
Hyperspectral images (HSIs) are obtained by imaging surface materials using a spectral imager. In practical applications, the hyperspectral remote sensing images are inevitably contaminated by noise, leading to errors in image processing results. Gaussian noise, Poisson noise, and stripe noise are common in HSIs. This article presents an HSI denoising model based on the low rank representation of multi -manifold double constructions. Introducing multiple manifolds into the field of hyperspectral image denoising for the first time, comprehensively obtaining complex information on hyperspectral surfaces. We extended the graph -regularized low -rank representation model (GLRR) by using maximum data variance to construct global information. GLRR obtains local information, and the parallel effects of nearest neighbor and maximum variance graphs take into account both global and local consistency in the denoising process. Our model is not only applicable to the case of a single manifold, but also to the case of multiple manifolds, especially in the case of complex ground target images with multiple manifold spectra. Use the LADMAP iterative algorithm to iteratively solve the proposed algorithm. Avoid introducing auxiliary variables to reduce runtime costs and algorithm complexity. Both on simulated and real datasets, the runtime is relatively fast. We experiments on both real and simulated datasets, as well as real datasets, to verify that our method has good denoising performance when Gaussian, Poisson, and stripe noise occur simultaneously.
更多查看译文
关键词
Hyperspectral images,Denoising,Multi-manifold,Low-rank representation
AI 理解论文
溯源树
样例
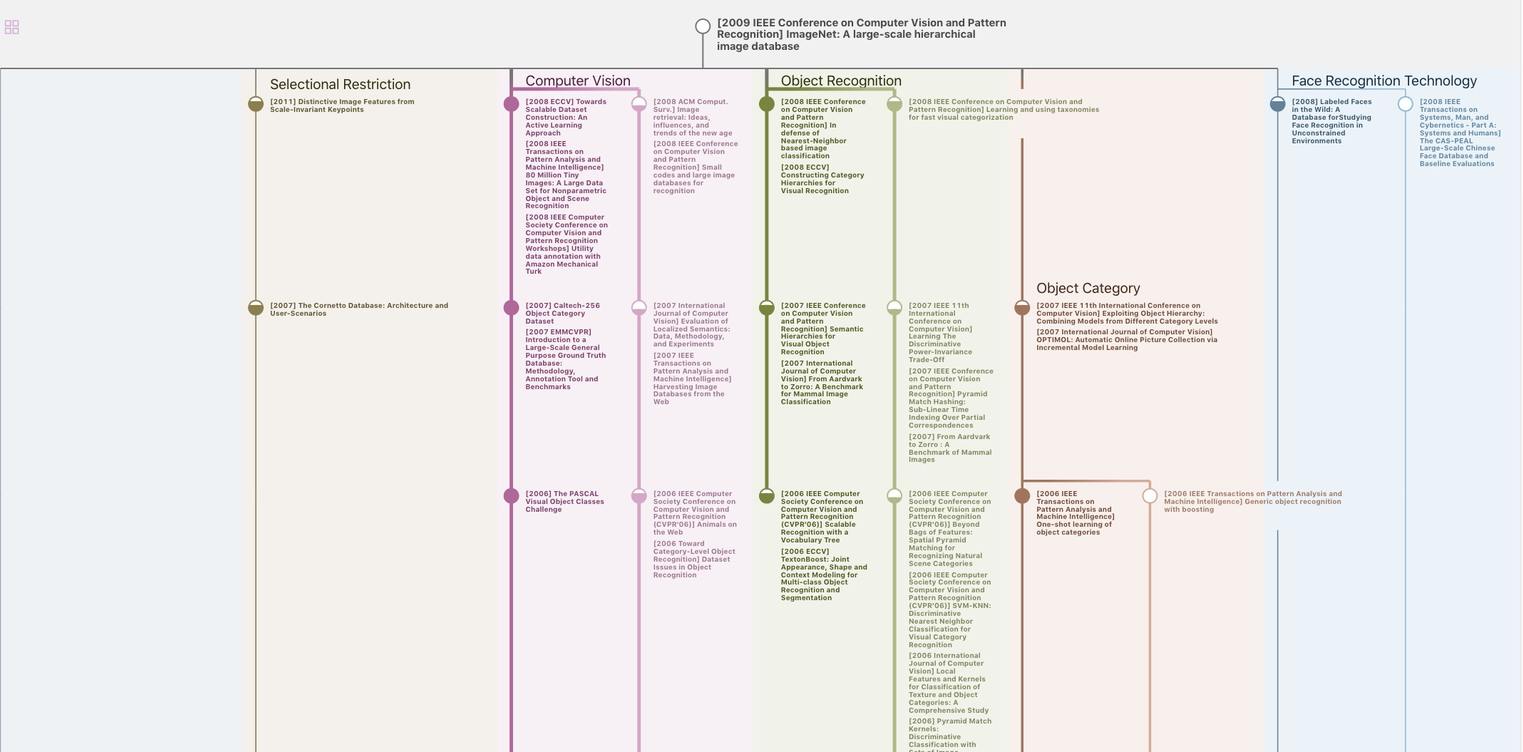
生成溯源树,研究论文发展脉络
Chat Paper
正在生成论文摘要