Time-Domain Feature Extraction and Selection for Detecting Worn Bearings Using Vibration Signals
2023 15th IEEE International Conference on Industry Applications (INDUSCON)(2023)
Abstract
Bearing failure detection in induction motors is one of the most important processes in the industry. When bearing wear reaches a critical level, the machine collapses, and the production process must be stopped. Since bearings are the most susceptible to failure, several works have proposed a solution for their early diagnosis. However, most of these methods employ signal-processing techniques to extract relevant information from the acquired data. This requires a significant computational effort that can make hardware implementation impractical. In this paper, we propose to extract features directly from vibration time series to overcome this problem. The developed method evaluates the relevance of the temporal measures and iteratively removes those that have a lower impact on the classification. Three techniques are compared to estimate the feature importance, including logistic regression (LR), mutual information (MI), and variance inflation factor. Classifications using decision trees, support vector machines, multilayer perceptron, and k-Nearest Neighbors demonstrate that a smaller set of attributes can provide a more accurate classification than the original set of attributes. In addition, this work compares vibration signals acquired by accelerometers and an industrial vibration sensor. The experiments show that only seven temporal attributes from vibration signals are sufficient to achieve a success rate of about 93% in detecting incipient bearing wear, considering different load and voltage unbalance levels.
MoreTranslated text
Key words
Induction motor,bearing fault,machine learning,feature selection
AI Read Science
Must-Reading Tree
Example
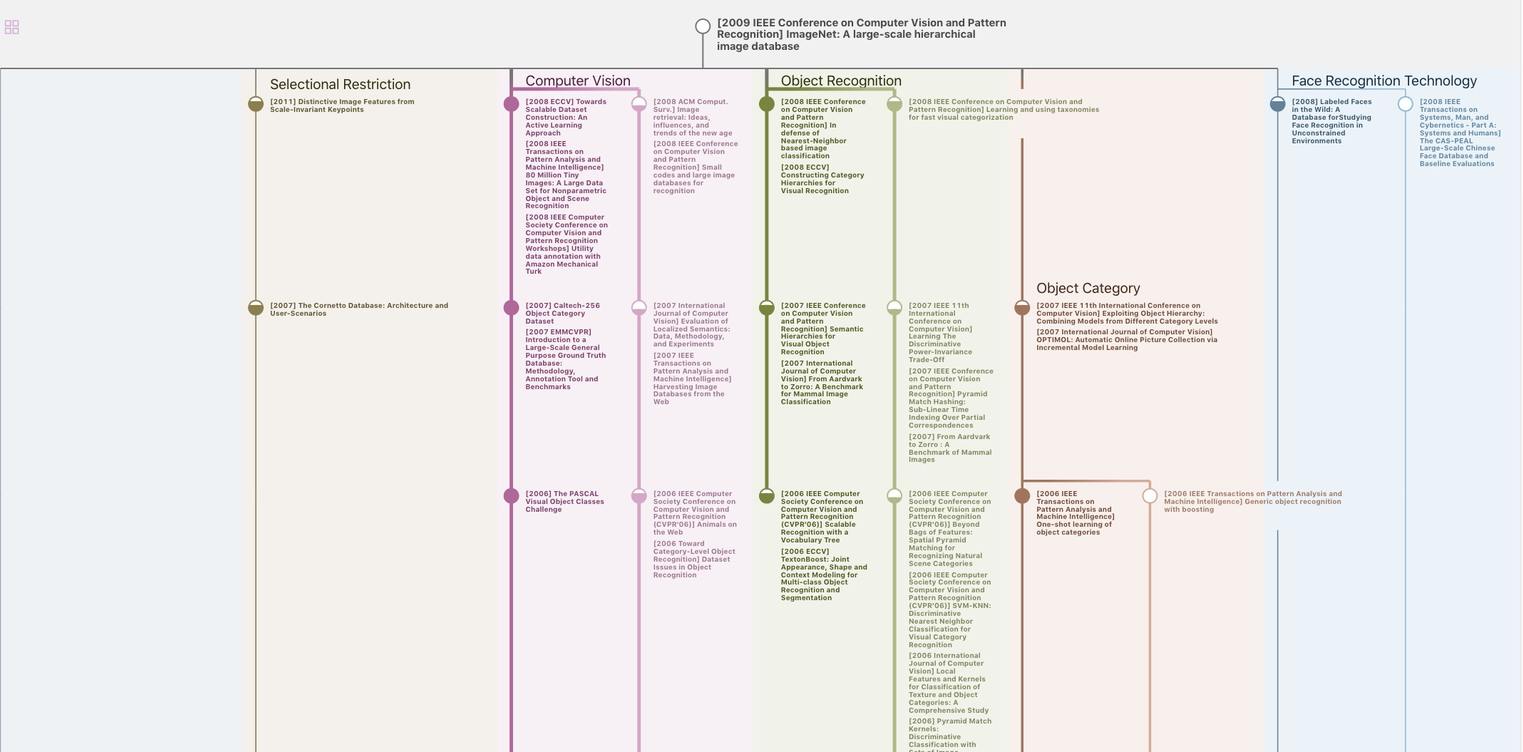
Generate MRT to find the research sequence of this paper
Chat Paper
Summary is being generated by the instructions you defined