RP-SG: Relation Prediction in 3D Scene Graphs for Unobserved Objects Localization
IEEE ROBOTICS AND AUTOMATION LETTERS(2024)
摘要
The ability to search for objects is a fundamental prerequisite for mobile robots when addressing a wide range of automation tasks. However, how to effectively estimate the positions of unobserved objects in a continuously changing environment remains an open challenge. Previous works have utilized probabilistic models to estimate the co-occurrence property between the target object and the observed landmark objects in a scene. However, few approaches can predict the precise spatial relations between objects based on a specific scene configuration. In this letter, we propose a novel unobserved object localization framework that achieves context-specific relation prediction based on the particular configuration of a scene. First, we leverage a 3D scene graph as a compact representation of the environment and propose a relation prediction model based on graph neural networks. This model can effectively interpret the information provided by the 3D scene graph and make accurate relation predictions. Second, to address the challenge of a high number of non-existent links between objects in the scene graph, we introduce a novel loss function that can better address imbalanced training data. Additionally, we propose an evaluation framework to comprehensively assess whether the relation prediction model benefits object search tasks. Comprehensive evaluation results obtained on public datasets and real-world scenes reveal the superiority of our method over competing approaches.
更多查看译文
关键词
Three-dimensional displays,Robots,Search problems,Task analysis,Location awareness,Semantics,Predictive models,Localization,semantic scene understanding,relation prediction,3D scene graph
AI 理解论文
溯源树
样例
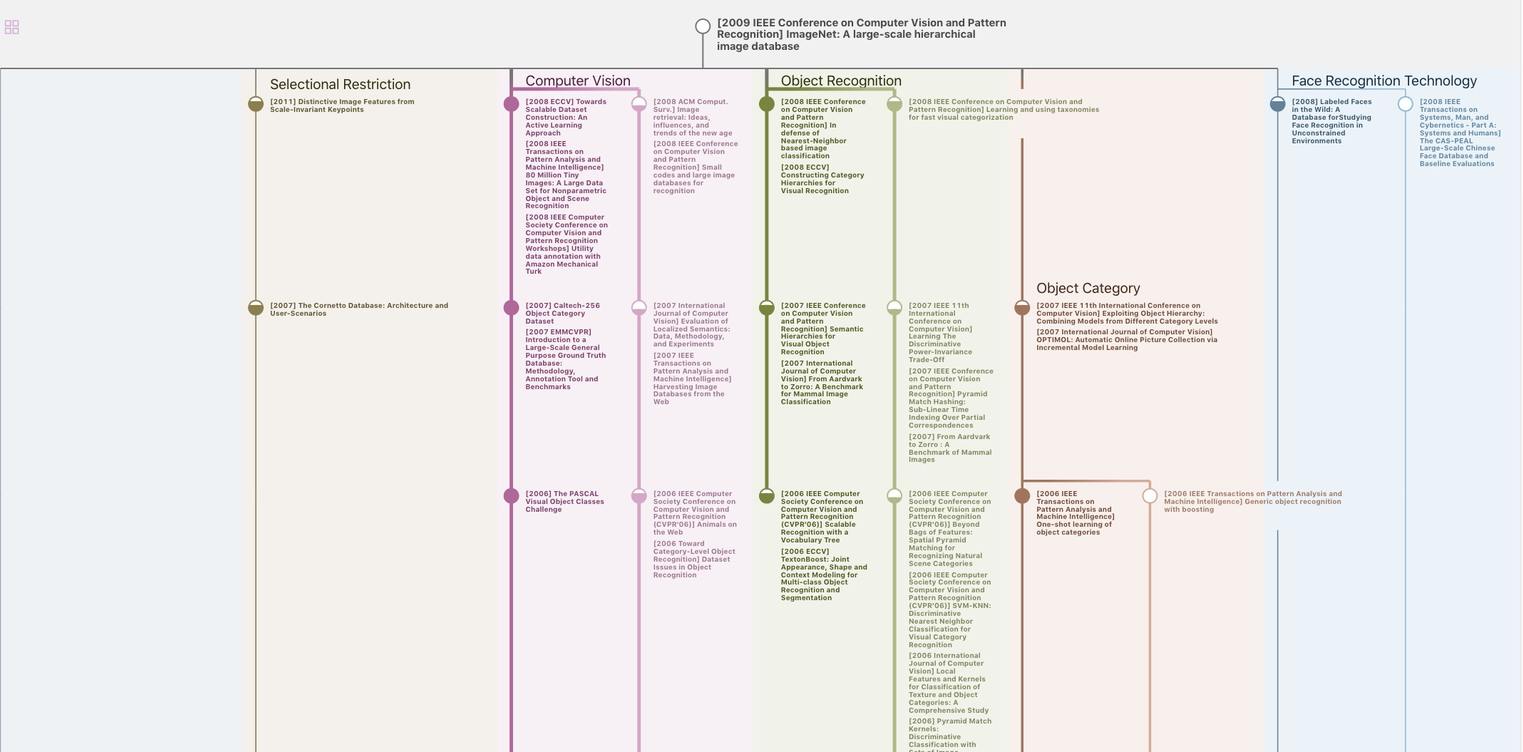
生成溯源树,研究论文发展脉络
Chat Paper
正在生成论文摘要