Factors Influencing Unfractionated Heparin Pharmacokinetics and Pharmacodynamics During a Cardiopulmonary Bypass
Clinical Pharmacokinetics(2024)
摘要
Background Unfractionated heparin (UFH) is commonly used during cardiac surgery with a cardiopulmonary bypass to prevent blood clotting. However, empirical administration of UFH leads to variable responses. Pharmacokinetic and pharmacodynamic modeling can be used to optimize UFH dosing and perform real-time individualization. In previous studies, many factors that could influence UFH pharmacokinetics/pharmacodynamics had not been taken into account such as hemodilution or the type of UFH. Few covariates were identified probably owing to a lack of statistical power. This study aims to address these limitations through a meta-analysis of individual data from two studies. Methods An individual patient data meta-analysis was conducted using data from two single-center prospective observational studies, where different UFH types were used for anticoagulation. A pharmacodynamic/pharmacodynamic model of UFH was developed using a non-linear mixed-effects approach. Time-varying covariates such as hemodilution and fluid infusions during a cardiopulmonary bypass were considered. Results Activities of UFH’s anti-activated factor/anti-thrombin were best described by a two-compartment model. Unfractionated heparin clearance was influenced by body weight and the specific UFH type. Volume of distribution was influenced by body weight and pre-operative fibrinogen levels. Pharmacodynamic data followed a log-linear model, accounting for the effect of hemodilution and the pre-operative fibrinogen level. Equations were derived from the model to personalize UFH dosing based on the targeted activated clotting time level and patient covariates. Conclusions The population model effectively characterized UFH’s pharmacokinetics/pharmacodynamics in cardiopulmonary bypass patients. This meta-analysis incorporated new covariates related to UFH’s pharmacokinetics/pharmacodynamics, enabling personalized dosing regimens. The proposed model holds potential for individualization using a Bayesian estimation.
更多查看译文
AI 理解论文
溯源树
样例
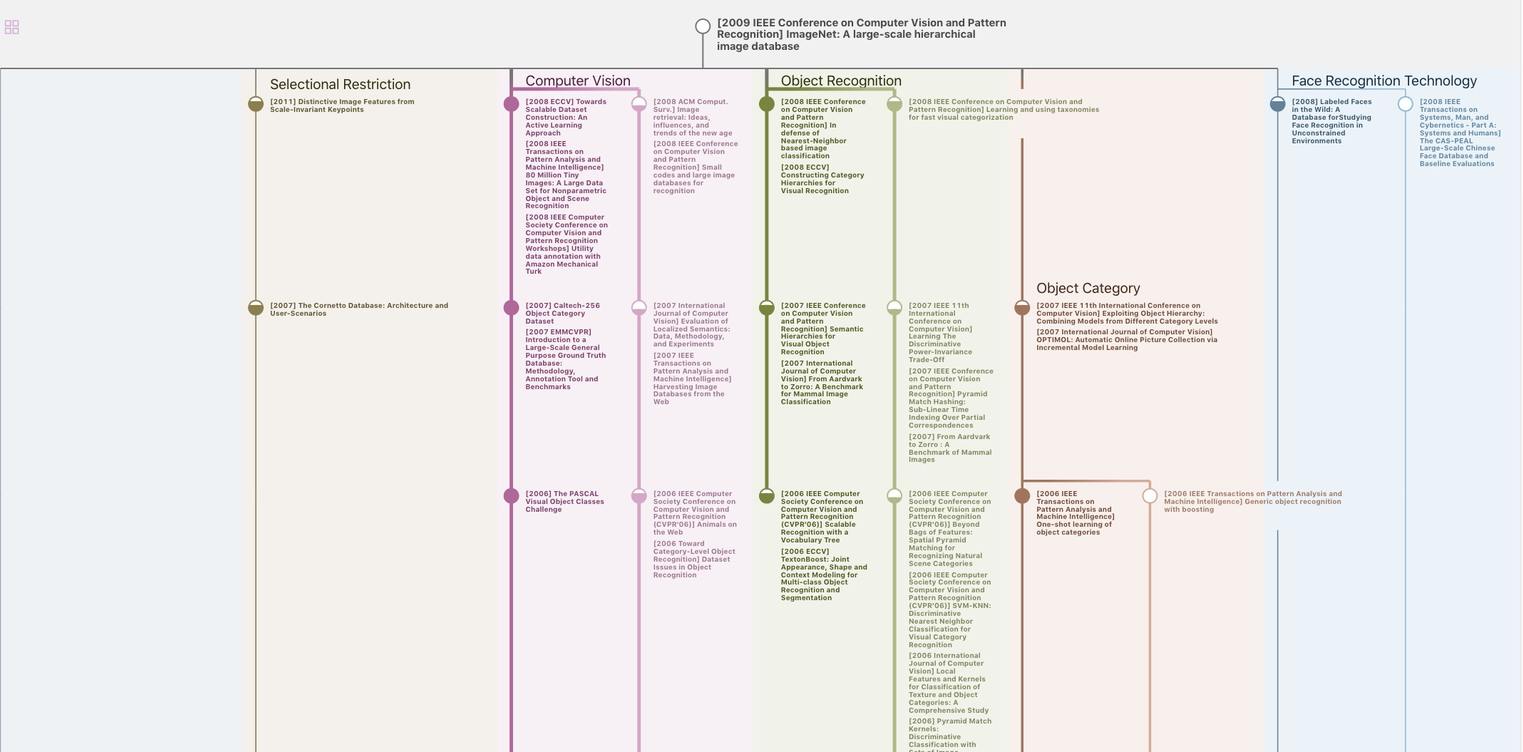
生成溯源树,研究论文发展脉络
Chat Paper
正在生成论文摘要