SALW-Net: a lightweight convolutional neural network based on self-adjusting loss function for spine MR image segmentation
Medical & Biological Engineering & Computing(2024)
摘要
Segmentation of intervertebral discs and vertebrae from spine magnetic resonance (MR) images is essential to aid diagnosis algorithms for lumbar disc herniation. Convolutional neural networks (CNN) are effective methods, but often require high computational costs. Designing a lightweight CNN is more suitable for medical sites lacking high-computing power devices, yet due to the unbalanced pixel distribution in spine MR images, the segmentation is often sub-optimal. To address this issue, a lightweight spine segmentation CNN based on a self-adjusting loss function, which is named SALW-Net, is proposed in this study. For SALW-Net, the self-adjusting loss function could dynamically adjust the loss weights of the two branches according to the differences in segmentation results and labels during the training; thus, the ability for learning unbalanced pixels is enhanced. Two separate datasets are used to evaluate the proposed SALW-Net. Specifically, the proposed SALW-Net has fewer parameter numbers than U-net (only 2%) but achieves higher evaluation scores than that of U-net (the average DSC score of SALW-Net is 0.8781, and that of U-net is 0.8482). In addition, the practicality validation for SALW-Net is also proceeding, including deploying the model on a lightweight device and producing an aid diagnosis algorithm based on segmentation results. This means our SALW-Net has clinical application potential for assisted diagnosis in low computational power scenarios. Graphical Abstract
更多查看译文
关键词
Self-adjusting loss function,Lightweight convolutional neural network,Spine MR images segmentation,Lumbar disc aid diagnosis
AI 理解论文
溯源树
样例
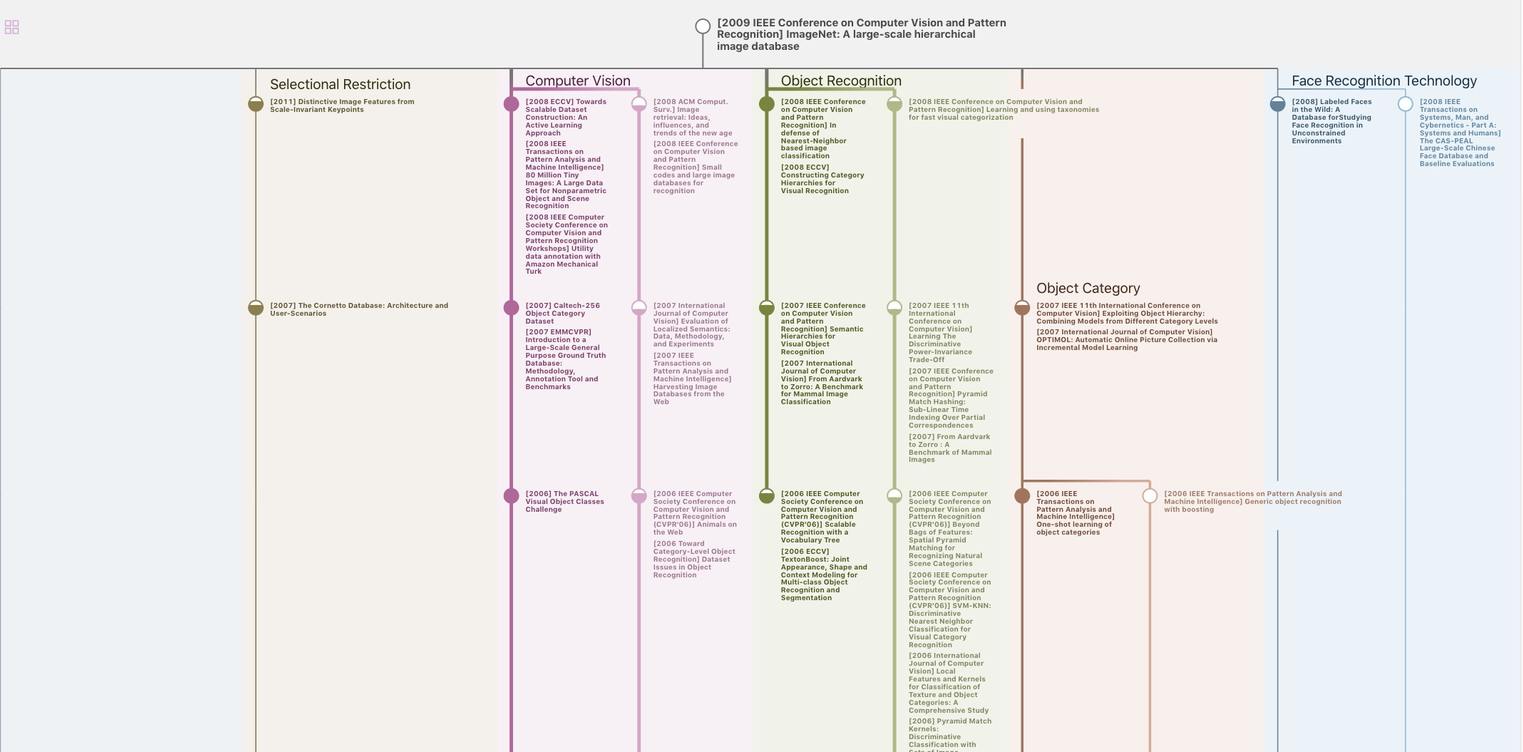
生成溯源树,研究论文发展脉络
Chat Paper
正在生成论文摘要