Natural Language Processing to Identify Racial and Ethnic Disparities in Aortic Stenosis
medrxiv(2023)
摘要
IMPORTANCE This study uses artificial intelligence (AI) technologies to augment quality measurement and improvement in the setting of aortic stenosis (AS). We characterise racial and ethnic disparities in the diagnosis, management, and outcome of AS within a universal healthcare system.
OBJECTIVE To use natural language processing (NLP) AI methods applied to the electronic health records (EHR) to identify racial and ethnic disparities in AS while correcting for the effects of socioeconomic deprivation.
DESIGN Retrospective cohort study.
SETTING King’s College Hospital NHS Foundation Trust, a multi-site tertiary care hospital in London, UK
PARTICIPANTS Adult patients with a diagnosis of AS between 2010-2020.
MAIN OUTCOMES AND MEASURES Key outcomes were all-cause mortality, frequency of AS intervention (TAVI or surgical aortic valve replacement [AVR]) and the time from diagnosis of severe AS to intervention. All analyses were adjusted for age, sex and socioeconomic deprivation.
RESULTS 5859 patients with AS were identified, with self-reported race and ethnicity labels as 4.5% Asian, 7.5% Black, and 88.0% White. For those with severe AS, TAVI was performed in 19.6% of Asian patients, 17.6% of Black patients and 24.9% of White patients; AVR was performed in 39.2% of Asian patients, 27.9% of Black patients and 32.8% of White patients. The mean time from severe AS diagnosis to TAVI was 0.69 years for Asian patients, 1.03 years for Black patients and 0.62 years for White patients (P=n.s.). The mean time to AVR was longer for Black patients (1.35 years) compared to Asian (0.49 years) and White patients (0.41 years, P<0.001). Survival in the overall cohort did not associate with ethnicity. However, in patients with severe AS, Black ethnicity was independently associated with increased mortality (hazard ratio=1.42, 95% CI=1.05-1.92, P=0.02).
CONCLUSIONS AND RELEVANCE In patients with severe AS, Black patients experience lower rates of TAVI, longer time from diagnosis to AVR and higher rates of mortality, despite correction for socioeconomic deprivation. These data exhibit how AI technologies may be leveraged to shed light on health inequities, here showing that racial and ethnic disparities in AS persist in a universal healthcare system, and should stimulate strategies to address inequity.
Question Do racial and ethnic disparities in the diagnosis, management, and outcome of aortic stenosis (AS) exist within a universal healthcare system?
Finding In this retrospective cohort study using natural language processing enabled analysis of electronic healthcare record data of 5859 patients with aortic stenosis, we identified that in severe AS, Black patients experience lower rates of transcatheter aortic valve implantation (TAVI), longer times from diagnosis to surgical aortic valve replacement (AVR) and higher rates of mortality.
Meaning Natural language processing may be used to identify health inequities. Here, we find racial and ethnic disparities in AS exist even in a universal healthcare setting.
### Competing Interest Statement
AMS serves as an advisor to Forcefield Therapeutics and CYTE - Global Network for Clinical Research.
### Funding Statement
This work was supported by grants from the British Heart Foundation (CH/1999001/11735, RG/20/3/34823 and RE/18/2/34213 to AMS; CC/22/250022 to RJDB, AMS, JT and KOG) and King's College Hospital Charity (D3003/122022/Shah/1188 to AMS). This work was funded by the British Heart Foundation Adrian Beecroft Cardiovascular Catalyst award CC/22/250022. With thanks to King's College Hospital Charity for charitable Grant that has made this research possible. Dr O'Gallagher is funded by the British Heart Foundation Centre of Research Excellence, King's College London and by a Medical Research Council Clinician Scientist Fellowship (MR/Y001311/1).
### Author Declarations
I confirm all relevant ethical guidelines have been followed, and any necessary IRB and/or ethics committee approvals have been obtained.
Yes
The details of the IRB/oversight body that provided approval or exemption for the research described are given below:
This project operated under London South-East Research Ethics Committee approval (18/LO/2048) granted to the King's Electronic Records Research Interface (KERRI). This study complies with the Declaration of Helsinki.
I confirm that all necessary patient/participant consent has been obtained and the appropriate institutional forms have been archived, and that any patient/participant/sample identifiers included were not known to anyone (e.g., hospital staff, patients or participants themselves) outside the research group so cannot be used to identify individuals.
Yes
I understand that all clinical trials and any other prospective interventional studies must be registered with an ICMJE-approved registry, such as ClinicalTrials.gov. I confirm that any such study reported in the manuscript has been registered and the trial registration ID is provided (note: if posting a prospective study registered retrospectively, please provide a statement in the trial ID field explaining why the study was not registered in advance).
Yes
I have followed all appropriate research reporting guidelines, such as any relevant EQUATOR Network research reporting checklist(s) and other pertinent material, if applicable.
Yes
The datasets analysed during the current study are not publicly available due to hospital information governance regulations but are available from the corresponding author on reasonable request.
* AS
: Aortic Stenosis
AVR
: Surgical aortic valve replacement
IMD
: Index of multiple deprivations
LVEF
: Left ventricular ejection fraction
NLP
: Natural language processing
TAVI
: Transcatheter aortic valve implantation
更多查看译文
AI 理解论文
溯源树
样例
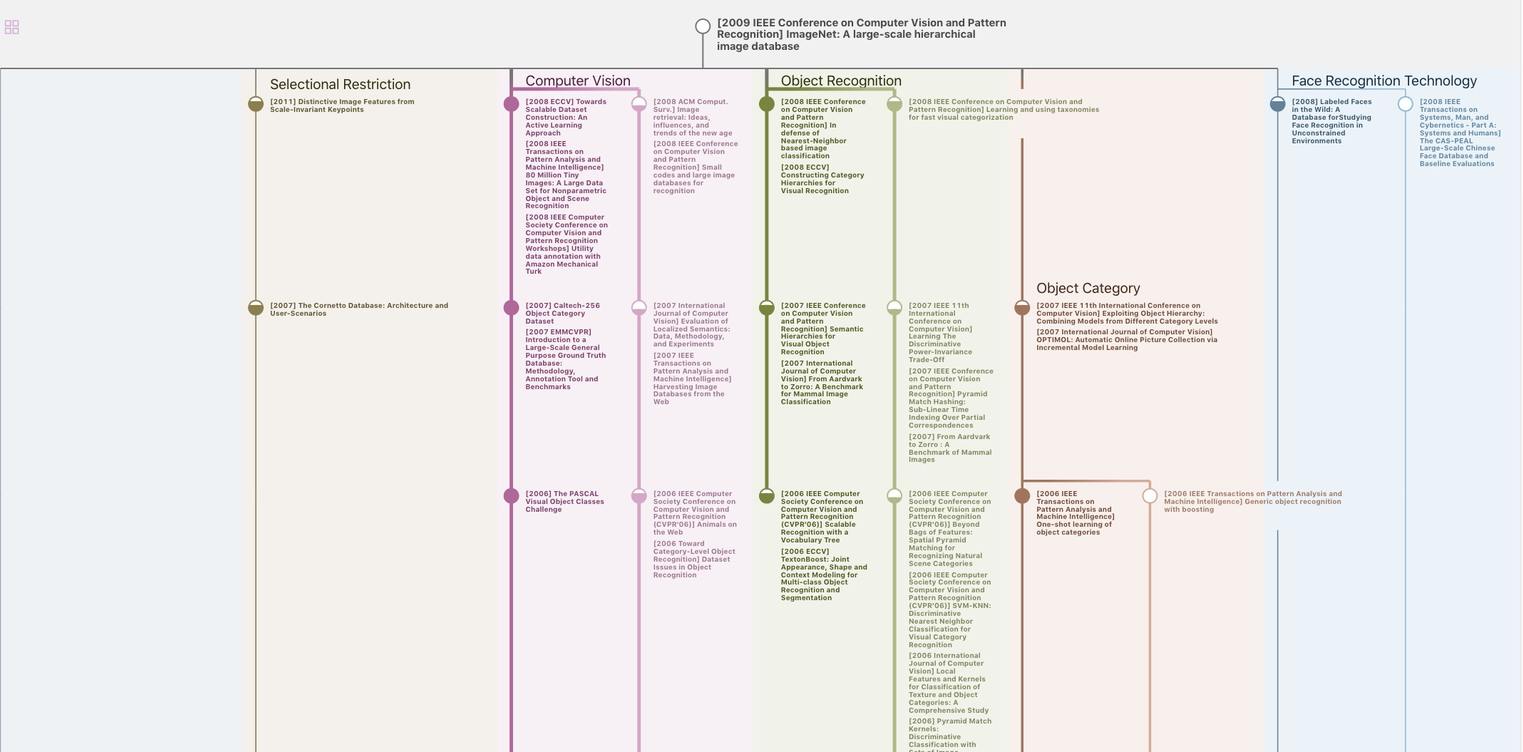
生成溯源树,研究论文发展脉络
Chat Paper
正在生成论文摘要