One-Step Late Fusion Multi-view Clustering with Compressed Subspace
CoRR(2024)
摘要
Late fusion multi-view clustering (LFMVC) has become a rapidly growing class
of methods in the multi-view clustering (MVC) field, owing to its excellent
computational speed and clustering performance. One bottleneck faced by
existing late fusion methods is that they are usually aligned to the average
kernel function, which makes the clustering performance highly dependent on the
quality of datasets. Another problem is that they require subsequent k-means
clustering after obtaining the consensus partition matrix to get the final
discrete labels, and the resulting separation of the label learning and cluster
structure optimization processes limits the integrity of these models. To
address the above issues, we propose an integrated framework named One-Step
Late Fusion Multi-view Clustering with Compressed Subspace (OS-LFMVC-CS).
Specifically, we use the consensus subspace to align the partition matrix while
optimizing the partition fusion, and utilize the fused partition matrix to
guide the learning of discrete labels. A six-step iterative optimization
approach with verified convergence is proposed. Sufficient experiments on
multiple datasets validate the effectiveness and efficiency of our proposed
method.
更多查看译文
关键词
Multi-view Clustering,Unsupervised learning and clustering,Late Fusion,One Step
AI 理解论文
溯源树
样例
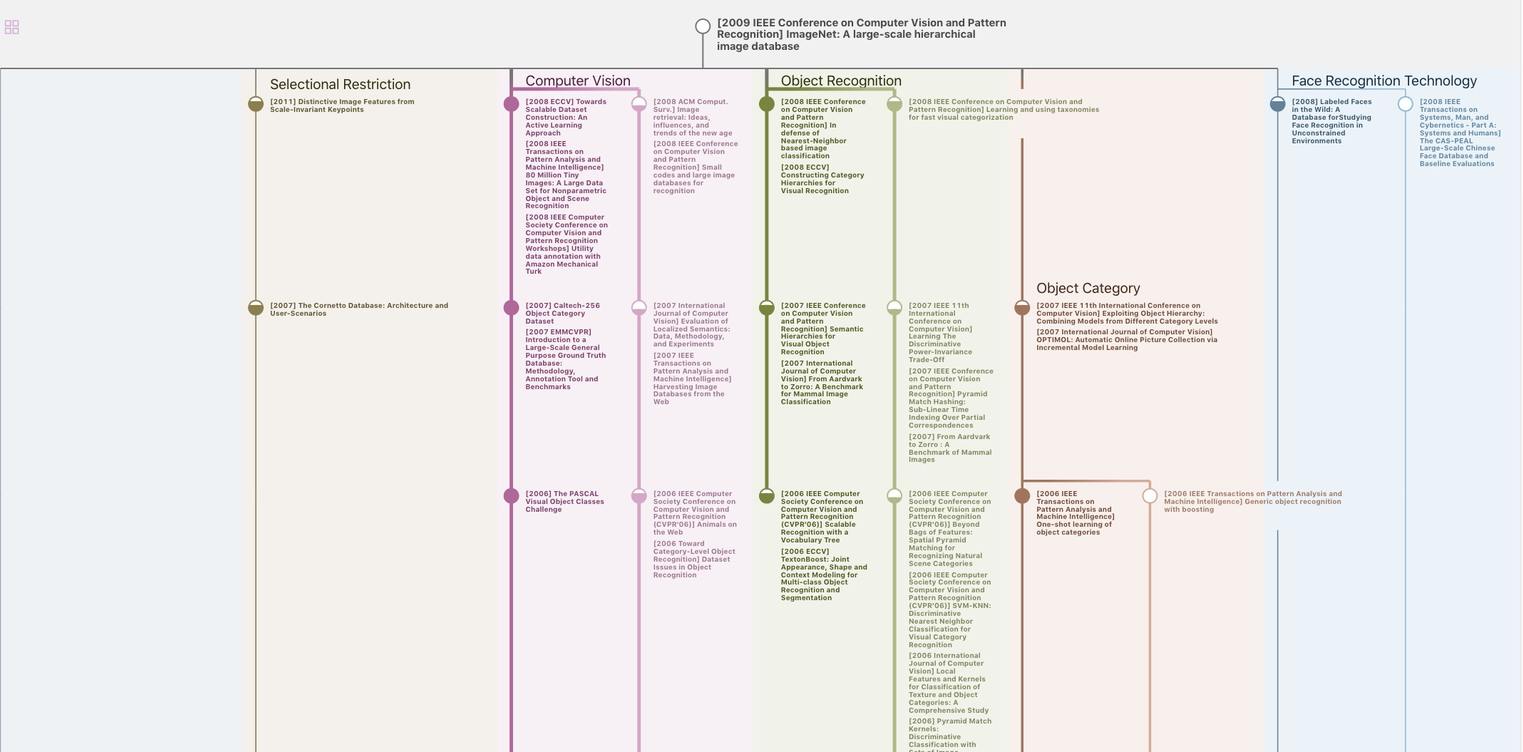
生成溯源树,研究论文发展脉络
Chat Paper
正在生成论文摘要