Prompted representation joint contrastive learning for aspect-based sentiment analysis
KNOWLEDGE-BASED SYSTEMS(2024)
摘要
As a fine-grained and challenging subtask in the natural language processing (NLP) community, aspectbased sentiment analysis (ABSA) aims to predict the sentiment polarity towards a given aspect term. In previous ABSA research, most works utilized the pre-trained language model (PLM) as the backbone of their proposed methods, without any specific task-related instructions. Besides, some works focused on learning the dependency information or the external knowledge-enhanced dependency information separately, which lacked the exploitation of the mutual interaction between the normal dependency and knowledge-enhanced dependency. Therefore, we propose a novel ABSA method namely prompted representation joint contrastive learning enhanced graph convolutional networks (PRCL-GCN) to strengthen the robustness of the ABSA model. Specifically, to achieve the task-oriented contextual representation, we design the task-specific prompt template to guide the fine-tuning process of PLM in the ABSA task. And a biaffine attention mechanism is employed to further extract the essential sentiment feature from the prompted representation. Moreover, we introduce the syntax dependency graph as prior knowledge, and construct an affective syntactic dependency graph by injecting the affective knowledge from SenticNet into the graph. Then, we utilize the multi-layer GCNs to process the above two syntactic graphs independently, which aims to learn multi-granularity syntactic features. Subsequently, a novel designed attention variant is leveraged to integrate these syntax features with the guided contextual representation, separately. Eventually, through designing a Kullback-Leibler divergencebased contrastive learning to encourage the model's learning, we improve the model's accuracy in modeling contextual representation by integrating the designed dual-ways information. Extensive experiments are conducted on five benchmark datasets, and the outstanding experiment results validate the effectiveness of our proposed model.
更多查看译文
关键词
Aspect-based sentiment analysis,Graph convolutional networks,Biaffine attention,Sentiment knowledge,Contrastive learning
AI 理解论文
溯源树
样例
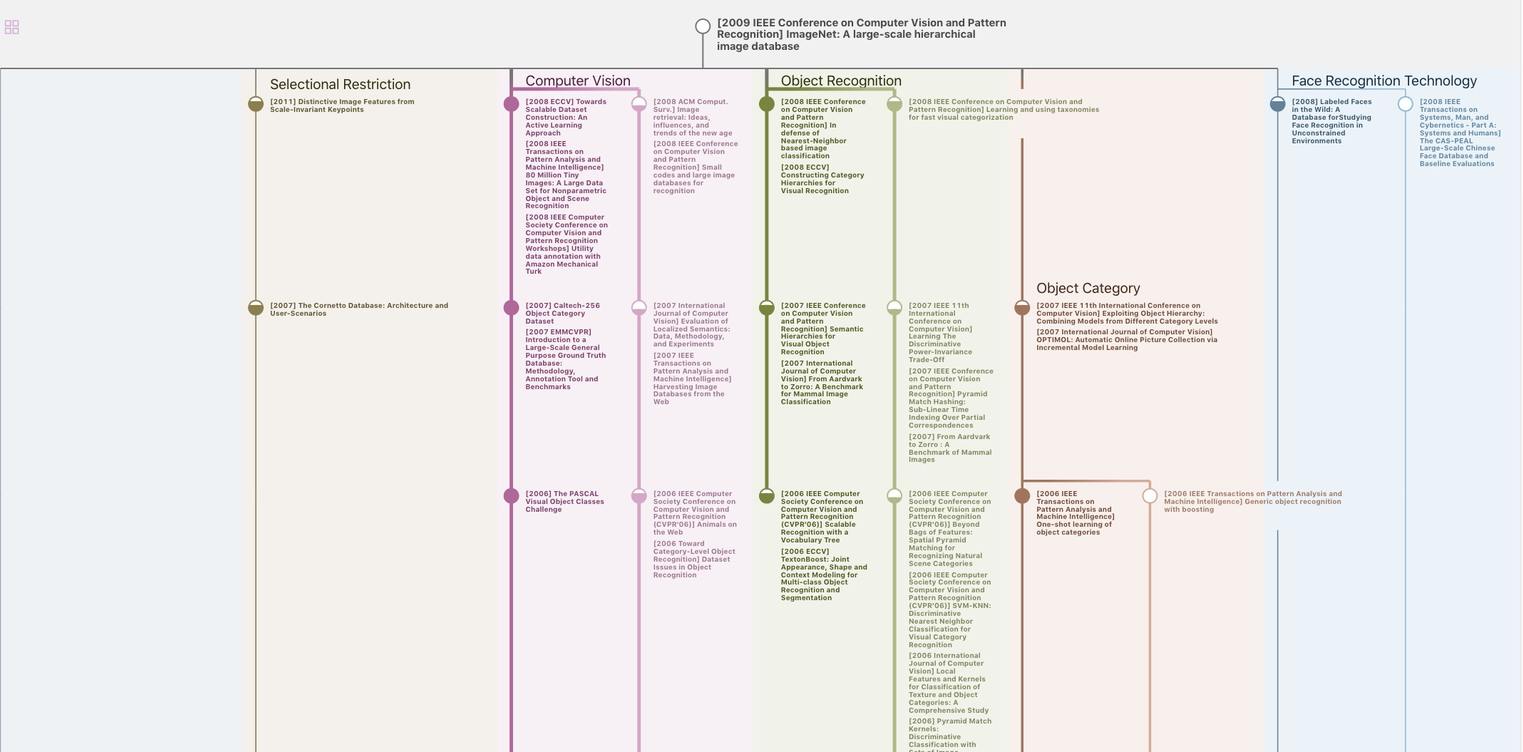
生成溯源树,研究论文发展脉络
Chat Paper
正在生成论文摘要