HubHSP graph: Capturing local geometrical and statistical data properties via spanning graphs
INFORMATION SYSTEMS(2024)
摘要
The computation of a continuous generative model to describe a finite sample of an infinite metric space can prove challenging and lead to erroneous hypothesis, particularly in high-dimensional spaces. In this paper, we follow a different route and define the Hubness Half Space Partitioning graph (HubHSP graph). By constructing this spanning graph over the dataset, we can capture both the geometrical and statistical properties of the data without resorting to any continuity assumption. Leveraging the classical graph-theoretic apparatus, the HubHSP graph facilitates critical operations, including the creation of a representative sample of the original dataset, without relying on density estimation. This representative subsample is essential for a range of operations, including indexing, visualization, and machine learning tasks such as clustering or inductive learning. With the HubHSP graph, we can bypass the limitations of traditional methods and obtain a holistic understanding of our dataset's properties, enabling us to unlock its full potential.
更多查看译文
关键词
Data analysis,Data modeling,Graph-based representation,Graph centrality,Half-space partitioning
AI 理解论文
溯源树
样例
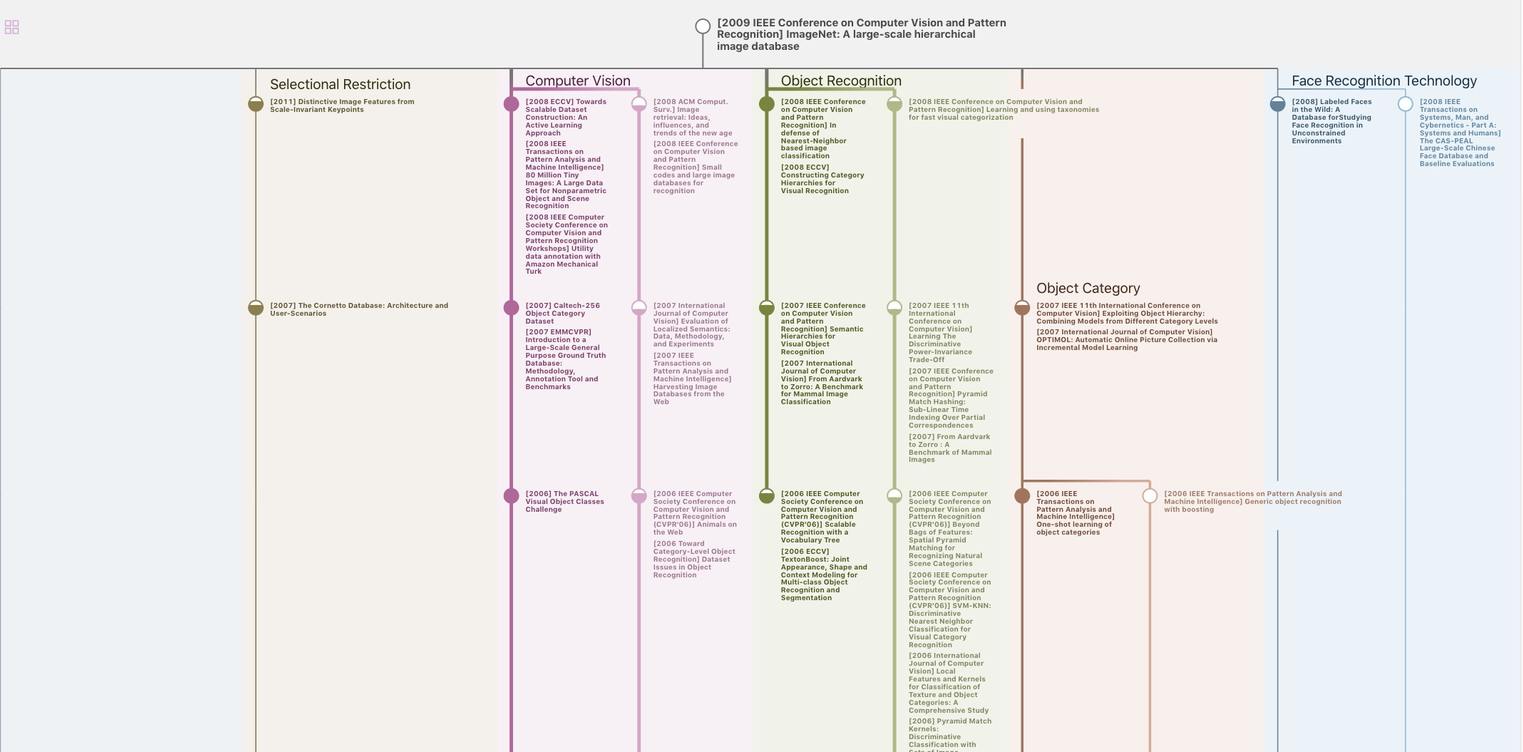
生成溯源树,研究论文发展脉络
Chat Paper
正在生成论文摘要